Quantifying Uncertainty In Traffic State Estimation Using Generative Adversarial Networks.
International Conference on Intelligent Transportation Systems (ITSC)(2022)
摘要
This paper aims to quantify uncertainty in traffic state estimation (TSE) using the generative adversarial network based physics-informed deep learning (PIDL). The uncertainty of the focus arises from fundamental diagrams, in other words, the mapping from traffic density to velocity. To quantify uncertainty for the TSE problem is to characterize the robustness of predicted traffic states. Since its inception, generative adversarial networks (GAN) have become a popular probabilistic machine learning framework. In this paper, we will inform the GAN based predictions using stochastic traffic flow models and develop a GAN based PIDL framework for TSE, named ``PhysGAN-TSE". By conducting experiments on a real-world dataset, the Next Generation SIMulation (NGSIM) dataset, this method is shown to be more robust for uncertainty quantification than the pure GAN model or pure traffic flow models. Two physics models, the Lighthill-Whitham-Richards (LWR) and the Aw-Rascle-Zhang (ARZ) models, are compared as the physics components for the PhysGAN, and results show that the ARZ-based PhysGAN achieves a better performance than the LWR-based one.
更多查看译文
关键词
traffic state estimation,state estimation,uncertainty,generative
AI 理解论文
溯源树
样例
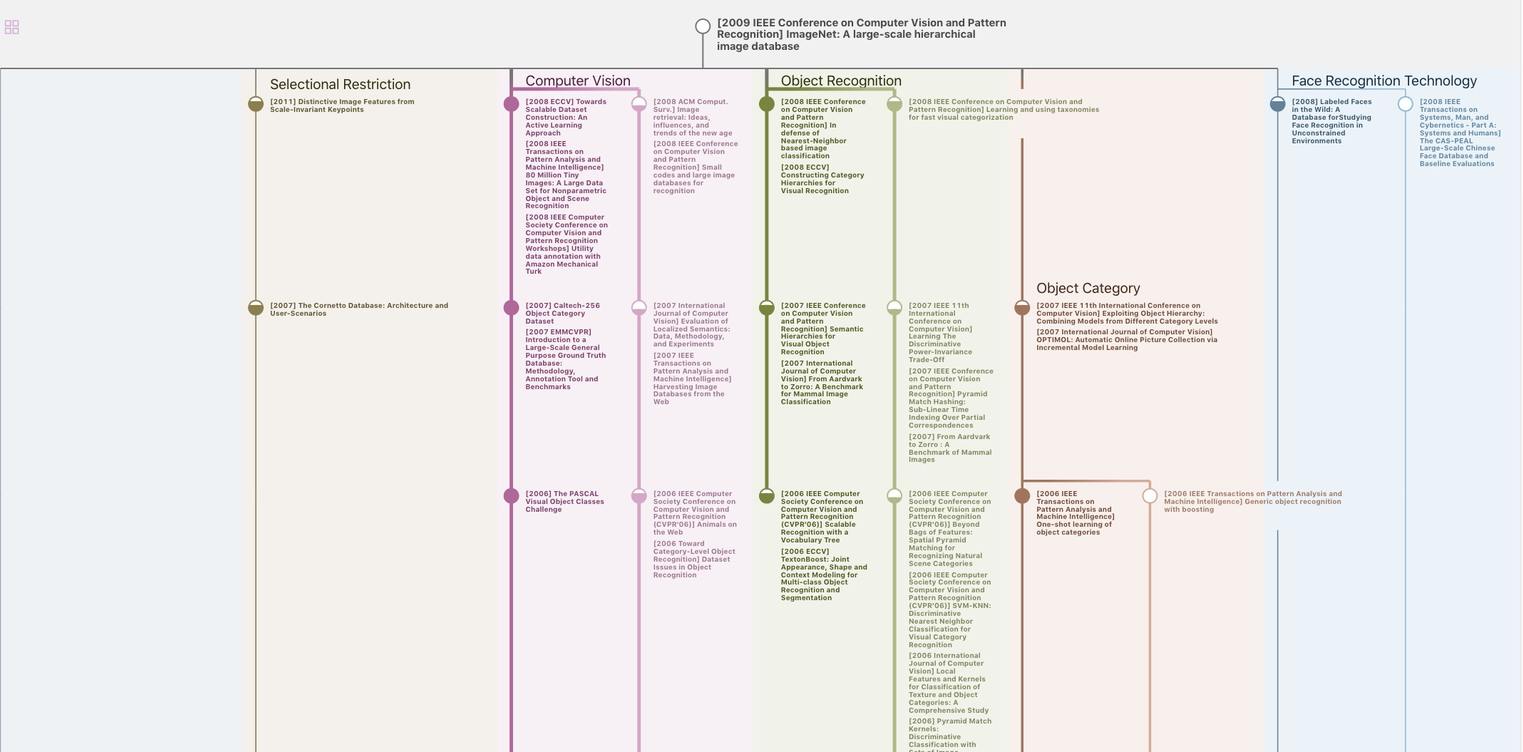
生成溯源树,研究论文发展脉络
Chat Paper
正在生成论文摘要