CAU-Net: A Deep Learning Method for Deep Gray Matter Nuclei Segmentation
FRONTIERS IN NEUROSCIENCE(2022)
摘要
The abnormal iron deposition of the deep gray matter nuclei is related to many neurological diseases. With the quantitative susceptibility mapping (QSM) technique, it is possible to quantitatively measure the brain iron content in vivo. To assess the magnetic susceptibility of the deep gray matter nuclei in the QSM, it is mandatory to segment the nuclei of interest first, and many automatic methods have been proposed in the literature. This study proposed a contrast attention U-Net for nuclei segmentation and evaluated its performance on two datasets acquired using different sequences with different parameters from different MRI devices. Experimental results revealed that our proposed method was superior on both datasets over other commonly adopted network structures. The impacts of training and inference strategies were also discussed, which showed that adopting test time augmentation during the inference stage can impose an obvious improvement. At the training stage, our results indicated that sufficient data augmentation, deep supervision, and nonuniform patch sampling contributed significantly to improving the segmentation accuracy, which indicated that appropriate choices of training and inference strategies were at least as important as designing more advanced network structures.
更多查看译文
关键词
convolutional neural network (CNN), deep learning, medical image segmentation, gray matter nuclei, quantitative susceptibility mapping, strategically acquired gradient echo (STAGE) imaging
AI 理解论文
溯源树
样例
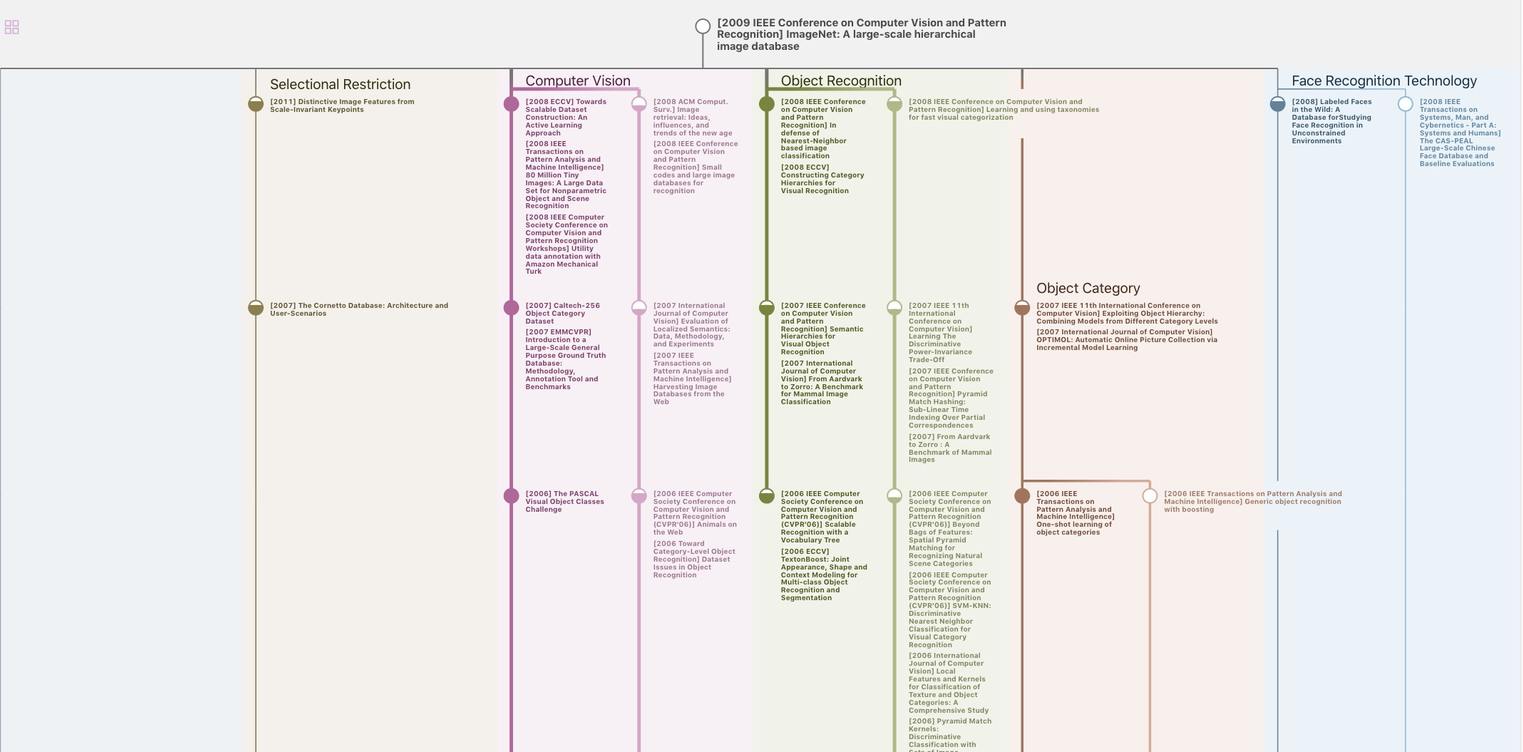
生成溯源树,研究论文发展脉络
Chat Paper
正在生成论文摘要