Abnormal traffic detection-based on memory augmented generative adversarial IIoT-assisted network
Wireless Networks(2022)
摘要
The implementation of IoT in industrial management is referred as Industrial IoT (IIoT). It is used to increase the overall operational efficiency. IIoT is considered as the backbone of the contemporary industries. For this purpose, strong security foundations have to be deployed with IIoT. This accelerates the industrial automation process by enrolling thousands of IoT devices and thus befitting the scattered connection and constrained functionalities of the IoT devices. In the Industrial Internet of Things, abnormal traffic detection is an emerging dilemma in network security. It can be ensured by monitoring the traffic flow in large-scale networks. In this connection, the deep learning paradigm has been accepted as the mainstream phenomenon in the field of abnormal traffic detection. It has achieved optimal results due to the constant improvement of computer performance with the help of Artificial Intelligence. Majority of the existing methods are based on supervised learning. As a result, due to numerous constraints, obtaining and marking abnormal traffic data samples in real life is extremely difficult. In addition, due to the diversity and complexity of the abnormal network data, the adaptability of various detection methods is low as well as difficult to judge the new abnormal traffic. Based on the above problems, this paper has proposed a semi-supervised abnormal flow detection framework in the IIoT based network scenario. The proposed model is named Memory Augment Based on Generative Adversarial Network (MeAEG-Net). It detects anomalies by training only normal flow of sample data and comparing the reconstruction errors of the underlying characteristics of input flow of generator module. To improve the generator's training, generative adversarial network has been deployed to tackle the problem of the autoencoder which is susceptible to noise. The generator uses an autoencoder and decoder structure. In addition, the memory augments module is introduced to the autoencoder's sub-network to reduce the generator module's generalization ability and raise the abnormal traffic reconstruction error. Our experimental results show that the method proposed in this paper can achieve a good effect of abnormal traffic detection in IIoT based networks under the premise of learning normal traffic data samples and improving the overall performance in all sectors of the industry.
更多查看译文
关键词
Abnormal traffic detection,Memory augmented,IIoT-assisted network
AI 理解论文
溯源树
样例
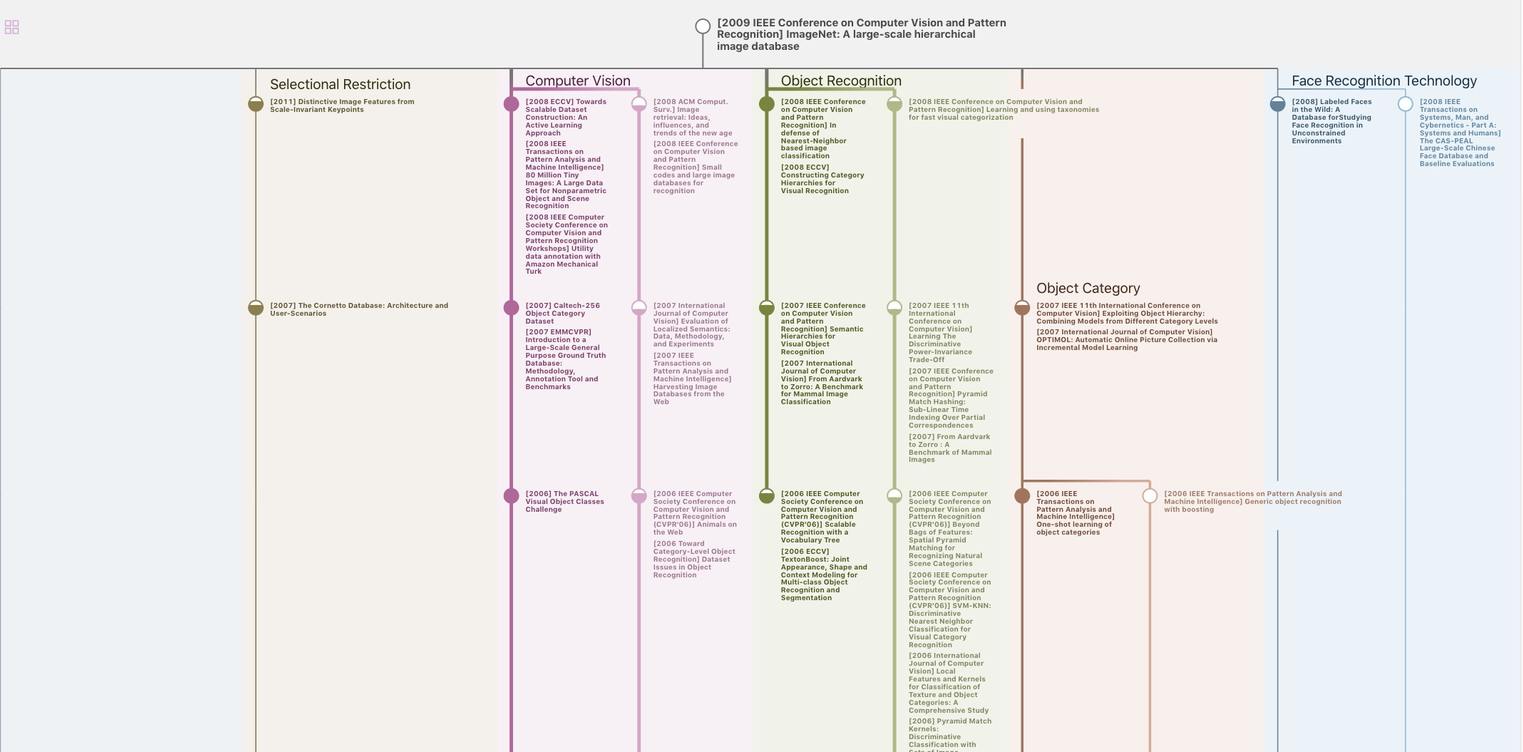
生成溯源树,研究论文发展脉络
Chat Paper
正在生成论文摘要