Abstract 251: Natural Language Processing Of Clinical Documentation To Assess Racial Differences In The Clinical Profile Of Patients Hospitalized With Heart Failure
Circulation-cardiovascular Quality and Outcomes(2022)
摘要
Background: Self-reported racial classification is often used as a proxy for phenotypic differences among clinical populations. We demonstrate an approach to identify differences between Black and White individuals using clinical notes among patients hospitalized with heart failure (HF). Methods: We identified HF hospitalizations for patients at Yale and developed a word frequency embedding (TF-IDF)-based natural language processing (NLP) model on clinical notes to classify “tokens” or words from notes of Black and White individuals based on their frequency. We manually classified these tokens into clinical and non-clinical entities. We evaluated the frequency of clinical tokens successfully identified in ICD diagnosis codes and qualitatively assessed non-clinical tokens for stigmatizing language across Black and White patients. Results: There were 8798 patients (mean age 81±13y, 50% female) with a total of 14,655 unique patient encounters. Of these, 4293 were for Black patients. The NLP-based model determined Black vs White race of patients based only on notes with an AUROC of 0.92 after removing all explicit mentions of race. Of the top 80 tokens that identified Black vs White patients from notes, 53 (66%) were identified as clinical entities. The clinical tokens were underrepresented in structured ICD diagnosis for both Black and White, with a median 88% (IQR=27%, Q1=66%, Q3=94%) of Black-identifying tokens defining clinical conditions and 72% (IQR=45%, Q1=43%, Q3=89%) for White-identifying tokens. Among non-clinical tokens, there was a greater occurrence of stigmatizing words in the notes of Black patients (40% vs 32% in White). Conclusion: We demonstrate that an NLP-based approach can better define clinical differences between Black and White patients, and can be used to screen for stigmatizing language against Black and other minority groups.
更多查看译文
AI 理解论文
溯源树
样例
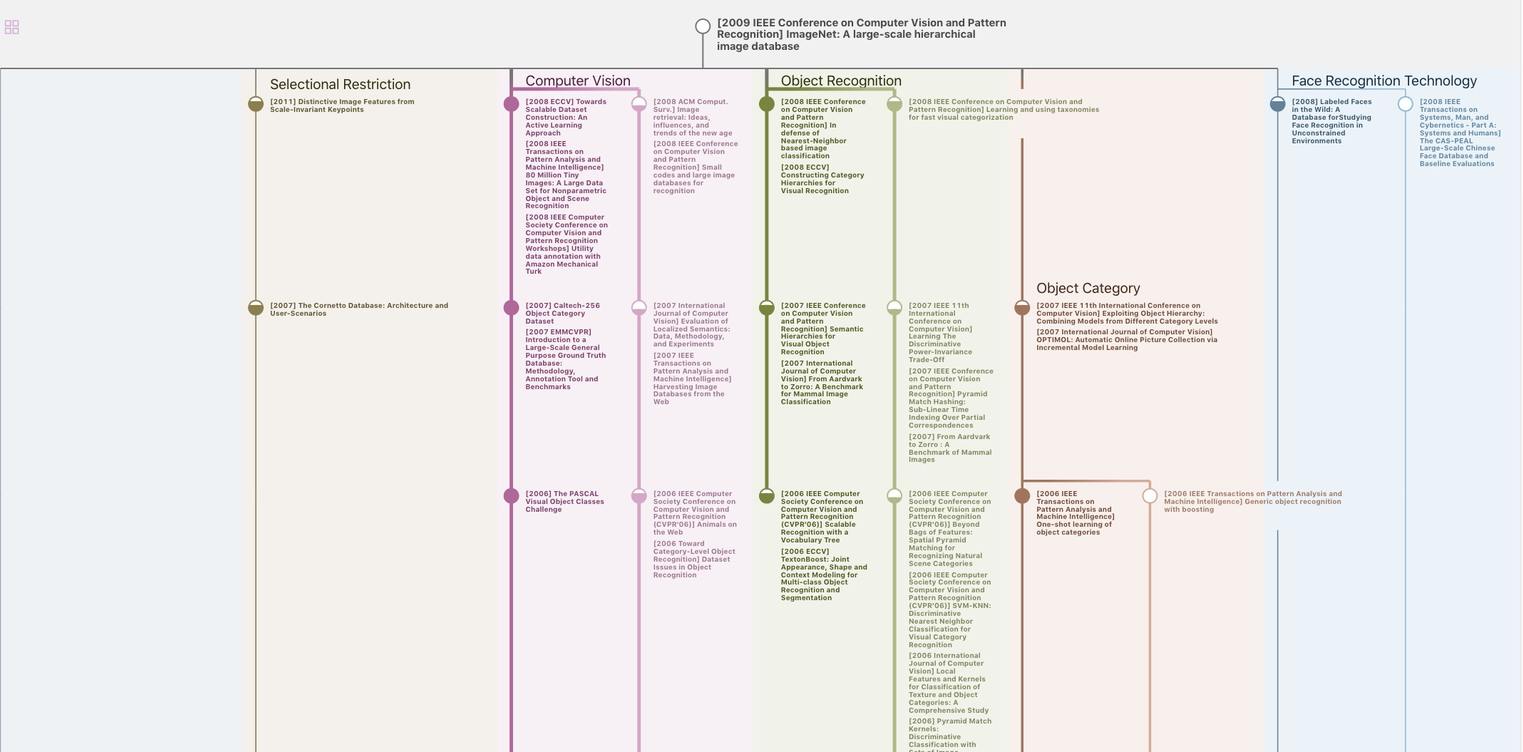
生成溯源树,研究论文发展脉络
Chat Paper
正在生成论文摘要