DualCoOp: Fast Adaptation to Multi-Label Recognition with Limited Annotations
NeurIPS 2022(2022)
摘要
Solving multi-label recognition (MLR) for images in the low-label regime is a challenging task with many real-world applications. Recent work learns an alignment between textual and visual spaces to compensate for insufficient image labels, but loses accuracy because of the limited amount of available MLR annotations. In this work, we utilize the strong alignment of textual and visual features pretrained with millions of auxiliary image-text pairs and propose Dual Context Optimization (DualCoOp) as a unified framework for partial-label MLR and zero-shot MLR. DualCoOp encodes positive and negative contexts with class names as part of the linguistic input (i.e. prompts). Since DualCoOp only introduces a very light learnable overhead upon the pretrained vision-language framework, it can quickly adapt to multi-label recognition tasks that have limited annotations and even unseen classes. Experiments on standard multi-label recognition benchmarks across two challenging low-label settings demonstrate the advantages of our approach over state-of-the-art methods.
更多查看译文
关键词
Multi-Label Recognition,Prompt Learning
AI 理解论文
溯源树
样例
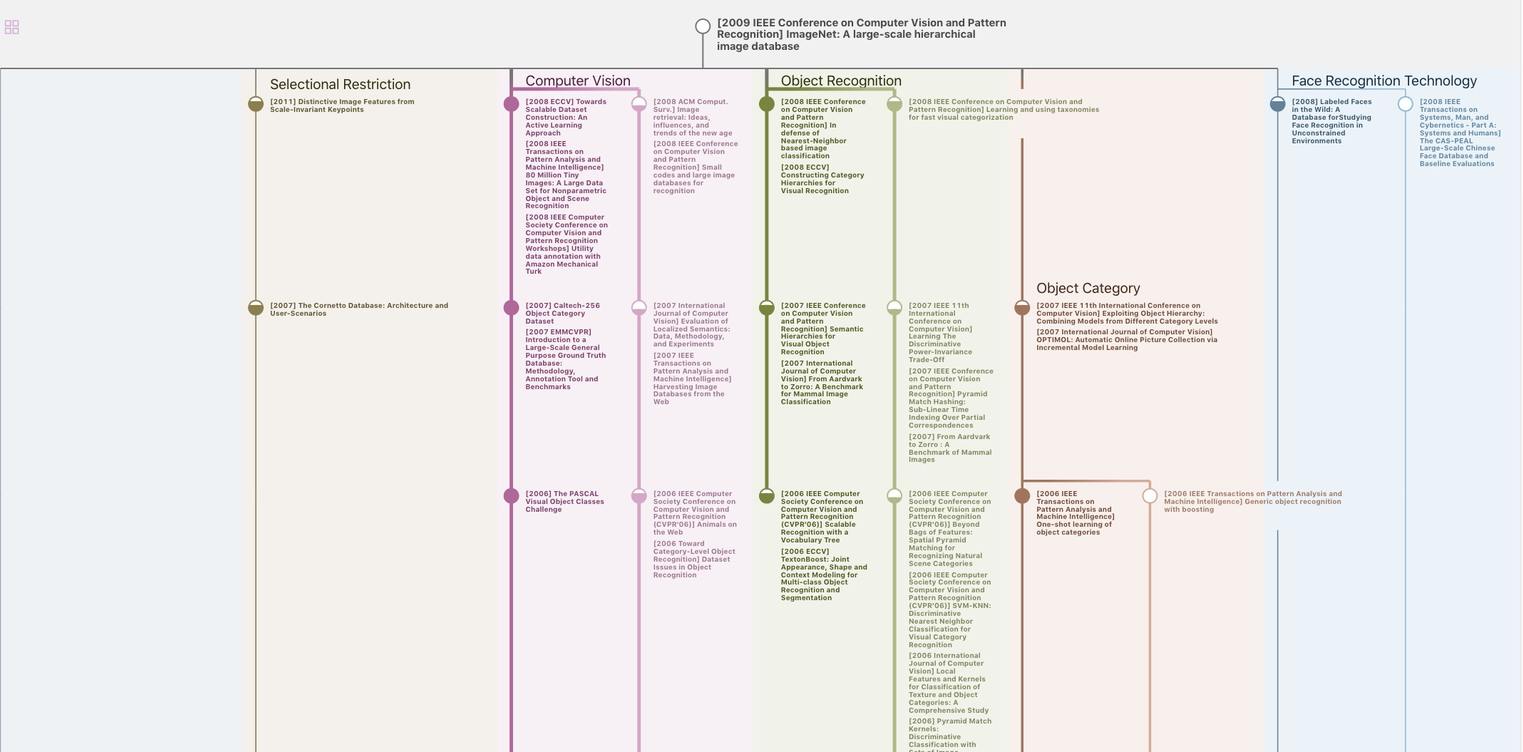
生成溯源树,研究论文发展脉络
Chat Paper
正在生成论文摘要