What makes forest-based heterogeneous treatment effect estimators work?
ANNALS OF APPLIED STATISTICS(2024)
摘要
Estimation of heterogeneous treatment effects (HTE) is of prime importance in many disciplines, from personalized medicine to economics among many others. Random forests have been shown to be a flexible and powerful approach to HTE estimation in both randomized trials and observational studies. In particular "causal forests" introduced by Athey, Tibshirani and Wager (Ann. Statist. 47 (2019) 1148-1178), along with the R implementation in package grf were rapidly adopted. A related approach, called "model -based forests" that is geared toward randomized trials and simultaneously captures effects of both prognostic and predictive variables, was introduced by Seibold, Zeileis and Hothorn (Stat. Methods Med. Res. 27 (2018) 3104-3125) along with a modular implementation in the R package model4you. Neither procedure is directly applicable to the estimation of individualized predictions of excess postpartum blood loss caused by a cesarean section in comparison to vaginal delivery. Clearly, randomization is hardly possible in this setup, and thus model -based forests lack clinical trial data to address this question. On the other hand, the skewed and interval -censored postpartum blood loss observations violate assumptions made by causal forests. Here we present a tailored model -based forest for skewed and interval -censored data to infer possible predictive prepartum characteristics and their impact on excess postpartum blood loss caused by a cesarean section. As a methodological basis, we propose a unifying view on causal and model -based forests that goes beyond the theoretical motivations and investigates which computational elements make causal forests so successful and how these can be blended with the strengths of model -based forests. To do so, we show that both methods can be understood in terms of the same parameters and model assumptions for an additive model under L2 loss. This theoretical insight allows us to implement several flavors of "model -based causal forests" and dissect their different elements in silico. The original causal forests and model -based forests are compared with the new blended versions in a benchmark study exploring both randomized trials and observational settings. In the randomized setting, both approaches performed akin. If confounding was present in the data -generating process, we found local centering of the treatment indicator with the corresponding propensities to be the main driver for good performance. Local centering of the outcome was less important and might be replaced or enhanced by simultaneous split selection with respect to both prognostic and predictive effects. This lays the foundation for future research combining random forests for HTE estimation with other types of models.
更多查看译文
关键词
Causal forests,heterogeneous treatment effects,observational data,personalized medicine,postpartum hemorrhage,random forest
AI 理解论文
溯源树
样例
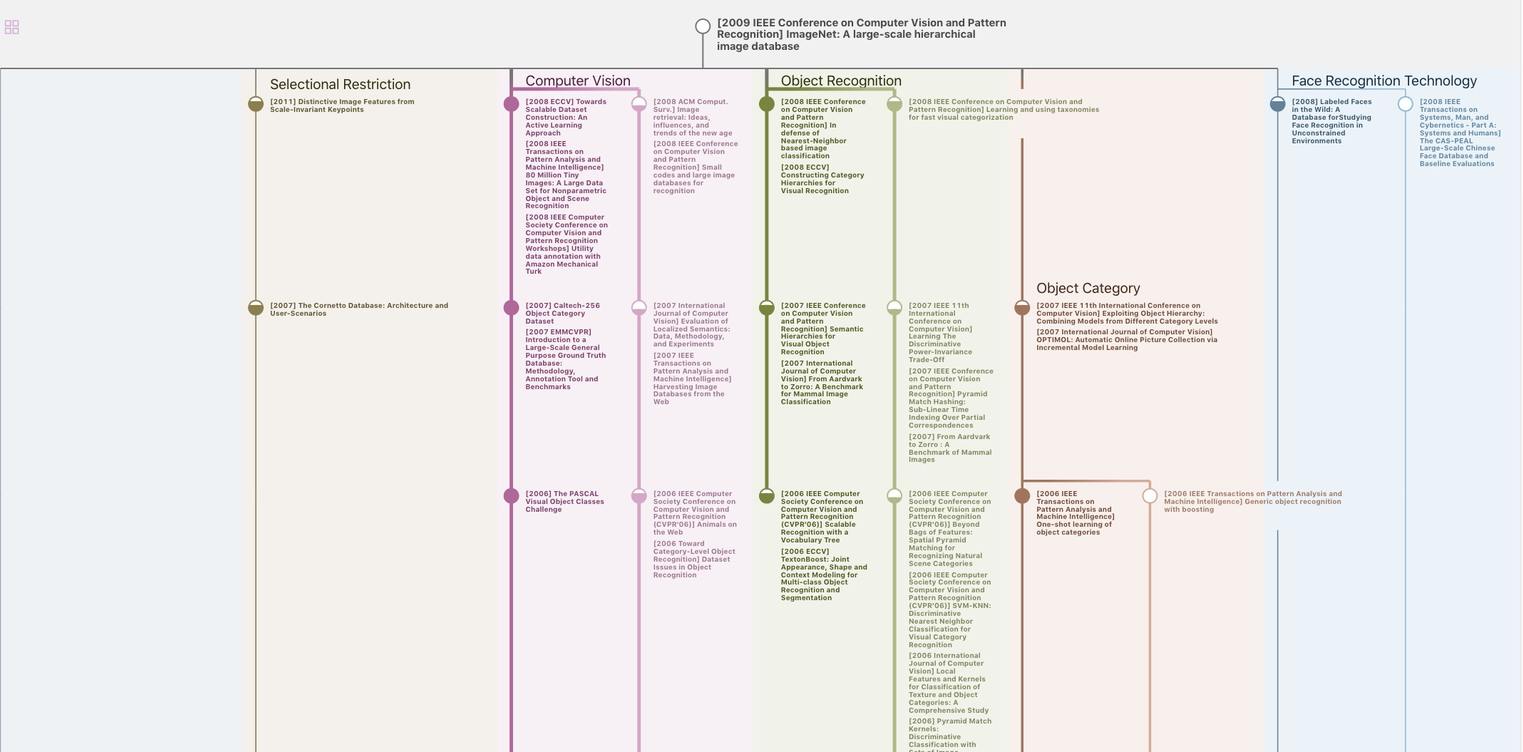
生成溯源树,研究论文发展脉络
Chat Paper
正在生成论文摘要