OMGH: Online Manifold-Guided Hashing for Flexible Cross-Modal Retrieval
IEEE TRANSACTIONS ON MULTIMEDIA(2023)
摘要
Cross-modal hashing hasrecently gained an increasing attention for its efficiency and fast retrieval speed in indexing the multimedia data across different modalities. Nevertheless, the multimedia data points often emerge in a streaming manner, and existing online methods often lack of learning capacity to handle both labeled and unlabeled data.To alleviate these concerns, this paper proposes an Online Manifold-Guided Hashing (OMGH) framework, which can incrementally learn the compact hash code of streaming data while adaptively optimizing the hash function in a streaming manner. To be specific, OMGH first exploits a matrix tri-factorization framework to learn the discriminative hash codes for streaming multi-modal data. Then, an online anchor-based manifold structure is designed to sparsely represent the old data and adaptively guide the hash code learning process, which can wellreduce the complexity in preserving the semantic correlation between the old data and streaming data. Meanwhile, such anchor-based manifold embedding is adaptive to the unsupervised and supervised learning strategies in a flexible way. Besides, an online discrete optimization method is efficiently addressed to incrementally update the hash functions and optimize the hash codes on streaming data points. As a result, the derived hash codes are more semantically meaningful for various online cross-modal retrieval tasks. Extensive experiments verify the advantages of the proposed OMGH model, by achieving and improving the state-of-the-art cross-modal retrieval performances on three benchmark datasets.
更多查看译文
关键词
Codes,Semantics,Hash functions,Manifolds,Correlation,Streaming media,Training,Cross-modal hashing,streaming data,Anchor-based manifold structure,online discrete optimization
AI 理解论文
溯源树
样例
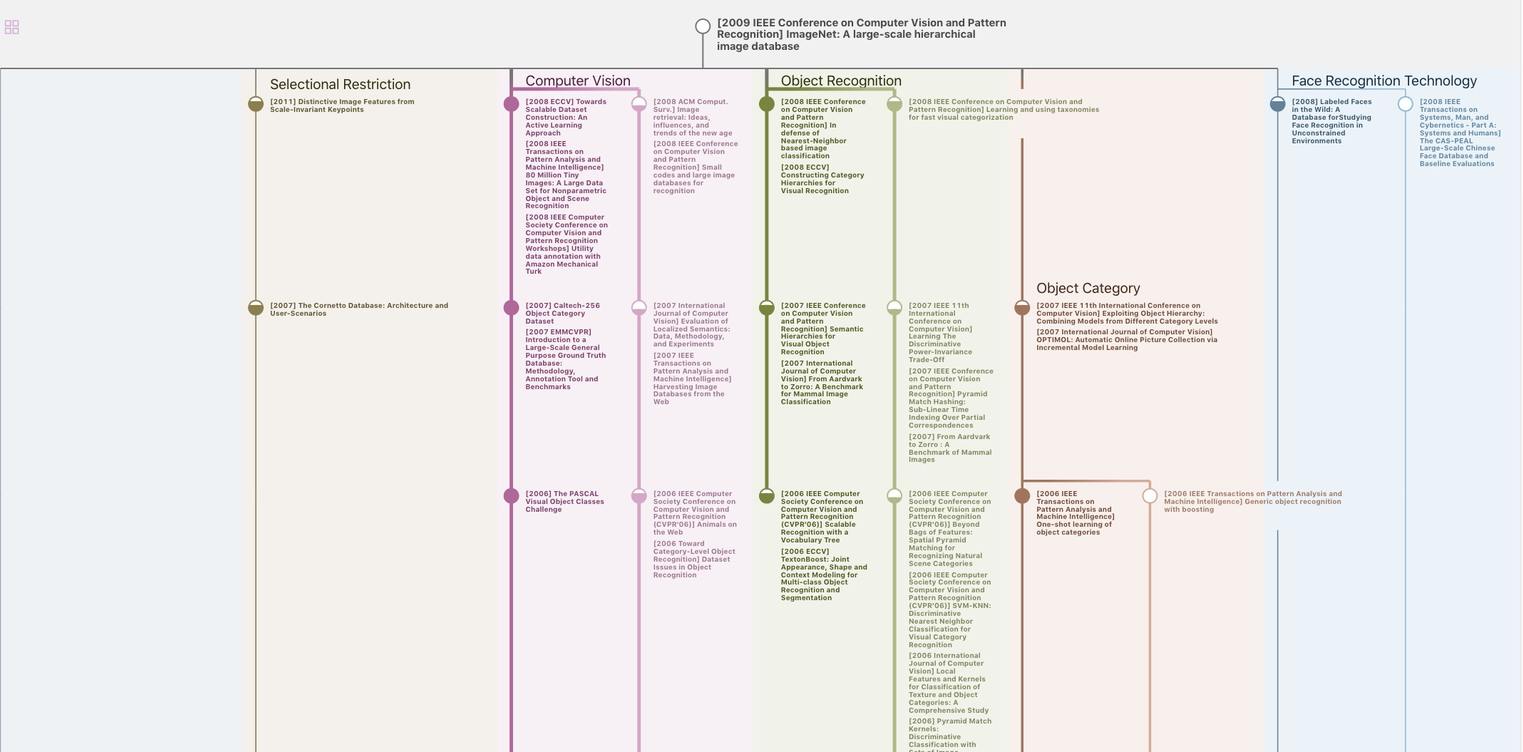
生成溯源树,研究论文发展脉络
Chat Paper
正在生成论文摘要