Provable Generalization of Overparameterized Meta-learning Trained with SGD
NeurIPS 2022(2022)
摘要
Despite the superior empirical success of deep meta-learning, theoretical understanding of overparameterized meta-learning is still limited. This paper studies the generalization of a widely used meta-learning approach, Model-Agnostic Meta-Learning (MAML), which aims to find a good initialization for fast adaptation to new tasks. Under a mixed linear regression model, we analyze the generalization properties of MAML trained with SGD in the overparameterized regime. We provide both upper and lower bounds for the excess risk of MAML, which captures how SGD dynamics affect these generalization bounds. With such sharp characterizations, we further explore how various learning parameters impact the generalization capability of overparameterized MAML, including explicitly identifying typical data and task distributions that can achieve diminishing generalization error with overparameterization, and characterizing the impact of adaptation learning rate on both excess risk and the early stopping time. Our theoretical findings are further validated by experiments.
更多查看译文
关键词
MAML,generalization theory,excess risk,mixed linear regression
AI 理解论文
溯源树
样例
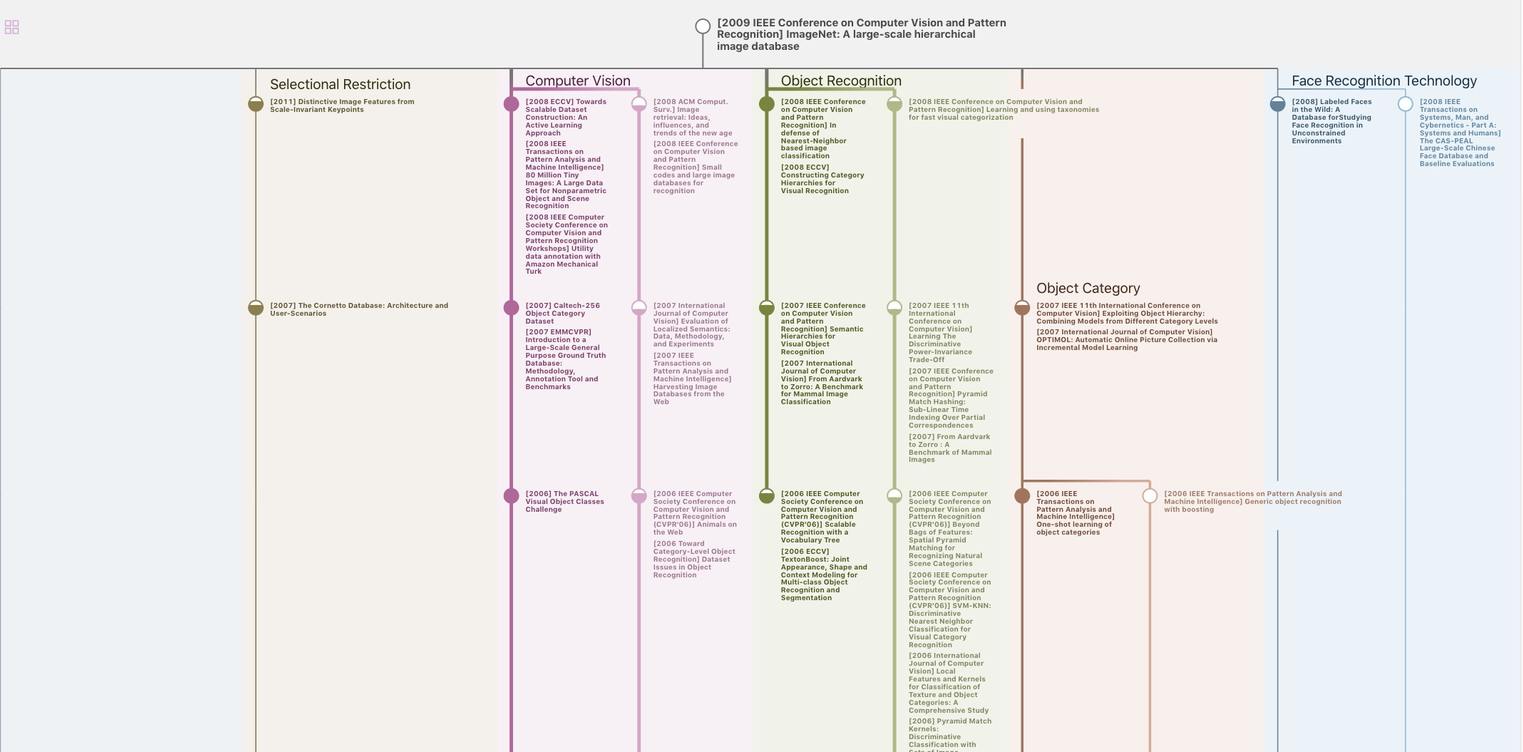
生成溯源树,研究论文发展脉络
Chat Paper
正在生成论文摘要