Simultaneous approximation of a smooth function and its derivatives by deep neural networks with piecewise-polynomial activations
NEURAL NETWORKS(2023)
摘要
This paper investigates the approximation properties of deep neural networks with piecewise-polynomial activation functions. We derive the required depth, width, and sparsity of a deep neural network to approximate any Holder smooth function up to a given approximation error in Holder norms in such a way that all weights of this neural network are bounded by 1. The latter feature is essential to control generalization errors in many statistical and machine learning applications.(c) 2023 Elsevier Ltd. All rights reserved.
更多查看译文
关键词
Deep neural networks,Approximation complexity,ReQU activations,ReLUk activations,H?lder class
AI 理解论文
溯源树
样例
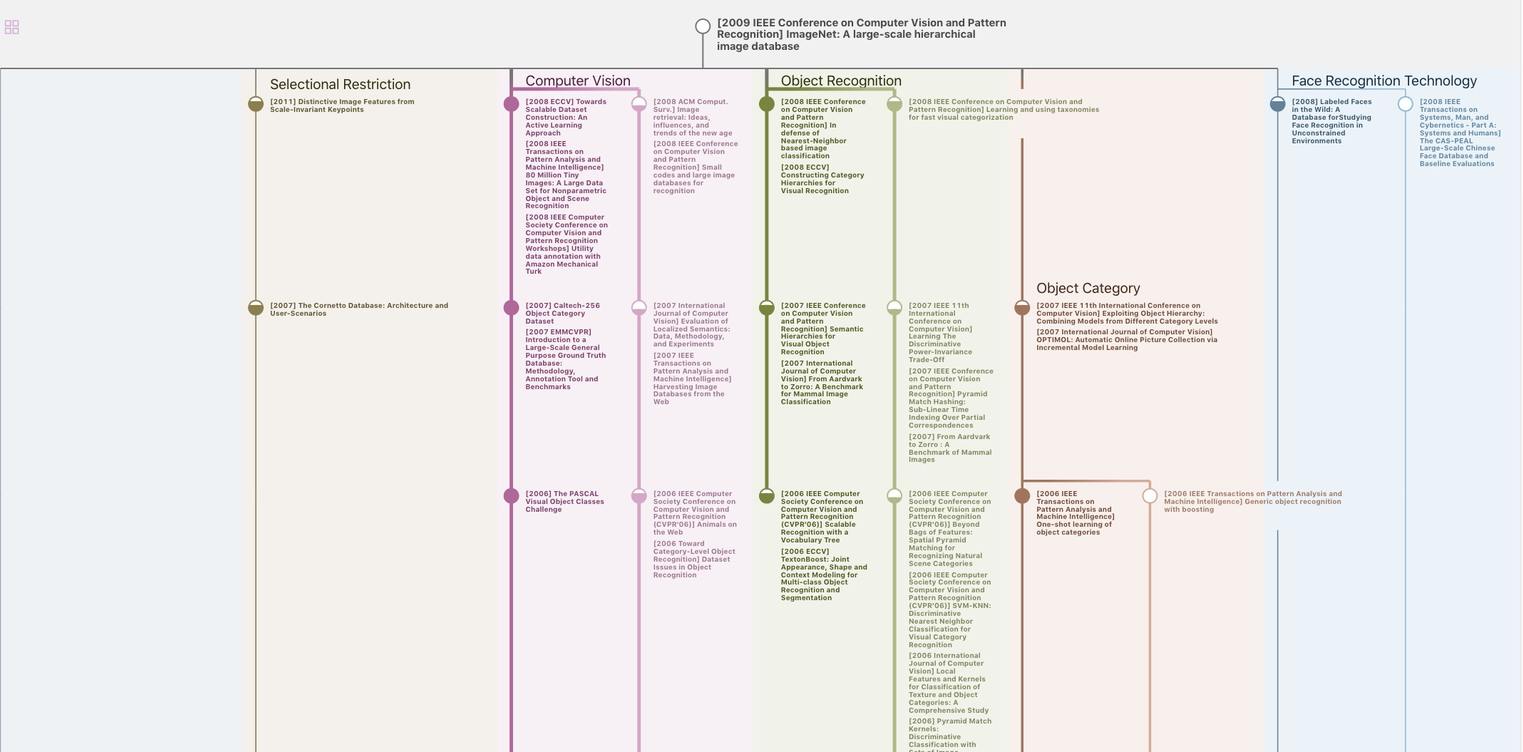
生成溯源树,研究论文发展脉络
Chat Paper
正在生成论文摘要