Confusion-Based Metric Learning for Regularizing Zero-Shot Image Retrieval and Clustering
IEEE TRANSACTIONS ON NEURAL NETWORKS AND LEARNING SYSTEMS(2024)
摘要
Deep metric learning turns to be attractive in zero-shot image retrieval and clustering (ZSRC) task in which a good embedding/metric is requested such that the unseen classes can be distinguished well. Most existing works deem this "good" embedding just to be the discriminative one and race to devise the powerful metric objectives or the hard-sample mining strategies for learning discriminative deep metrics. However, in this article, we first emphasize that the generalization ability is also a core ingredient of this "good" metric and it largely affects the metric performance in zero-shot settings as a matter of fact. Then, we propose the confusion-based metric learning (CML) framework to explicitly optimize a robust metric. It is mainly achieved by introducing two interesting regularization terms, i.e., the energy confusion (EC) and diversity confusion (DC) terms. These terms daringly break away from the traditional deep metric learning idea of designing discriminative objectives and instead seek to "confuse" the learned model. These two confusion terms focus on local and global feature distribution confusions, respectively. We train these confusion terms together with the conventional deep metric objective in an adversarial manner. Although it seems weird to "confuse" the model learning, we show that our CML indeed serves as an efficient regularization framework for deep metric learning and it is applicable to various conventional metric methods. This article empirically and experimentally demonstrates the importance of learning an embedding/metric with good generalization, achieving the state-of-the-art performances on the popular CUB, CARS, Stanford Online Products, and In-Shop datasets for ZSRC tasks.
更多查看译文
关键词
Measurement,Task analysis,Training,Learning systems,Image retrieval,Head,Semantics,Confusion,generalization,image retrieval,clustering,regularization,zero-shot learning (ZSL)
AI 理解论文
溯源树
样例
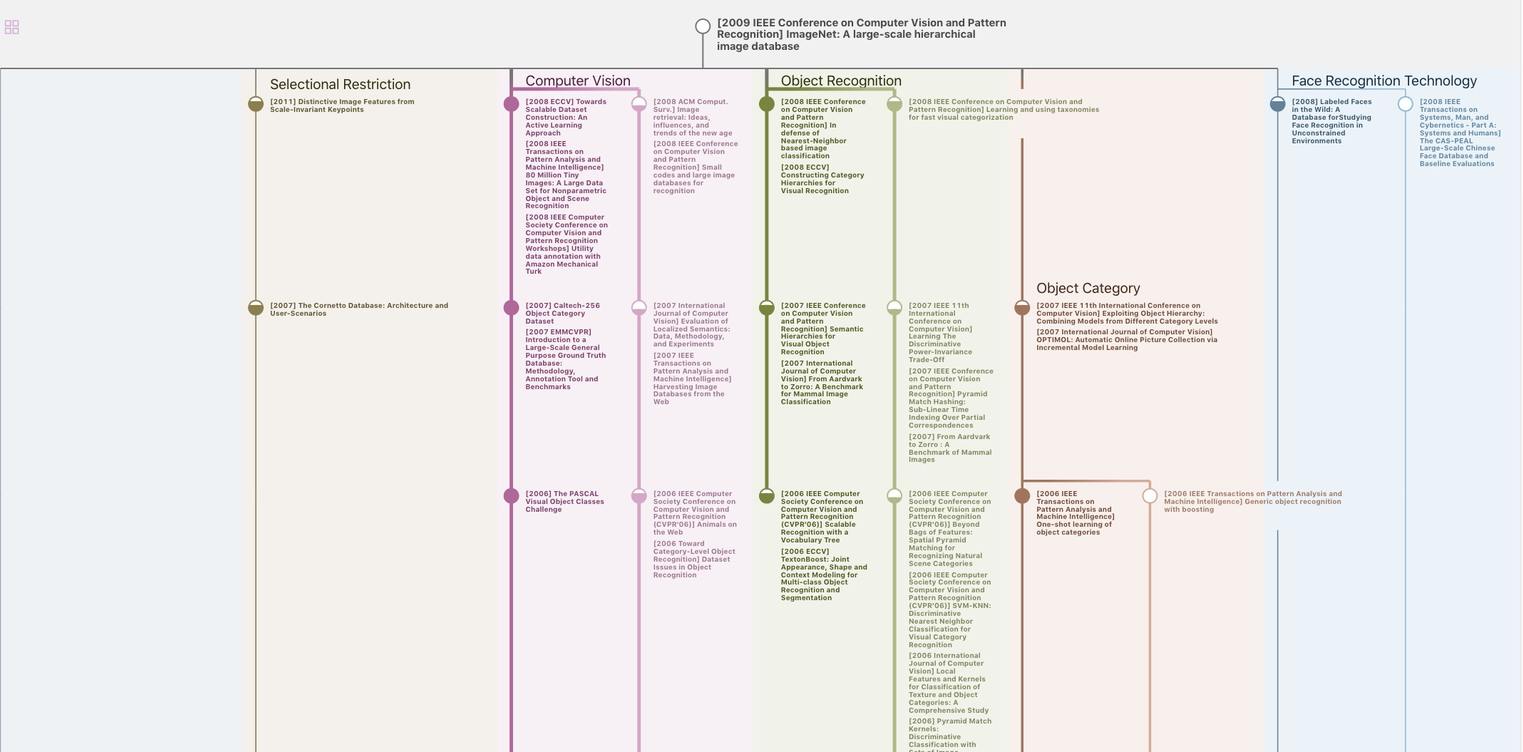
生成溯源树,研究论文发展脉络
Chat Paper
正在生成论文摘要