Identifying Top Predictors of Change in Noncalcified Coronary Burden in Psoriasis by Machine Learning Over 1-Year
Journal of Psoriasis and Psoriatic Arthritis(2021)
摘要
Background: Psoriasis is associated with accelerated non-calcified coronary burden (NCB) by coronary computed tomography angiography (CCTA). Machine learning (ML) algorithms have been shown to effectively identify cardiometabolic variables with NCB in cross-sectional analysis. Objective: To use ML methods to characterize important predictors of change in NCB by CCTA in psoriasis over 1-year of observation. Methods: The analysis included 182 consecutive patients with 80 available variables from the Psoriasis Atherosclerosis Cardiometabolic Initiative, a prospective, observational cohort study at baseline and 1-year using the random forest regression algorithm. NCB was assessed at baseline and 1-year from CCTA. Results: Using ML, we identified variables of high importance in the context of predicting changes in NCB. For the cohort that improved NCB (n = 102), top baseline variables were cholesterol (total and HDL), white blood cell count, psoriasis area severity index score, and diastolic blood pressure. Top predictors of 1-year change were change in visceral adiposity, white blood cell count, total cholesterol, c-reactive protein, and absolute lymphocyte count. For the cohort that worsened NCB (n = 80), the top baseline variables were HDL cholesterol related including apolipoprotein A1, basophil count, and psoriasis area severity index score, and top predictors of 1-year change were change in apoA, apoB, and systolic blood pressure. Conclusion: ML methods ranked predictors of progression and regression of NCB in psoriasis over 1 year providing strong evidence to focus on treating LDL, blood pressure, and obesity; as well as the importance of controlling cutaneous disease in psoriasis.
更多查看译文
AI 理解论文
溯源树
样例
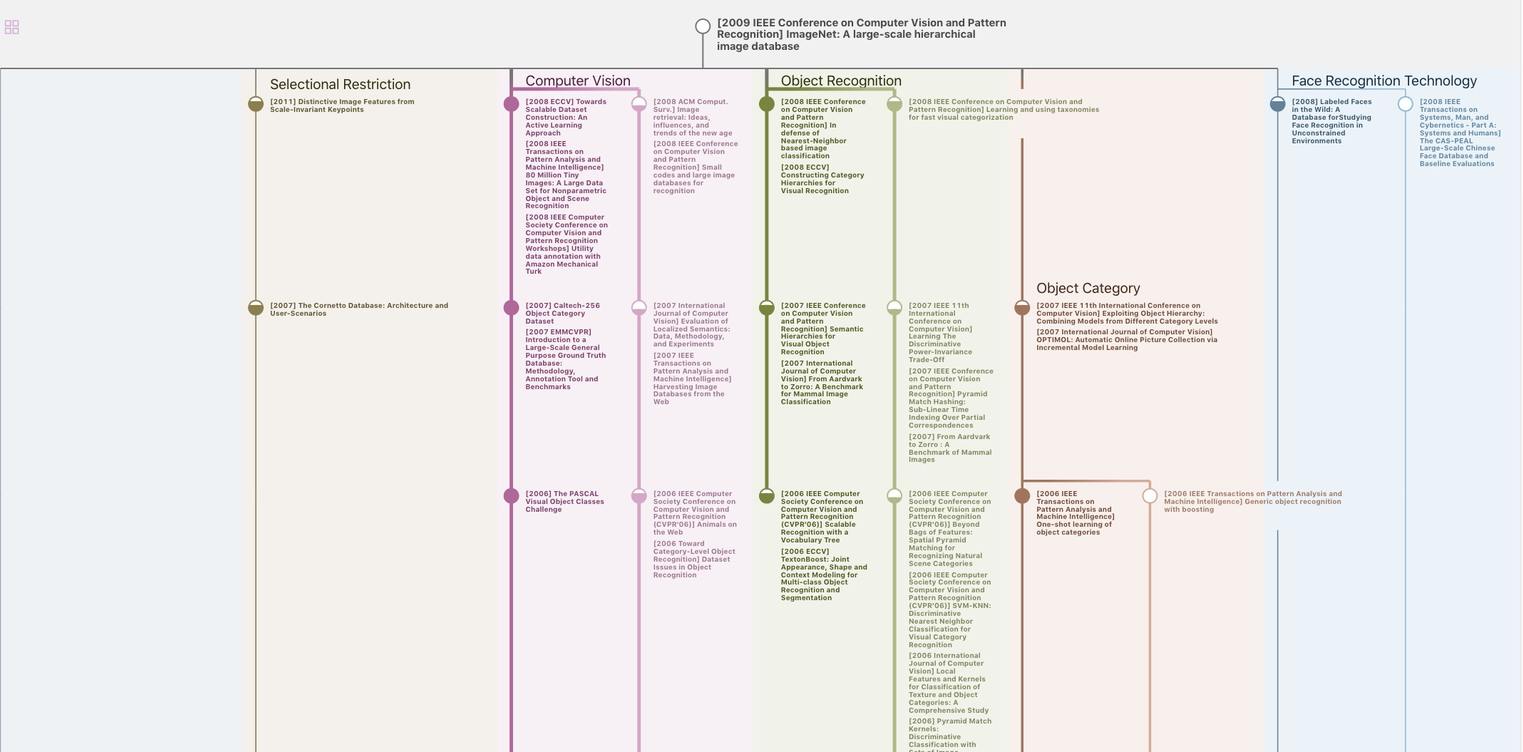
生成溯源树,研究论文发展脉络
Chat Paper
正在生成论文摘要