A physics-informed neural network-based surrogate framework to predict moisture concentration and shrinkage of a plant cell during drying
Journal of Food Engineering(2022)
摘要
This paper presents a Physics-Informed Neural Network-based (PINN-based) surrogate framework, which can couple time-based moisture concentration and moisture-content-based shrinkage of a plant cell during drying. For this, a set of differential equations are coupled to two distinct multilayer feedforward neural networks: (a) PINN-MC to predict Moisture Concentration (MC) with Fick's law of diffusion; and (b) PINN-S to predict Shrinkage (S) with ‘free shrinkage’ hypothesis. Results indicate that compared to a regular deep neural network (DNN), the PINN-MC with fundamental physics guidance produces 53% and 81% accuracy values when unknown data has the lowest five timesteps and the lowest 27 data points, respectively. Moreover, its accuracy is 80% better when predicting any unknown spatiotemporal domain variations. PINN-MC further demonstrates stable and accurate MC predictions irrespective of drying process parameters and microstructural variations. In addition, the PINN-S separately proves that utilising a derived relationship based on the ‘free shrinkage’ hypothesis can improve shrinkage predictions into a realistic behaviour. Also, the PINN-based surrogate framework combines multiple physics for predicting moisture concentration and shrinkage, reassuring its capability as a powerful tool for investigating complicated drying mechanisms. Accordingly, to the best of the authors' knowledge, this surrogate framework is the first of its kind in food engineering applications.
更多查看译文
关键词
Deep neural network,Food drying,Moisture concentration,Physics-informed neural network,Shrinkage,Surrogate framework
AI 理解论文
溯源树
样例
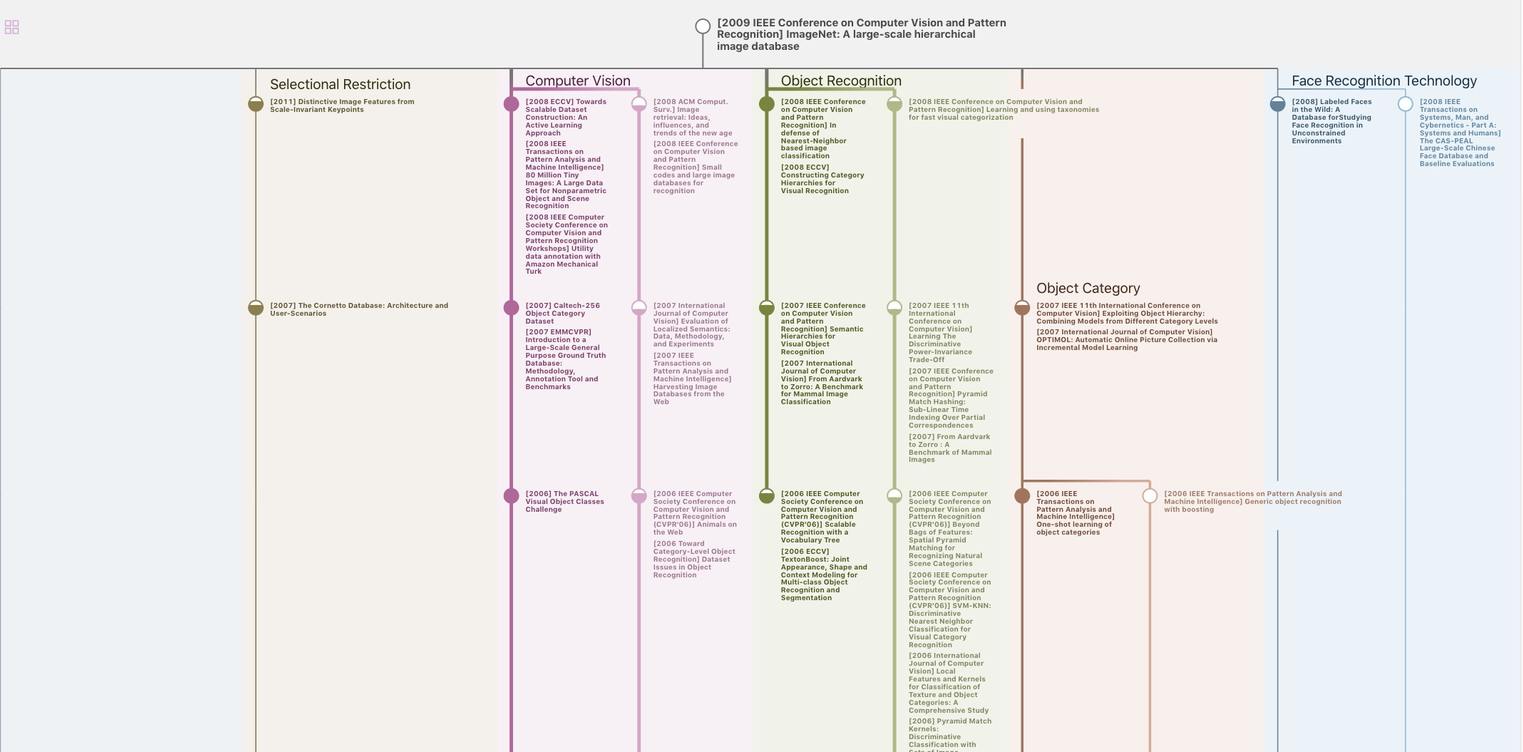
生成溯源树,研究论文发展脉络
Chat Paper
正在生成论文摘要