Supervision-Guided Codebooks for Masked Prediction in Speech Pre-training
Conference of the International Speech Communication Association (INTERSPEECH)(2022)
摘要
Recently, masked prediction pre-training has seen remarkable progress in self-supervised learning (SSL) for speech recognition. It usually requires a codebook obtained in an unsupervised way, making it less accurate and difficult to interpret. We propose two supervision-guided codebook generation approaches to improve automatic speech recognition (ASR) performance and also the pre-training efficiency, either through decoding with a hybrid ASR system to generate phoneme-level alignments (named PBERT), or performing clustering on the supervised speech features extracted from an end-to-end CTC model (named CTC clustering). Both the hybrid and CTC models are trained on the same small amount of labeled speech as used in fine-tuning. Experiments demonstrate significant superiority of our methods to various SSL and self-training baselines, with up to 17.0% relative WER reduction. Our pre-trained models also show good transferability in a non-ASR speech task.
更多查看译文
关键词
masked prediction,codebooks,speech,supervision-guided,pre-training
AI 理解论文
溯源树
样例
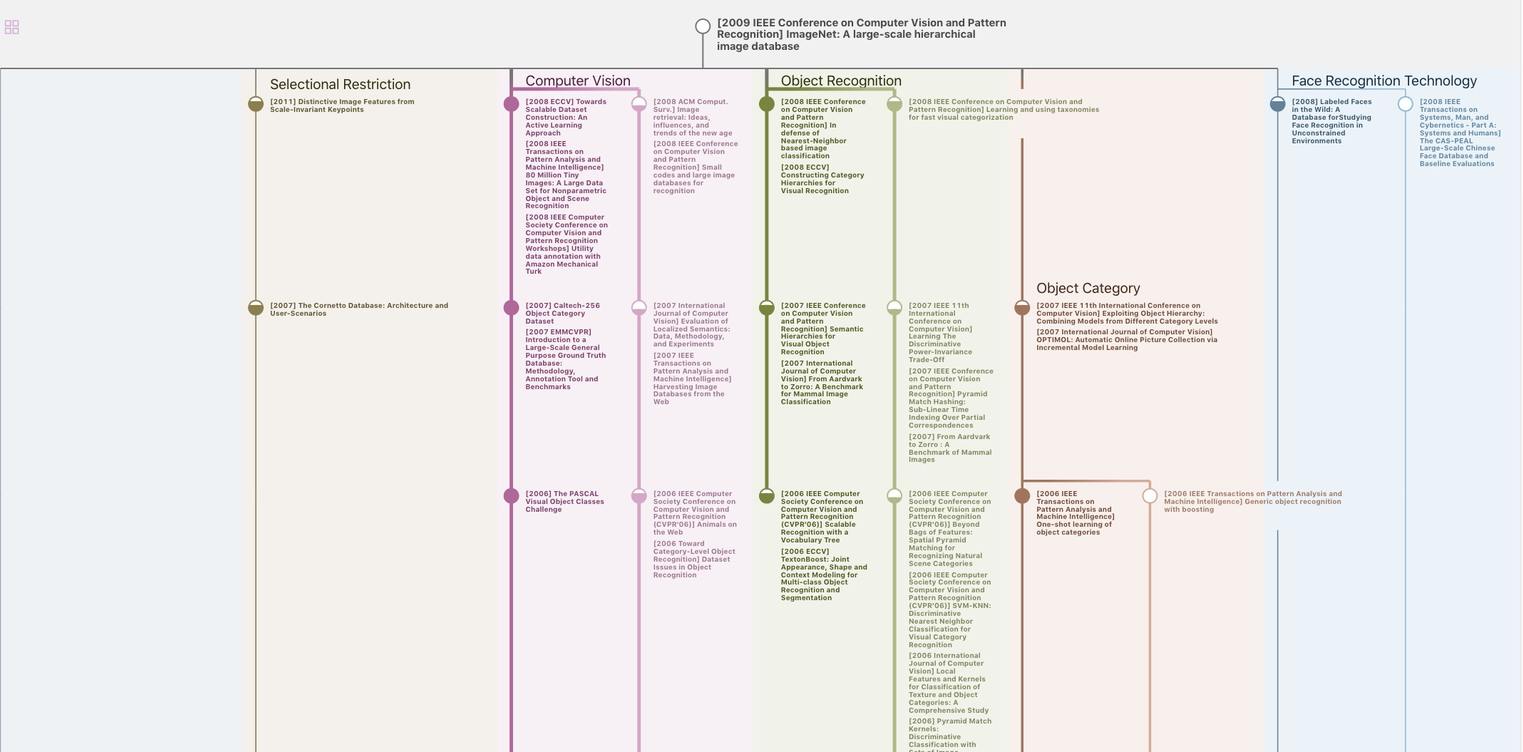
生成溯源树,研究论文发展脉络
Chat Paper
正在生成论文摘要