Embarrassingly Parallel Independent Training of Multi-Layer Perceptrons with Heterogeneous Architectures
arxiv(2022)
摘要
The definition of a Neural Network architecture is one of the most critical and challenging tasks to perform. In this paper, we propose ParallelMLPs. ParallelMLPs is a procedure to enable the training of several independent Multilayer Perceptron Neural Networks with a different number of neurons and activation functions in parallel by exploring the principle of locality and parallelization capabilities of modern CPUs and GPUs. The core idea of this technique is to use a Modified Matrix Multiplication that replaces an ordinal matrix multiplication by two simple matrix operations that allow separate and independent paths for gradient flowing, which can be used in other scenarios. We have assessed our algorithm in simulated datasets varying the number of samples, features and batches using 10,000 different models. We achieved a training speedup from 1 to 4 orders of magnitude if compared to the sequential approach.
更多查看译文
关键词
parallel independent training,multi-layer
AI 理解论文
溯源树
样例
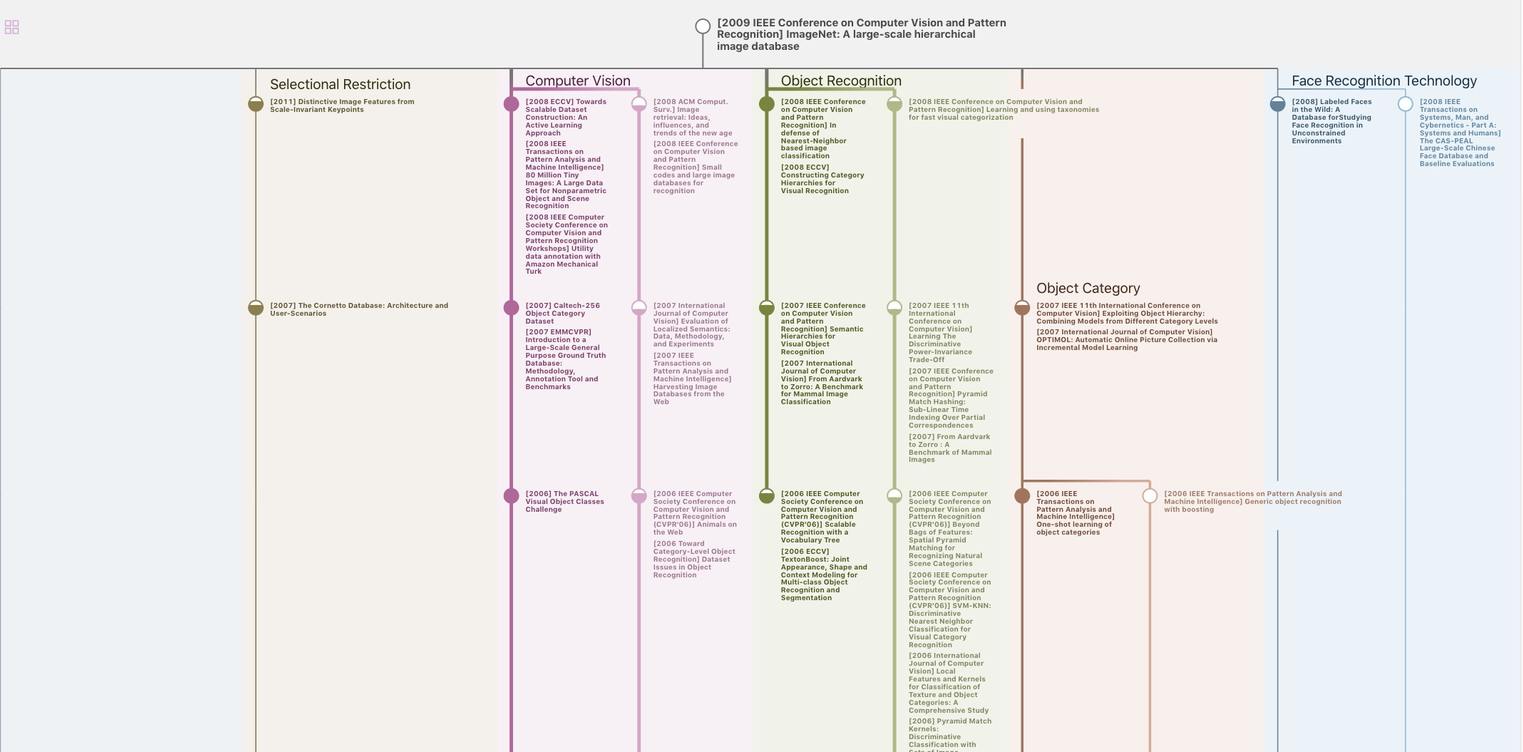
生成溯源树,研究论文发展脉络
Chat Paper
正在生成论文摘要