Maize yield forecasts for Sub-Saharan Africa using Earth Observation data and machine learning
Global Food Security(2022)
摘要
Food insecurity continues to grow in Sub-Saharan Africa (SSA). In 2019, chronically malnourished people numbered nearly 240 million, or 20% of the population in SSA. Globally, numerous efforts have been made to anticipate potential droughts, crop conditions, and food shortages in order to improve early warning and risk management for food insecurity. To support this goal, we develop an Earth Observation (EO) and machine-learning-based operational, subnational maize yield forecast system and evaluate its out-of-sample forecast skills during the growing seasons for Kenya, Somalia, Malawi, and Burkina Faso. In general, forecast skills improve substantially during the vegetative growth period (VP) and gradually during the reproductive development period (RP). Thus, mid-season assessment can provide effective early warning months before harvest. Skillful forecasts (Nash Sutcliffe Efficiency (NSE) > 0.6 and Mean Absolute Percentage Error (MAPE) < 20%) appear approximately two dekads after the VP; for example, skillful forecasts appear in May in Kenya and Somalia, January in Malawi, and July in Burkina Faso. During model development, effective EO features are also identified, such as precipitation and available water during VP, and dry days and extreme temperatures in early VP. Compared to monthly standard EO features, sub-monthly (dekadal), non-standard, and serial EO features significantly improve forecast skills by + 0.3 NSE and -10% of MAPE, demonstrating the ability to precisely and effectively capture favorable or detrimental crop development conditions. Finally, skillful forecasts and practical utility are demonstrated in the recent normal and dry years in each region. Overall, the developed yield forecasting system can provide skillful predictions during the growing season, supporting regional and international agricultural decision-making processes, including informing food-security planning and management, thereby helping to mitigate food shortages caused by unfavorable climate conditions.
更多查看译文
关键词
Earth observation,Machine learning,Food security,Maize yield,Operational forecast,Sub-Saharan Africa
AI 理解论文
溯源树
样例
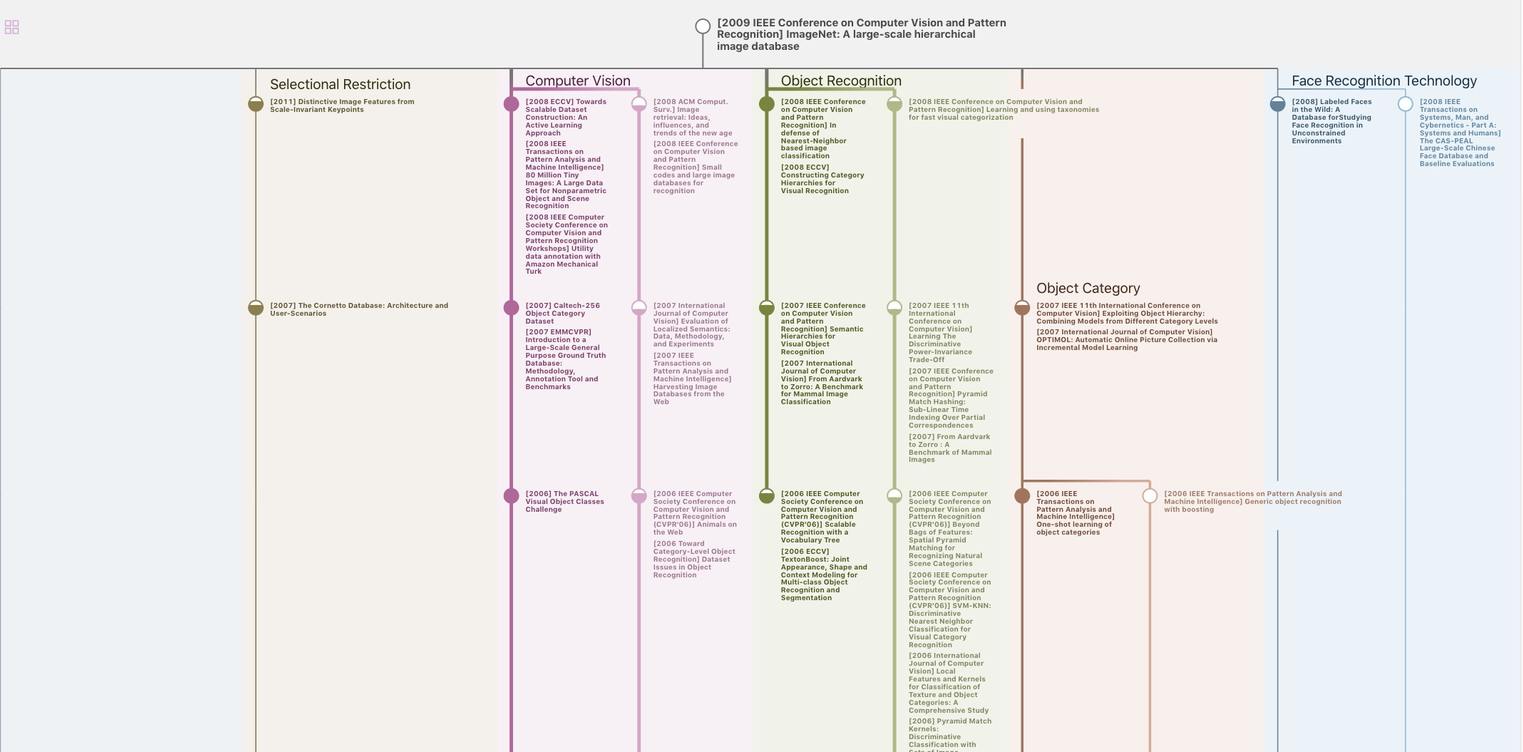
生成溯源树,研究论文发展脉络
Chat Paper
正在生成论文摘要