Linked Data Effectiveness in Neural Machine Translation
Proceedings of the 2020 4th International Symposium on Computer Science and Intelligent Control(2020)
摘要
Quality of data-driven Machine Translation (MT) systems depends on large volumes of data from which models can be constructed to leverage patterns and knowledge from these datasets. In corpus-based MT systems, Out-Of-Vocabulary (OOV) words and ambiguous translations are the most common sources of error. In this paper, JRC-Names and DBpedia have been employed as Linked Data (LD) to minimize the aforementioned types of errors on top of a Neural MT (NMT) model. Three strategies have been evaluated for exploiting knowledge from LD in translating named entities; 1) Dictionaries, 2) Pre-decoding, and 3) Post-editing. Based on the experimental results, these strategies optimize the benefit of the multilingual LD to NMT application. The experiments on English-Spanish translation as well as English-French translation evaluate the validity of the proposed idea.
更多查看译文
AI 理解论文
溯源树
样例
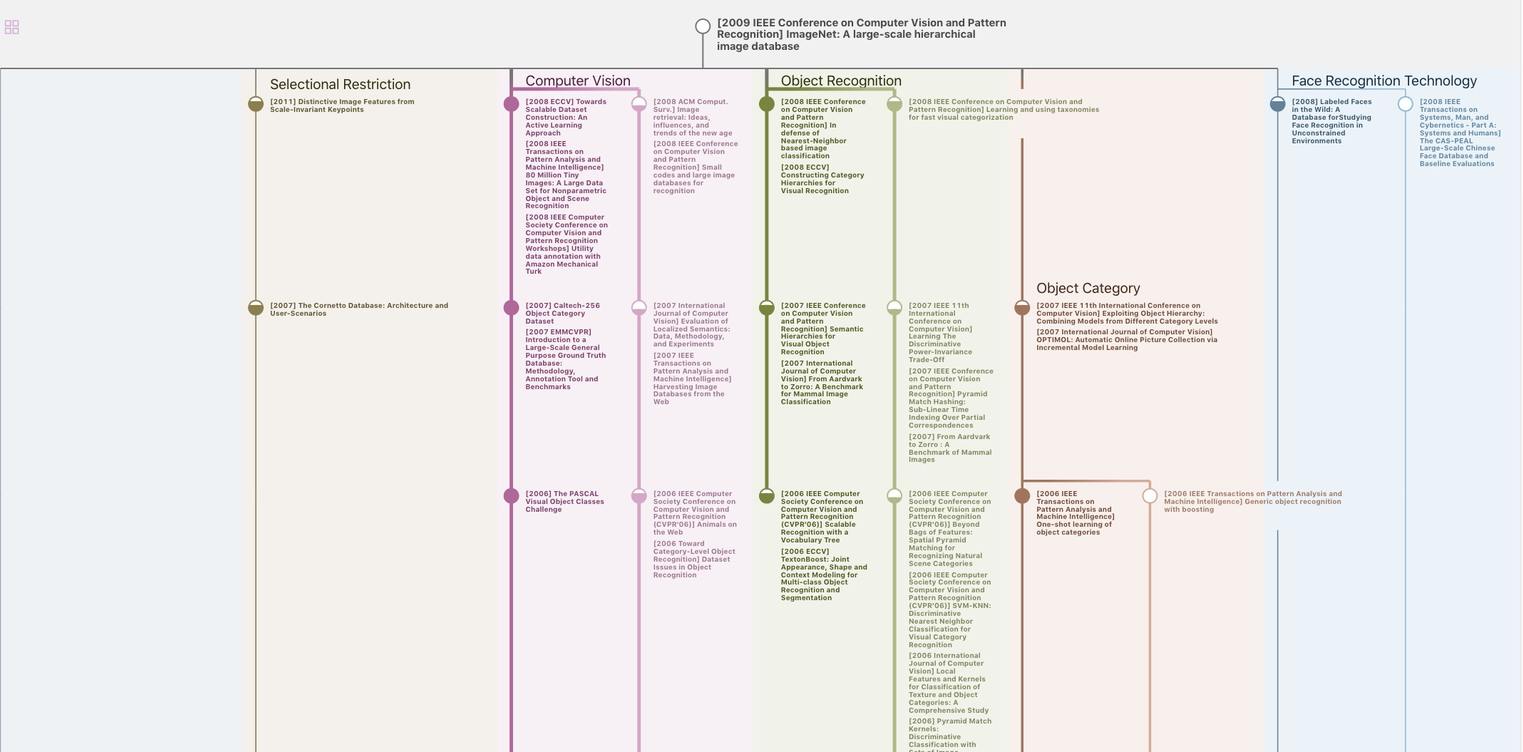
生成溯源树,研究论文发展脉络
Chat Paper
正在生成论文摘要