TrafficFlowGAN: Physics-informed Flow based Generative Adversarial Network for Uncertainty Quantification
arxiv(2022)
摘要
This paper proposes the TrafficFlowGAN, a physics-informed flow based generative adversarial network (GAN), for uncertainty quantification (UQ) of dynamical systems. TrafficFlowGAN adopts a normalizing flow model as the generator to explicitly estimate the data likelihood. This flow model is trained to maximize the data likelihood and to generate synthetic data that can fool a convolutional discriminator. We further regularize this training process using prior physics information, so-called physics-informed deep learning (PIDL). To the best of our knowledge, we are the first to propose an integration of flow, GAN and PIDL for the UQ problems. We take the traffic state estimation (TSE), which aims to estimate the traffic variables (e.g. traffic density and velocity) using partially observed data, as an example to demonstrate the performance of our proposed model. We conduct numerical experiments where the proposed model is applied to learn the solutions of stochastic differential equations. The results demonstrate the robustness and accuracy of the proposed model, together with the ability to learn a machine learning surrogate model. We also test it on a real-world dataset, the Next Generation SIMulation (NGSIM), to show that the proposed TrafficFlowGAN can outperform the baselines, including the pure flow model, the physics-informed flow model, and the flow based GAN model.
更多查看译文
关键词
Uncertainty Quantification (UQ), Normalizing flow, Generative Adversarial Networks (GAN), Physics-informed Deep Learning (PIDL)
AI 理解论文
溯源树
样例
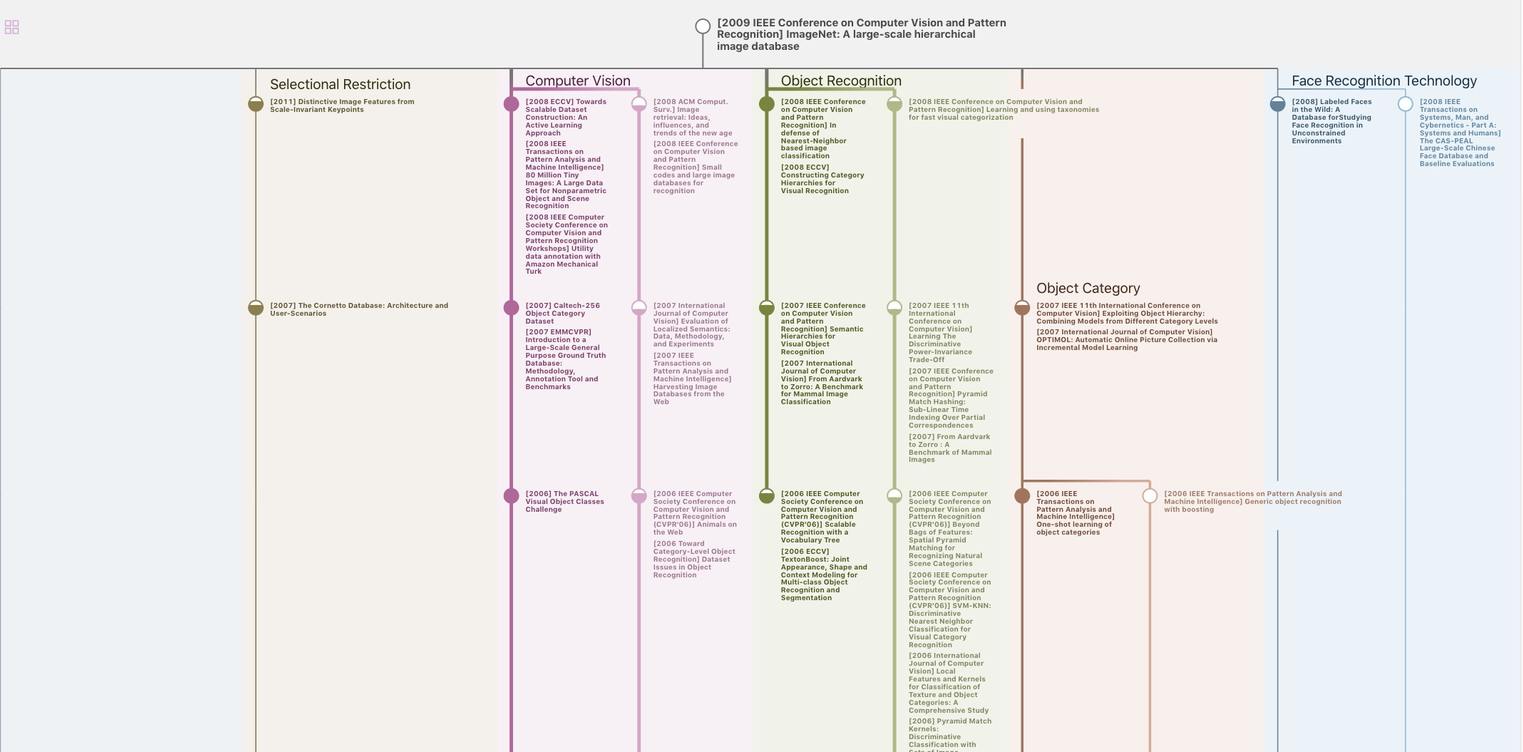
生成溯源树,研究论文发展脉络
Chat Paper
正在生成论文摘要