DeepFake Face Video Detection Using Hybrid Deep Residual Networks and LSTM Architecture
AI and Deep Learning in Biometric Security(2021)
摘要
Millions of images and videos are daily being shared on internet due to recent advances in social media and multimedia technology. However, many of them are manipulated using readily available image and video editing software and apps. Specifically, “DeepFake” videos, which are created by swapping an individual’s face with the face of other individual by utilising deep learning techniques, may constitute a substantial risk either to attack character of public figures or to fool automated face recognition systems. In this paper, a hybrid and high-confidence DeepFake face video detection framework is proposed to discriminate manipulated face videos from non-manipulated face videos. The proposed framework is composed of three main stages: face detection, deep feature extraction, and long short-term memory (LSTM) classification. The face detection is achieved by a well-known Viola–Jones detector. The detected face images are rescaled to make them compatible with an input of the pre-trained convolutional neural network model. The residual network with 50 layers is employed for deep feature extraction. Finally, the LSTM model is considered in classification to gather final verdict: bona fide or DeepFake. The considered LSTM network contains seven layers such as input layer, two biLSTM layers, dropout layer, fully connected layer, softmax layer, and classification layer. The proposed framework is capable of spotting DeepFake face videos with high accuracy that is substantiated via experimental analysis on two diverse and publicly available datasets (i.e., Celeb-DF and DeepFakeTIMIT).
更多查看译文
关键词
deepfake face video detection,hybrid deepfake residual networks,lstm architecture
AI 理解论文
溯源树
样例
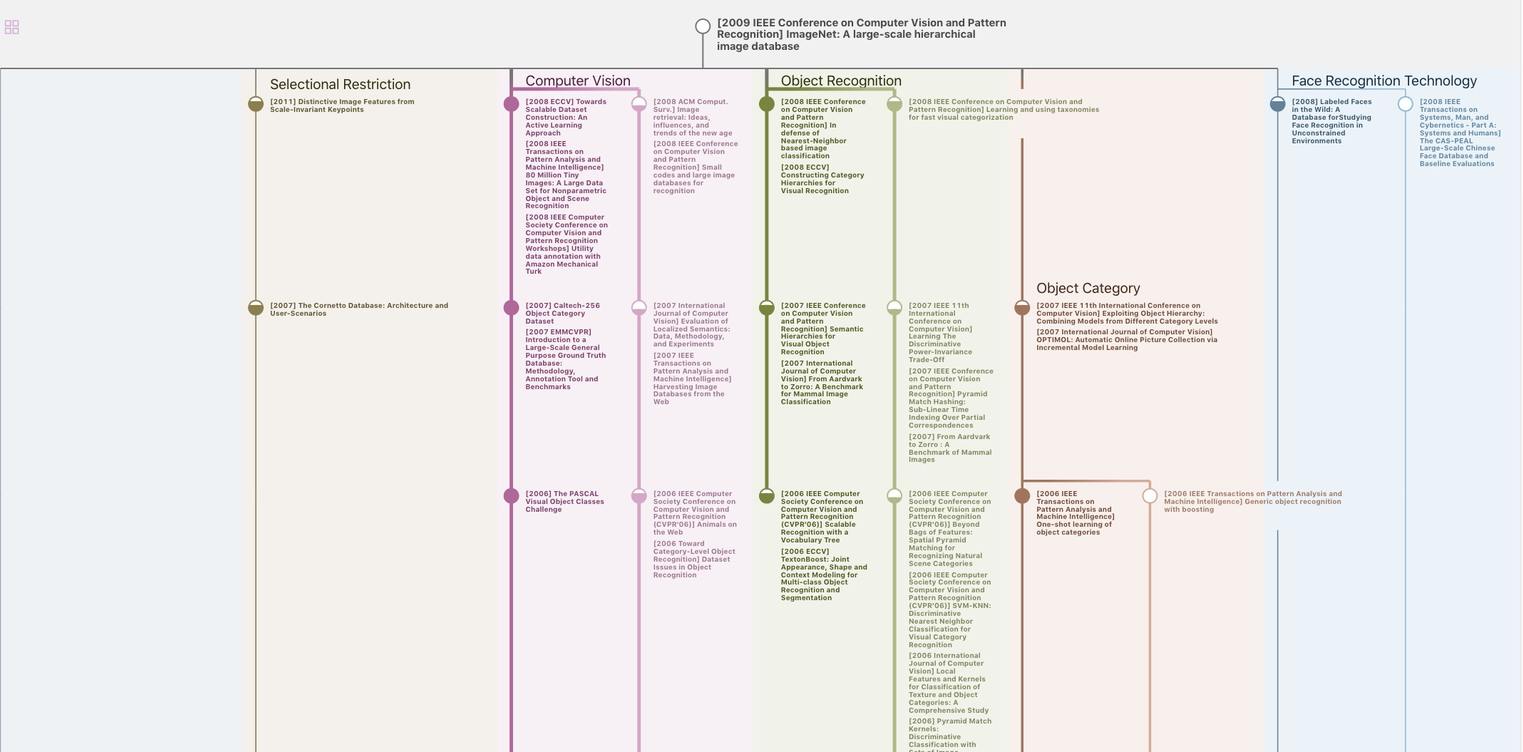
生成溯源树,研究论文发展脉络
Chat Paper
正在生成论文摘要