Limit surface/states searching algorithm with a deep neural network and Monte Carlo dropout for nuclear power plant safety assessment
Applied Soft Computing(2022)
摘要
For decades, efforts have been made to enhance the realism of risk assessment methods for nuclear power plants (NPPs) by augmenting the number of representative scenarios. The common issue though is that each scenario should be simulated individually by computationally demanding physical models such as thermal-hydraulic system codes. To address this problem, this research proposes an algorithm that identifies the success or failure of each scenario with a minimized number of simulations. The algorithm seeks to locate the limit surface/states that distinguishes success and failure regions and impels the physical models to simulate only the scenarios where the consequence is close to the given failure criterion. To this end, the algorithm is an iterative process that estimates the LS by a metamodel, samples the scenarios close to the estimated LS, simulates the sampled scenarios, and trains the metamodel with accumulated simulation results. A deep neural network (DNN) is employed as the metamodel for predicting the consequence of each scenario, and Monte Carlo dropout informs the predictive uncertainty. Referring to this uncertainty, the DNN metamodel meticulously ‘sails’ across the scenario space to accurately pinpoint the LS. We name the proposed algorithm the deep neural network-based searching algorithm of informative limit surfaces and states, or Deep-SAILS. Combining the simulation results of risk-sensitive scenarios (close to the LS) and the ability of the trained DNN metamodel to reasonably predict the non-simulated scenarios, Deep-SAILS identified the success or the failure of tens of thousands of dynamic scenarios of loss of coolant accidents at more than 99% accuracy with only thousands of simulations.
更多查看译文
关键词
Nuclear power plant,Reliability,Limit surface,Critical failure region,Deep neural network,Active learning,Adaptive sampling,Loss of coolant accident
AI 理解论文
溯源树
样例
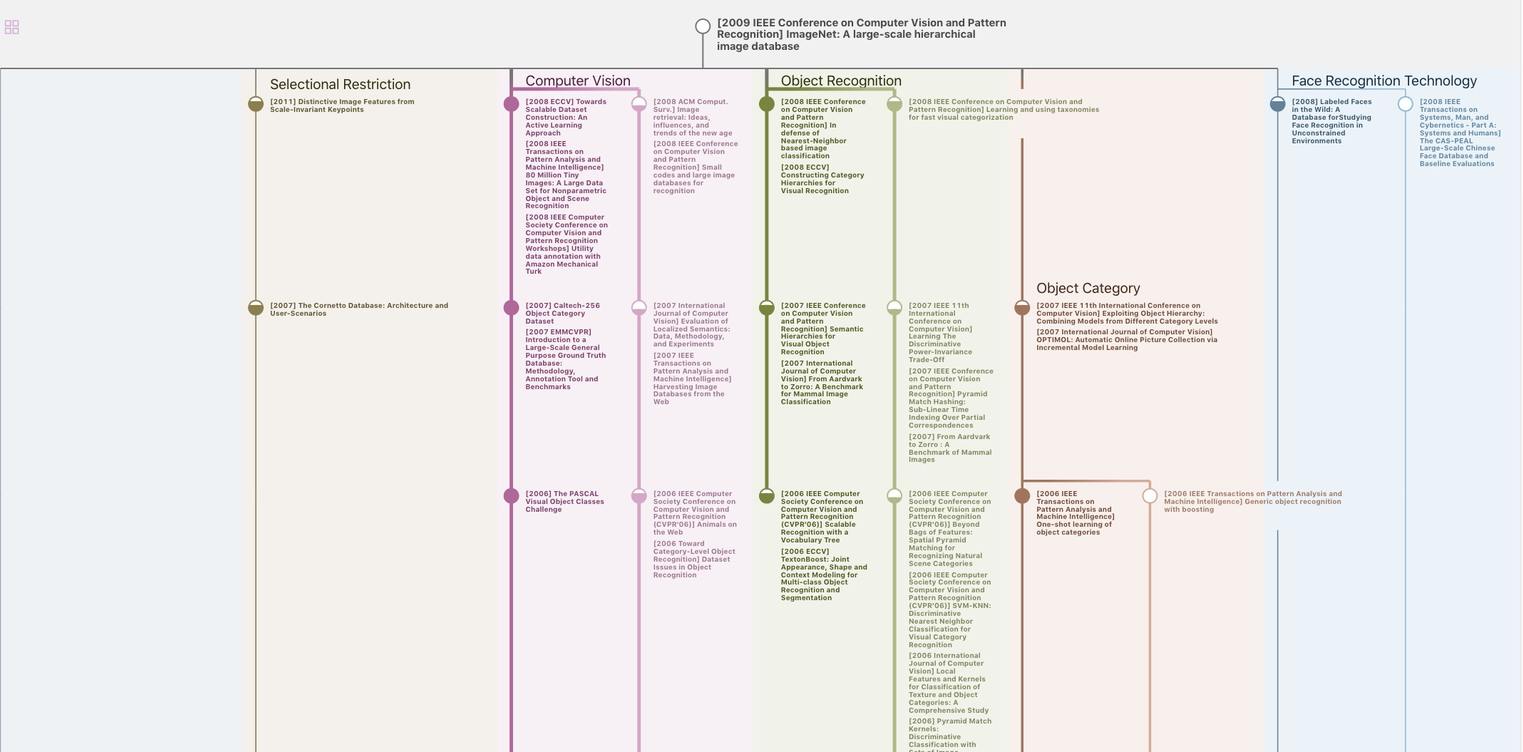
生成溯源树,研究论文发展脉络
Chat Paper
正在生成论文摘要