A consistent and flexible framework for deep matrix factorizations
Pattern Recognition(2023)
摘要
Deep matrix factorizations (deep MFs) are recent unsupervised data mining techniques inspired by con-strained low-rank approximations. They aim to extract complex hierarchies of features within high -dimensional datasets. Most of the loss functions proposed in the literature to evaluate the quality of deep MF models and the underlying optimization frameworks are not consistent because different losses are used at different layers. In this paper, we introduce two meaningful loss functions for deep MF and present a generic framework to solve the corresponding optimization problems. We illustrate the effec-tiveness of this approach through the integration of various constraints and regularizations, such as spar-sity, nonnegativity and minimum-volume. The models are successfully applied on both synthetic and real data, namely for hyperspectral unmixing and extraction of facial features.(c) 2022 Elsevier Ltd. All rights reserved.
更多查看译文
关键词
Deep matrix factorization,Loss functions,Constrained optimization,First -order methods,Hyperspectral unmixing
AI 理解论文
溯源树
样例
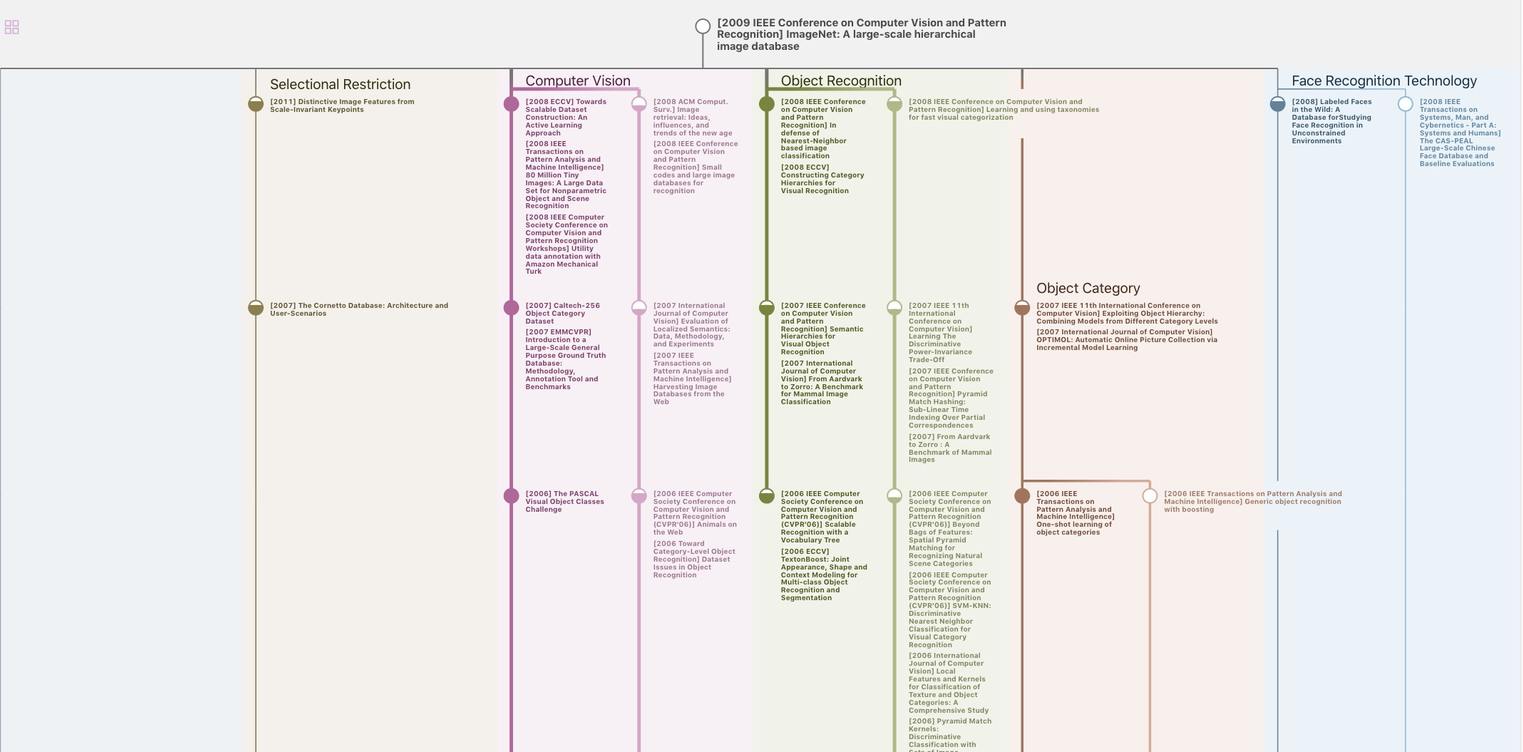
生成溯源树,研究论文发展脉络
Chat Paper
正在生成论文摘要