A Generalized Deep Learning Algorithm Based on NMF for Multi-View Clustering
IEEE Transactions on Big Data(2023)
摘要
Multi-view clustering research is a hot topic in the field of data mining, where complementary information between views can better describe data objects and improve the clustering performance. Non-negative matrix factorization (NMF) based multi-view clustering algorithm suffers from weak feature extraction, slow convergence speed and low accuracy. To solve these problems, this paper proposes a generalized deep learning multi-view clustering (GDLMC) algorithm based on NMF. Firstly, via decoupling the elements in the matrix, the matrix elements are non-negatively restricted using an activation function with a non-negative value domain, and the elements are updated employing stochastic gradient descent with learning rate guidance. Then, the corresponding gradients when the elements update are transformed into generalized weights and generalized biases, followed by combining the generalized weights and generalized biases with activation functions to construct generalized deep learning (GDL). Further, GDL is adopted to learn the corresponding low-dimensional matrix of each view and consensus matrix for obtaining the GDLMC algorithm. In addition, the detailed reasoning of the GDLMC algorithm are given. Finally, extensive experiments are conducted on four public datasets including regular and large-scale datasets, and the experimental results show that GDLMC has significant advantages.
更多查看译文
关键词
Multi-view clustering,stochastic gradient descent,non-negative matrix factorization,deep learning
AI 理解论文
溯源树
样例
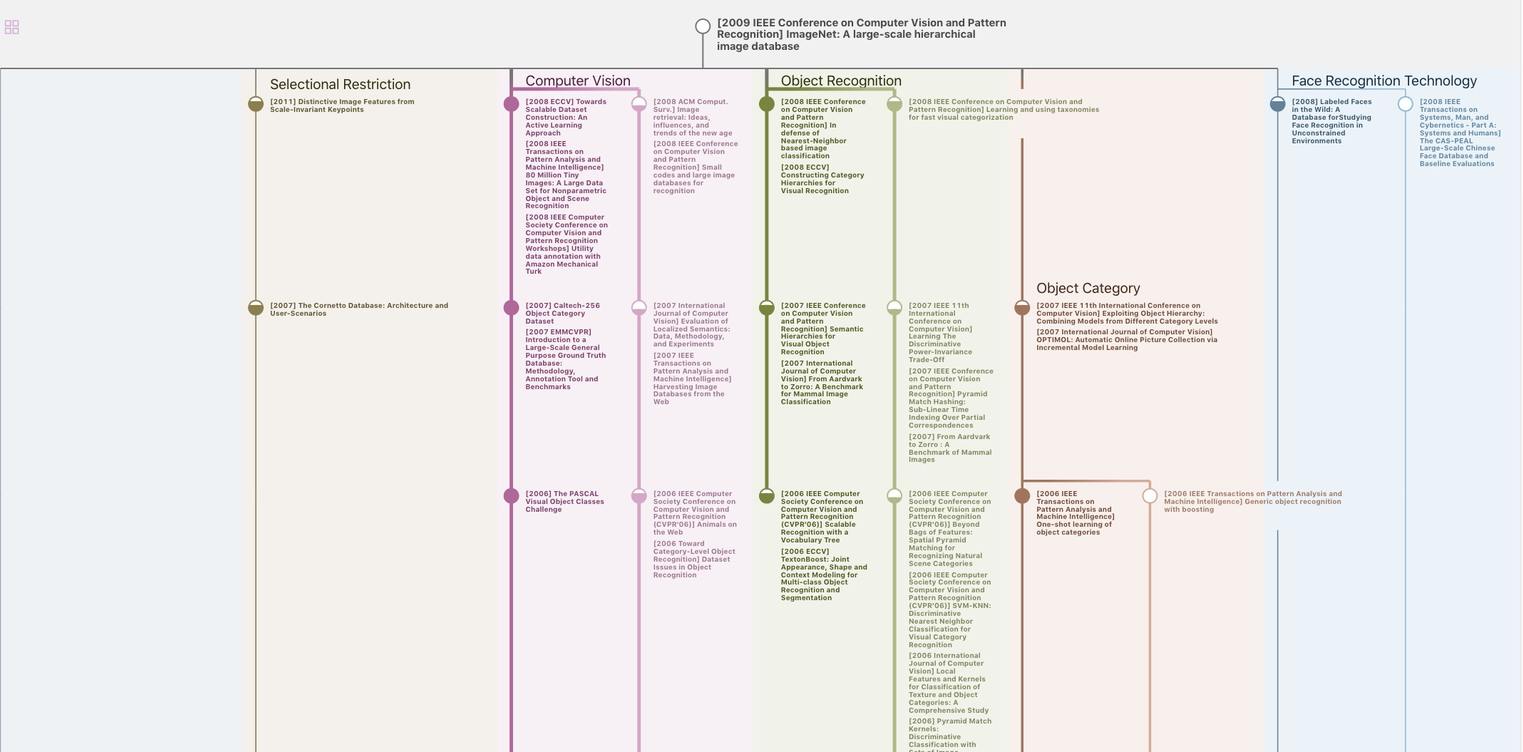
生成溯源树,研究论文发展脉络
Chat Paper
正在生成论文摘要