Multi-Agent Recommender System
Recent Advances in Agent-based NegotiationStudies in Computational Intelligence(2021)
摘要
A recommender agent (RA) provides users with recommendations about products/services. Recommendations are made on the basis of information available about the products/services and the users, and this process typically involves making predictions about user preferences and matching them with product attributes. Machine learning methods are being studied extensively to design RAs. In this approach, a model is learnt from historical data about trading (i.e. data about products and the users buying them). There are numerous different learning methods, and how accurately a method can make a recommendation depends on the adopted method and also on the use of historical data. Given this, we propose a multi-agent recommender system called MARS which combines various different machine learning methods. Within MARS, different agents are designed to make recommendations using different machine learning methods. Since different agents use different machine learning methods, the recommendations they make may be conflicting. Negotiation is used to come to an agreement on a recommendation. Negotiation is conducted using a contract-net protocol. The performance of MARS is evaluated in terms of recommendation error. The results of simulations show that MARS outperforms five existing recommender systems.
更多查看译文
关键词
multi-agent
AI 理解论文
溯源树
样例
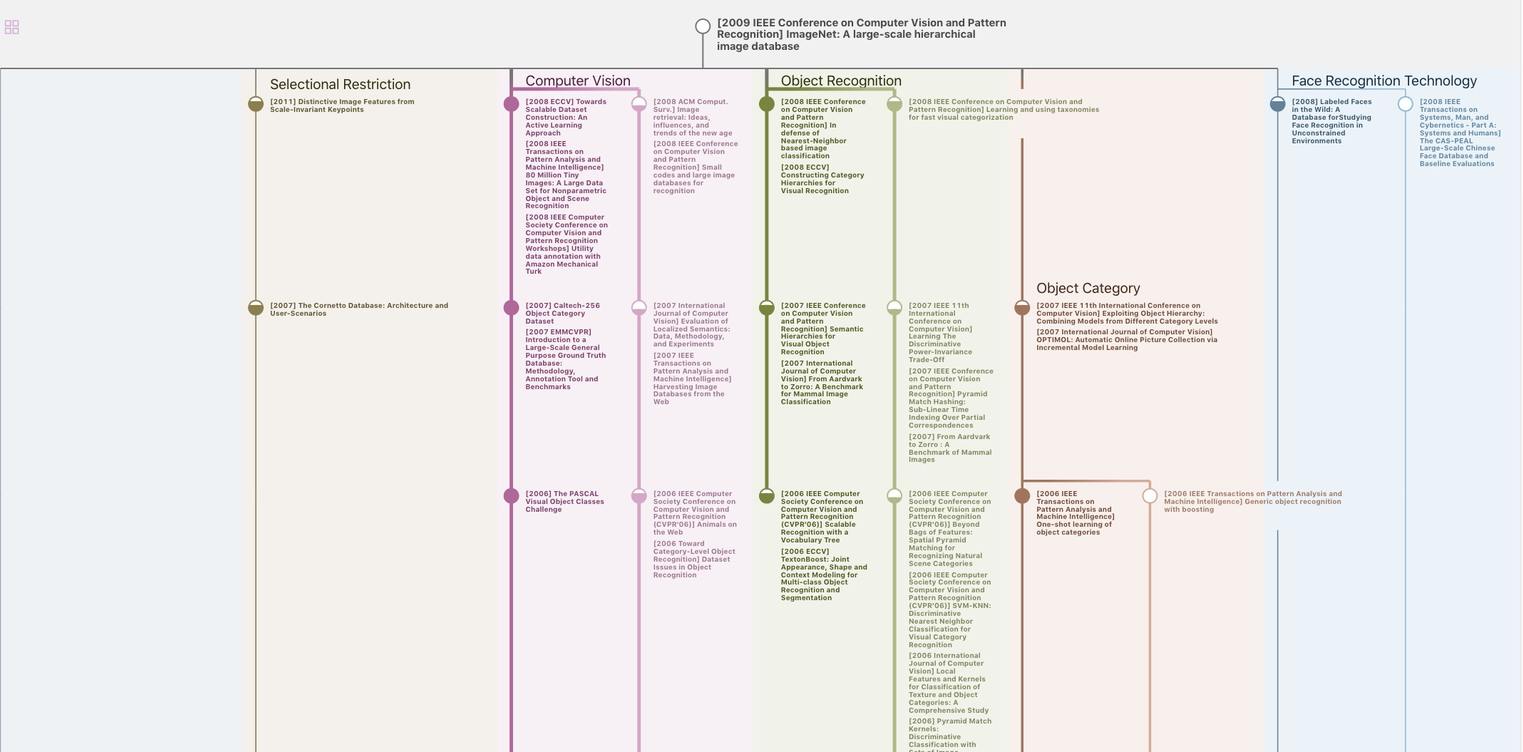
生成溯源树,研究论文发展脉络
Chat Paper
正在生成论文摘要