Human activity recognition using penalized support vector machines and Hidden Markov Models in multimodal systems
Revista Facultad de Ingeniería Universidad de Antioquia(2021)
摘要
Human activity detection has evolved due to the advances and developments of machine learning techniques, which have enabled solutions to new challenges without ignoring prevalent difficulties that need to be addressed. One of the challenges is the learning model’s sensitivity regarding the unbalanced, atypical, and overlapping information that directly affects the performance of the model. This article evaluates a methodology for the classification of human activities that penalizes defective information. The methodology is carried out through two redundant classifiers, a penalized support vector machine that detects the sub-movements (micro-movements) and the Marvok Hidden Model that predicts the activity given the micro- movements sequence. The performance of the method was compared with state-of-the-art techniques, and the findings suggested significative advance in the detection of micro-movements compared to the data obtained with non-penalized paradigms. In this research, an adequate performance is found in the classification of primitive movements, with hit rates of 95.15% for the Kinect One®, 96.86% for the IMU sensor network, and 67.51% for the EMG sensor network.
更多查看译文
关键词
multisensor,data fusion,primitive motion,machine learning,overlapping databases
AI 理解论文
溯源树
样例
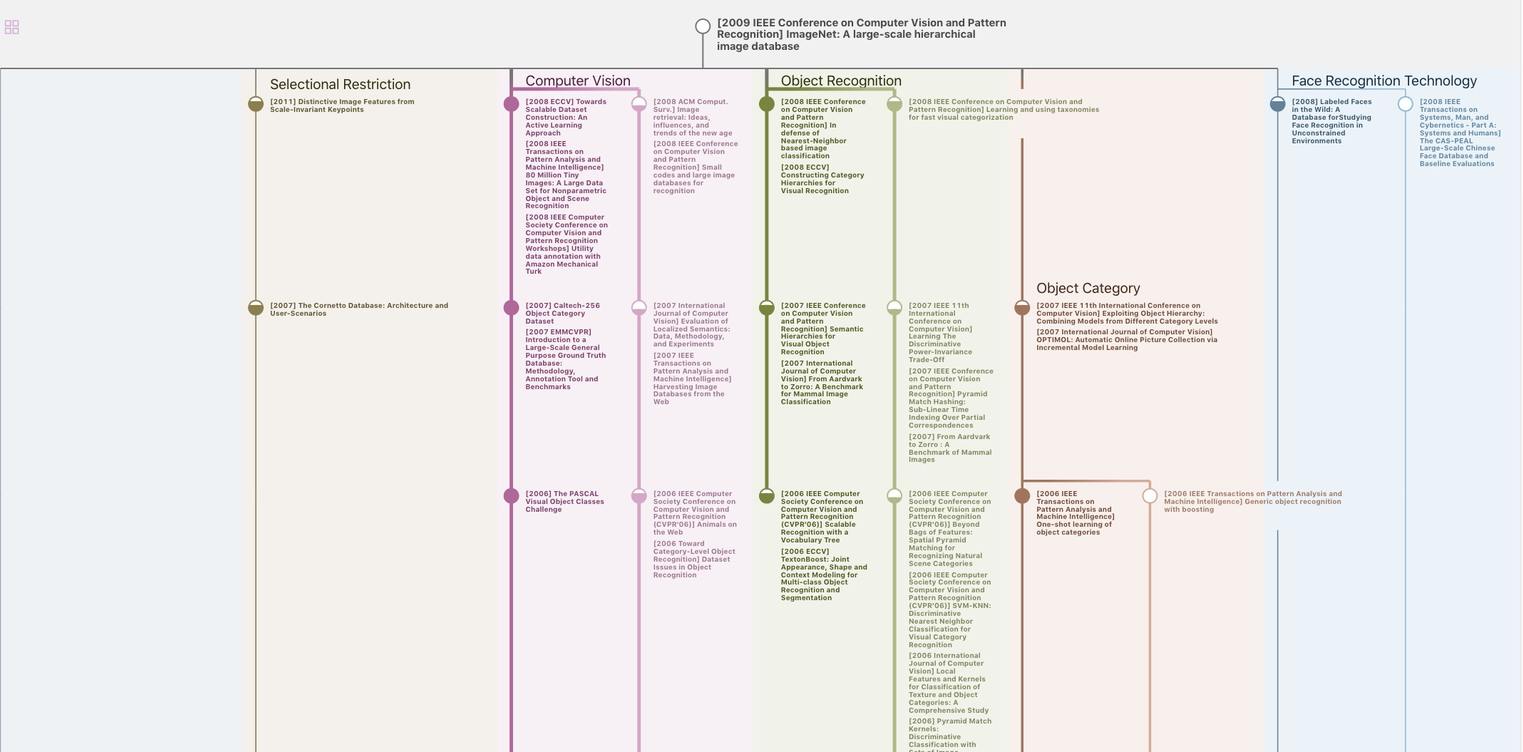
生成溯源树,研究论文发展脉络
Chat Paper
正在生成论文摘要