OUP accepted manuscript
Bioinformatics(2022)
摘要
The field of natural language processing (NLP) has recently seen a large change towards using pre-trained language models for solving almost any task. Despite showing great improvements in benchmark datasets for various tasks, these models often perform sub-optimal in non-standard domains like the clinical domain where a large gap between pre-training documents and target documents is observed. In this paper, we aim at closing this gap with domain-specific training of the language model and we investigate its effect on a diverse set of downstream tasks and settings.We introduce the pre-trained CLIN-X (Clinical XLM-R) language models and show how CLIN-X outperforms other pre-trained transformer models by a large margin for ten clinical concept extraction tasks from two languages. In addition, we demonstrate how the transformer model can be further improved with our proposed task- and language-agnostic model architecture based on ensembles over random splits and cross-sentence context. Our studies in low-resource and transfer settings reveal stable model performance despite a lack of annotated data with improvements of up to 47 F1 points when only 250 labeled sentences are available. Our results highlight the importance of specialized language models, such as CLIN-X, for concept extraction in non-standard domains, but also show that our task-agnostic model architecture is robust across the tested tasks and languages so that domain- or task-specific adaptations are not required.The CLIN-X language models and source code for fine-tuning and transferring the model are publicly available at https://github.com/boschresearch/clin_x/ and the huggingface model hub.
更多查看译文
关键词
concept extraction,language models,clinical,pre-trained,cross-task
AI 理解论文
溯源树
样例
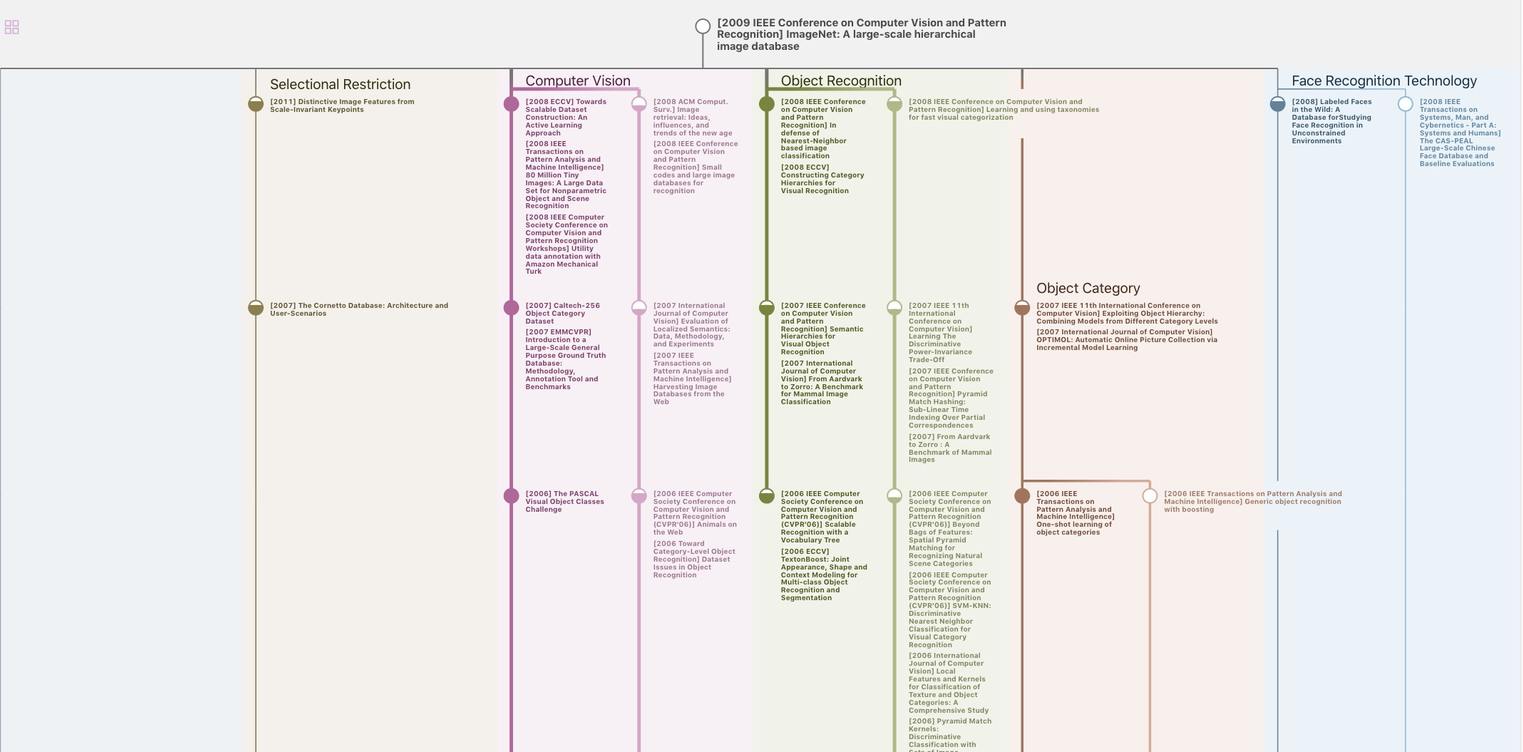
生成溯源树,研究论文发展脉络
Chat Paper
正在生成论文摘要