Performance Prediction Under Dataset Shift.
ICPR(2022)
摘要
ML models deployed in production often have to face unknown domain changes, fundamentally different from their training settings. Performance prediction models carry out the crucial task of measuring the impact of these changes on model performance. We study the generalization capabilities of various performance prediction models to new domains by learning on generated synthetic perturbations. Empirical validation on a benchmark of ten tabular datasets shows that models based upon state-of-the-art shift detection metrics are not expressive enough to generalize to unseen domains, while Error Predictors bring a consistent improvement in performance prediction under shift. We additionally propose a natural and effortless uncertainty estimation of the predicted accuracy that ensures reliable use of performance predictors. Our implementation is available at https: //github.com/dataiku-research/performance_prediction_under_shift.
更多查看译文
关键词
dataset shift,error predictors,generalization capabilities,ML models,performance prediction models,performance predictors,predicted accuracy,shift detection metrics,tabular datasets,training settings,unknown domain changes,unseen domains
AI 理解论文
溯源树
样例
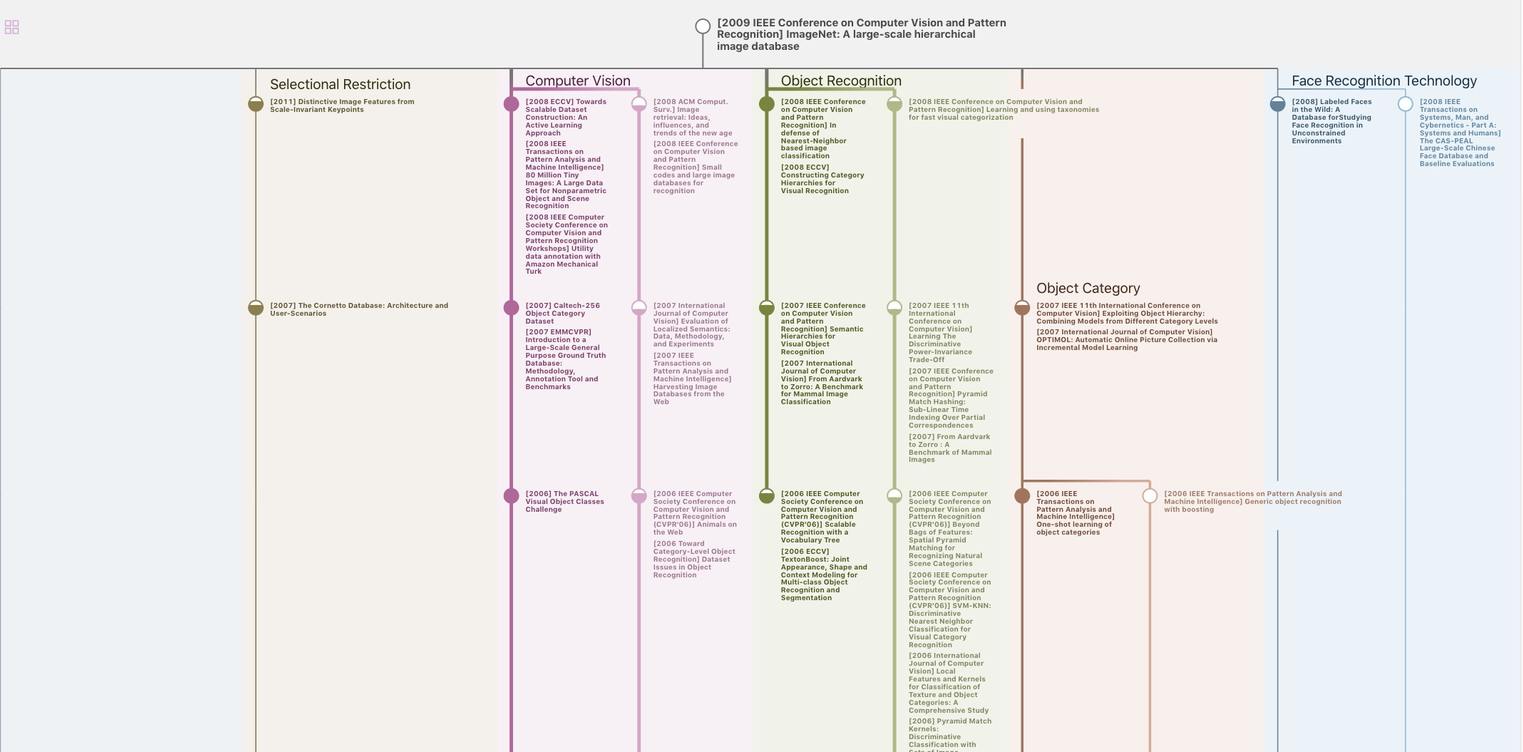
生成溯源树,研究论文发展脉络
Chat Paper
正在生成论文摘要