Meta-learning digitized-counterdiabatic quantum optimization
arxiv(2023)
摘要
The use of variational quantum algorithms for optimization tasks has emerged as a crucial application for the current noisy intermediate-scale quantum computers. However, these algorithms face significant difficulties in finding suitable ansatz and appropriate initial parameters. In this paper, we employ meta-learning using recurrent neural networks to address these issues for the recently proposed digitized-counterdiabatic quantum approximate optimization algorithm (QAOA). By combining meta-learning and counterdiabaticity, we find suitable variational parameters and reduce the number of optimization iterations required. We demonstrate the effectiveness of our approach by applying it to the MaxCut problem and the Sherrington-Kirkpatrick model. Our method offers a short-depth circuit ansatz with optimal initial parameters, thus improving the performance of the state-of-the-art QAOA.
更多查看译文
关键词
meta-learning,parameter concentration,shortcuts to adiabaticity (STA),counterdiabatic driving,QAOA,digitized-counterdiabatic quantum computing
AI 理解论文
溯源树
样例
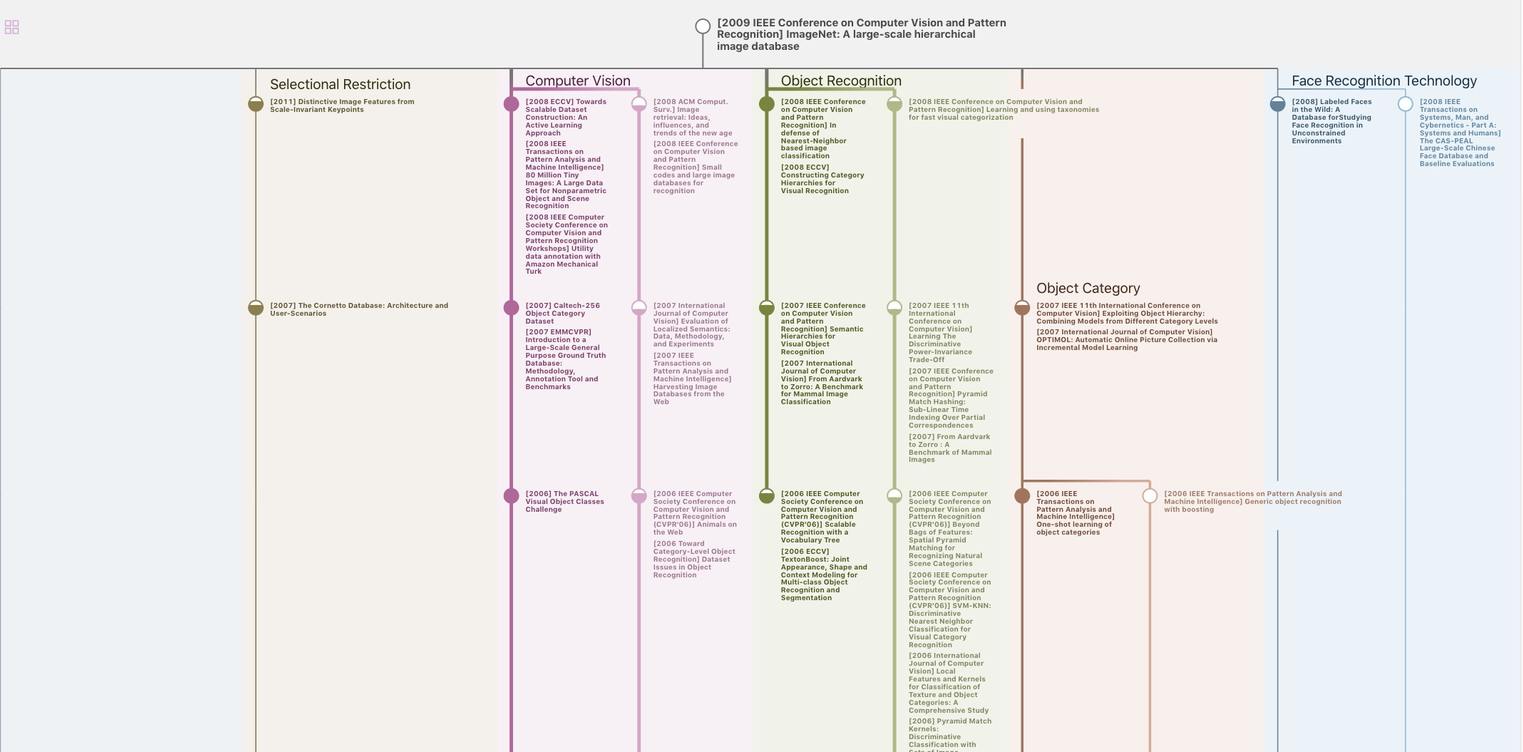
生成溯源树,研究论文发展脉络
Chat Paper
正在生成论文摘要