ω-net: Dual supervised medical image segmentation with multi-dimensional self-attention and diversely-connected multi-scale convolution
Neurocomputing(2022)
摘要
Although U-Net and its variants have achieved some great successes in medical image segmentation tasks, their segmentation performances for small objects are still unsatisfactory. Therefore, in this work, a new deep model, ω-Net, is proposed to achieve more accurate medical image segmentations. The advancements of ω-Net are mainly threefold: First, it incorporates an additional expansive path into U-Net to import an extra supervision signal and obtain a more effective and robust image segmentation by dual supervision. Then, a multi-dimensional self-attention mechanism is further developed to highlight salient features and suppress irrelevant ones consecutively in both spatial and channel dimensions. Finally, to reduce semantic disparity between the feature maps of the contracting and expansive paths, we further propose to integrate diversely-connected multi-scale convolution blocks into the skip connections, where several multi-scale convolutional operations are connected in both series and parallel. Extensive experimental results on three abdominal CT segmentation tasks show that (i) ω-Net greatly outperforms the state-of-the-art image segmentation methods in medical image segmentation tasks; (ii) the proposed three advancements are all effective and essential for ω-Net to achieve the superior performances; and (iii) the proposed multi-dimensional self-attention (resp., diversely-connected multi-scale convolution) is more effective than the state-of-the-art attention mechanisms (resp., multi-scale solutions) for medical image segmentations. The code will be released online after this paper is formally accepted.
更多查看译文
关键词
Medical image segmentation,Dual supervision,Multi-dimensional self-attention,Diversely-connected multi-scale convolution
AI 理解论文
溯源树
样例
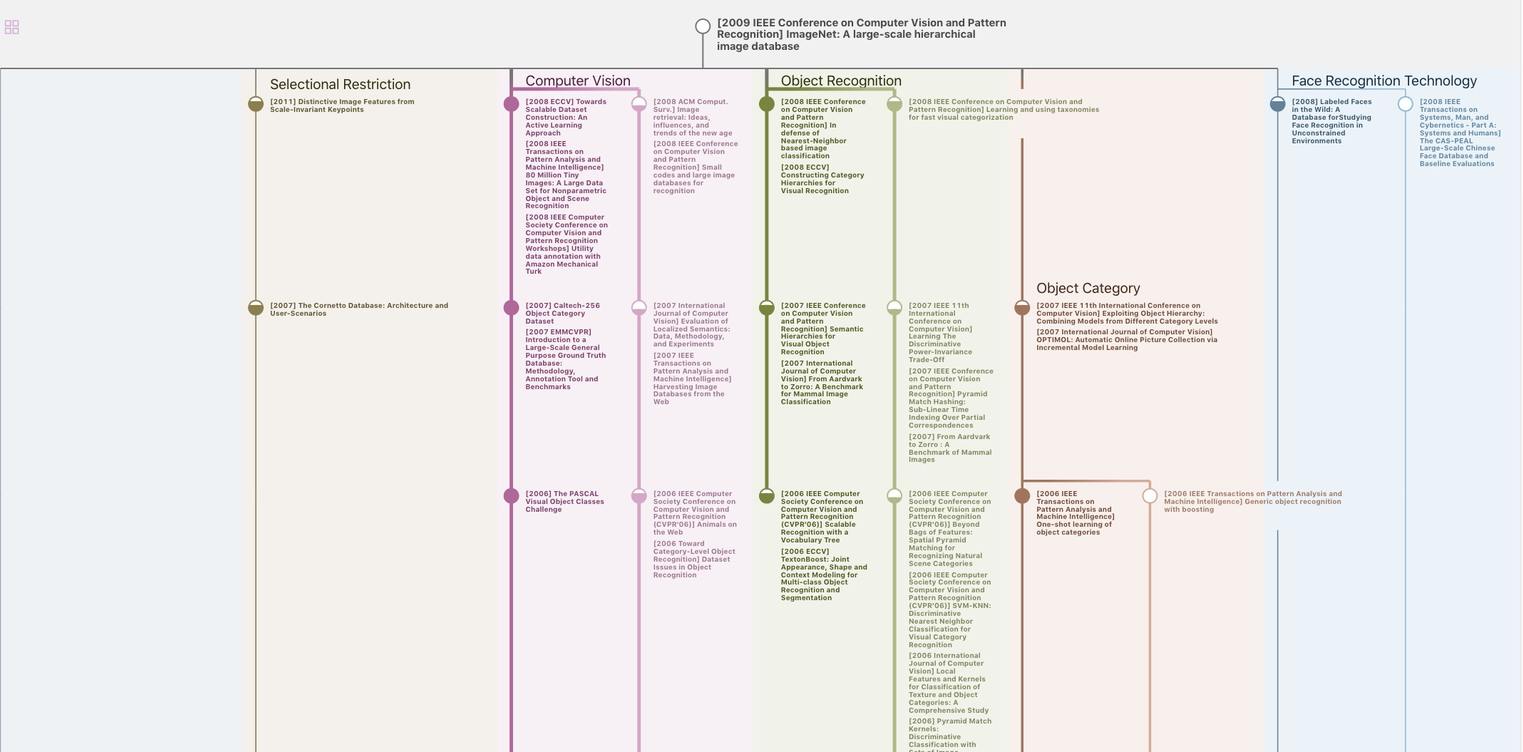
生成溯源树,研究论文发展脉络
Chat Paper
正在生成论文摘要