Global and local interaction matching model for knowledge-grounded response selection in retrieval-based chatbots
Neurocomputing(2022)
摘要
Knowledge-grounded response selection, the task of selecting a proper response from a set of response candidates given the conversation context and its background knowledge, is important for building intelligent dialogue systems. Challenges for the task mainly lie in how to interact between the context and the knowledge, and how to match between the response and the context-knowledge pair. Existing work mainly focuses on exploiting global or local interaction matching information, and hence cannot fully utilize the information. In this paper, we propose a global and local interaction matching model (GLIMM) that matches a response with the context-knowledge pair from global and local views. For global interaction matching, GLIMM takes the context and the knowledge as long sequences. For local interaction matching, GLIMM treats each context utterance and knowledge sentence separately. GLIMM integrates information from two different views that could provide complementary information for each other. Experiments on two datasets show that the proposed model outperforms the state-of-the-art baselines.
更多查看译文
关键词
Response selection,Knowledge,Global and local views,Matching,Deep neural networks
AI 理解论文
溯源树
样例
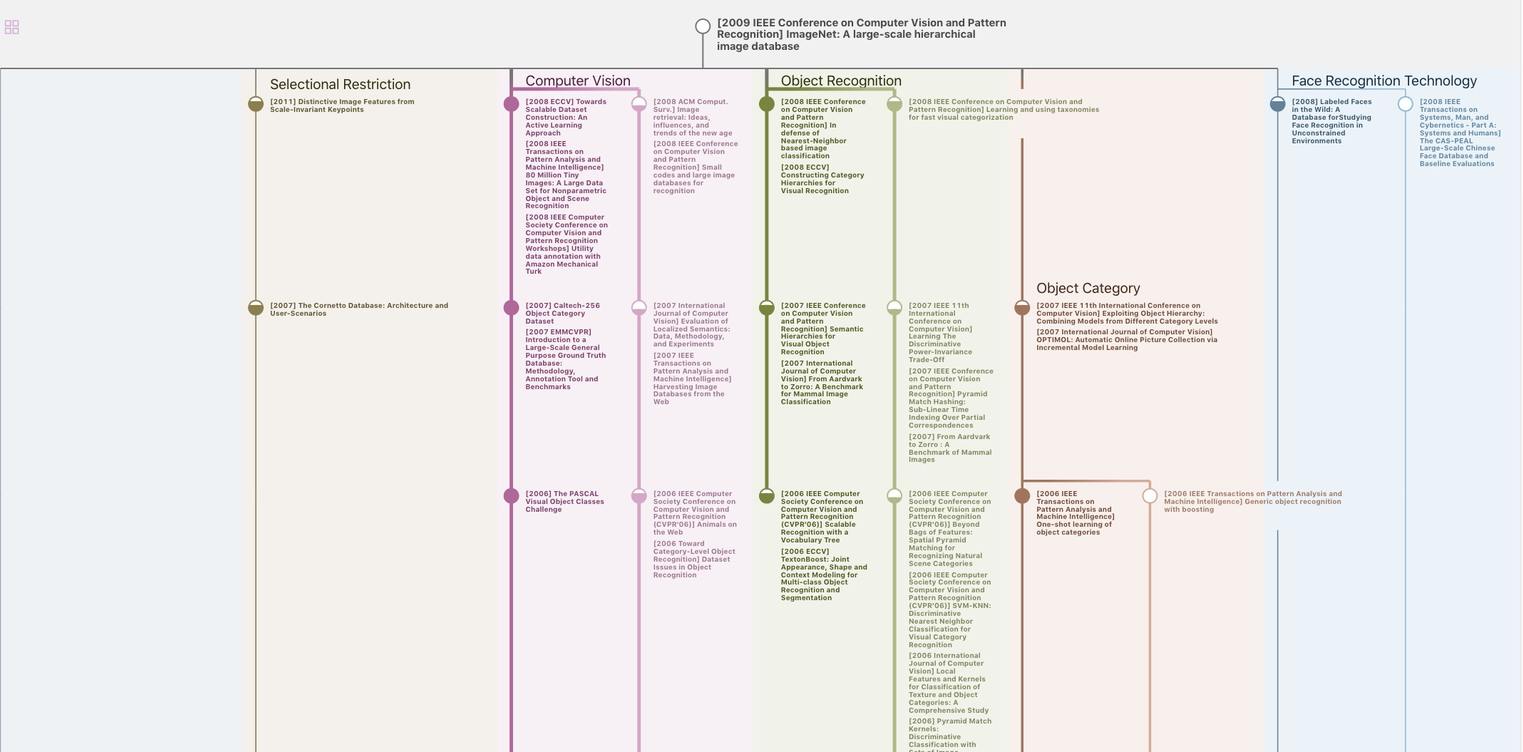
生成溯源树,研究论文发展脉络
Chat Paper
正在生成论文摘要