A Multiple-Target Simultaneous Detection Method for Immunosorbent Assay and Immunospot Assay
ANALYTICAL CHEMISTRY(2022)
摘要
Enzyme-linked immunosorbent assay (ELISA) is one of the most common methods in biological studies, and enzyme-linked immunospot (ELISpot) is a method to measure specific cell numbers by detecting protein secretion at a single-cell level. However, these two current methods can only detect one signal at one time and the sensitivity is not high enough to test low-concentration samples, which are major shortcomings in systematically analyzing the samples of interest. Herein, we demonstrated fluorescence-based oligo-linked immunosorbent assay (FOLISA) and fluorescence-based oligo-linked immunospot (FOLISPOT), which utilized DNA-barcoded antibodies to provide a highly multiplexed method with signal amplification. Signal amplification and simultaneous multiple-target detection were achieved by DNA complementary pairing and modular orthogonal DNA concatemers. By comparing FOLISA with traditional ELISA and comparing FOLISPOT with traditional ELISPOT, we found that the detection sensitivities of FOLISA and FOLISPOT are much higher than those of traditional ELISA and ELISPOT. The detection limit of ELISA is around 3 pg/mL, and the detection limit of FOLISA is below 0.06 pg/mL. FOLISPOT can detect more spots than ELISPOT and can detect targets that are undetectable for ELISPOT. Furthermore, FOLISA and FOLISPOT allowed sequential detection of multiple targets by using a single dye or multiple dyes in one round and sequential detection in multiple rounds. Thus, FOLISA and FOLISPOT enabled simultaneous detection of a large number of targets, significantly improved the detection sensitivity, and overcame the shortcomings of ELISA and ELISPOT. Overall, FOLISA and FOLISPOT presented effective and general platforms for rapid and multiplexed detection of antigens or antibodies with high sensitivity, either in laboratory tests or potentially in clinic tests.
更多查看译文
AI 理解论文
溯源树
样例
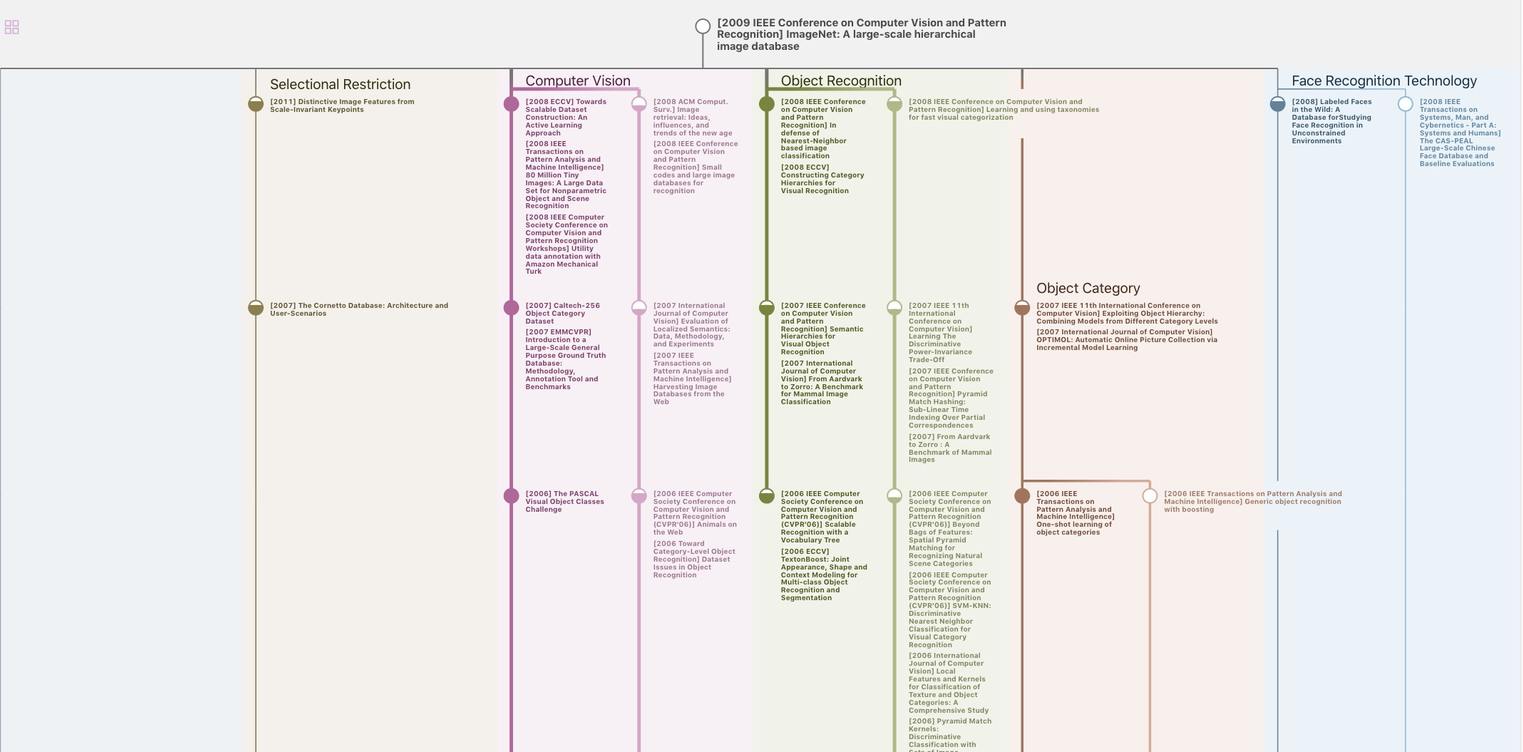
生成溯源树,研究论文发展脉络
Chat Paper
正在生成论文摘要