LEARNING FROM LAW ENFORCEMENT
Social Science Research Network(2022)
摘要
This paper studies how punishment affects future compliance behavior and isolates deterrence effects mediated by learning. Using administrative data from speed cameras that capture the full driving histories of more than a million cars over several years, we evaluate responses to punishment at the extensive (receiving a speeding ticket) and intensive margins (tickets with higher fines). Two complementary empirical strategies-a regression discontinuity design and an event studycoherently document strong responses to receiving a ticket: The speeding rate drops by a third and re-offense rates fall by 70%. Higher fines produce a small but imprecisely estimated additional effect. All responses occur immediately and are persistent over time, with no backsliding toward speeding even two years after receiving a ticket. Our evidence rejects unlearning and temporary salience effects. Instead, it supports a learning model in which agents update their priors on the expected punishment in a coarse manner.
更多查看译文
关键词
learning,law
AI 理解论文
溯源树
样例
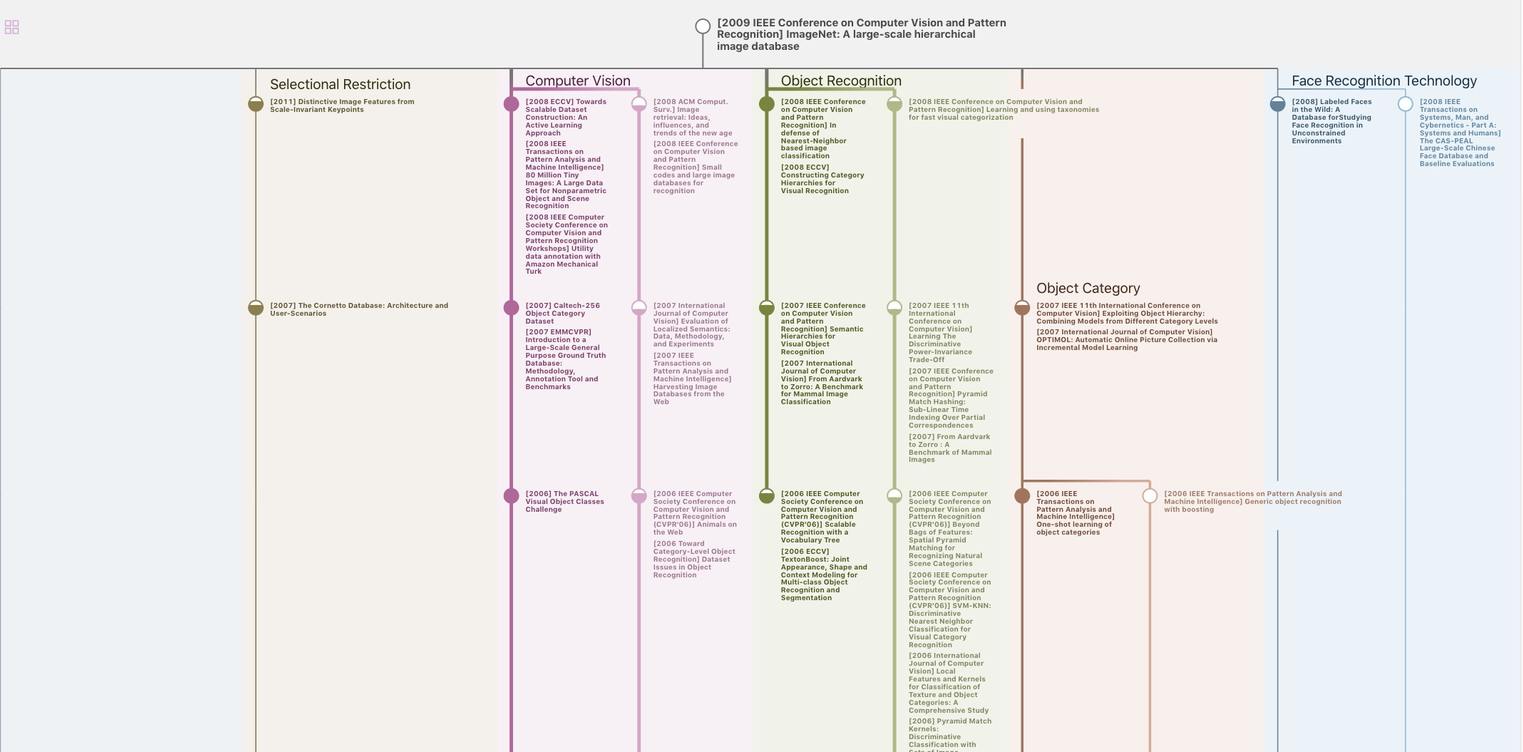
生成溯源树,研究论文发展脉络
Chat Paper
正在生成论文摘要