Reinforcement Learning Model With Dynamic State Space Tested on Target Search Tasks for Monkeys: Extension to Learning Task Events
FRONTIERS IN COMPUTATIONAL NEUROSCIENCE(2022)
摘要
Learning is a crucial basis for biological systems to adapt to environments. Environments include various states or episodes, and episode-dependent learning is essential in adaptation to such complex situations. Here, we developed a model for learning a two-target search task used in primate physiological experiments. In the task, the agent is required to gaze one of the four presented light spots. Two neighboring spots are served as the correct target alternately, and the correct target pair is switched after a certain number of consecutive successes. In order for the agent to obtain rewards with a high probability, it is necessary to make decisions based on the actions and results of the previous two trials. Our previous work achieved this by using a dynamic state space. However, to learn a task that includes events such as fixation to the initial central spot, the model framework should be extended. For this purpose, here we propose a "history-in-episode architecture." Specifically, we divide states into episodes and histories, and actions are selected based on the histories within each episode. When we compared the proposed model including the dynamic state space with the conventional SARSA method in the two-target search task, the former performed close to the theoretical optimum, while the latter never achieved target-pair switch because it had to re-learn each correct target each time. The reinforcement learning model including the proposed history-in-episode architecture and dynamic state scape enables episode-dependent learning and provides a basis for highly adaptable learning systems to complex environments.
更多查看译文
关键词
reinforcement learning, target search task, dynamic state space, episode-dependent learning, history-in-episode architecture
AI 理解论文
溯源树
样例
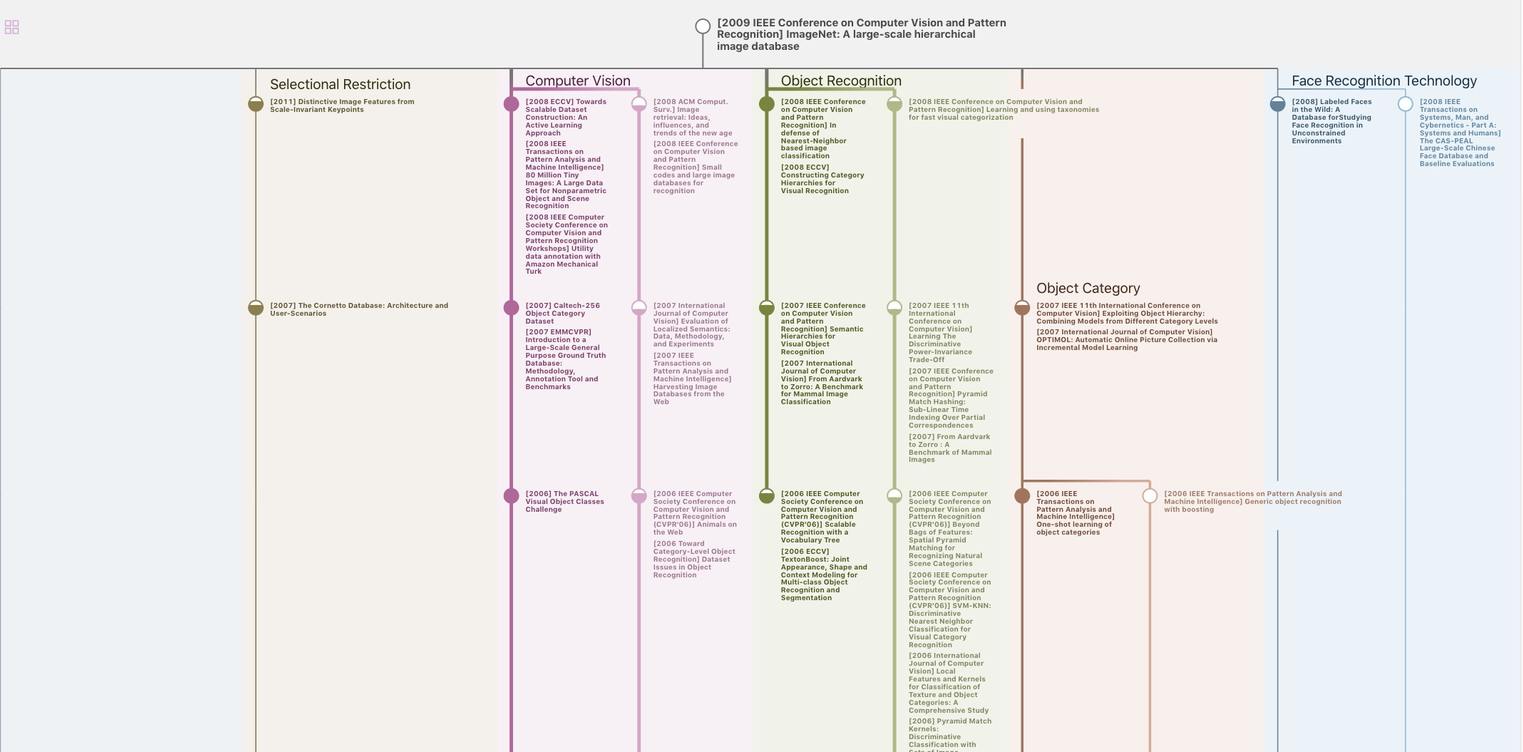
生成溯源树,研究论文发展脉络
Chat Paper
正在生成论文摘要