Models for Multiplicative Noise Removal
Handbook of Mathematical Models and Algorithms in Computer Vision and Imaging(2022)
摘要
Image denoising is the most important step in image processing for further image analysis. It is an important topic in many applications, such as object recognition, digital entertainment, etc. The digital image can be corrupted with noise during acquisition, storage, and transmission. Noise can be classified as additive noise, multiplicative noise, and non-additive non-multiplicative noise (such as salt and pepper noise, Poisson noise). The main properties of a good image denoising model are that it will remove noise while preserving details of the image. This chapter aims to present a review of multiplicative denoising models, especially for the multiplicative Gamma noise. Similar to denoising for additive Gaussian noise, these denoising approaches can be categorized as variational methods, non-local methods, and deep neural network-based methods. Due to space constraints, this chapter only discusses some of them. The rest of this chapter is organized as follows. Section "Introduction" is an introduction and section "Variational Methods with Different Data Fidelity Terms" describes variational methods with different data fidelity terms. Section "Variational Methods with Different Regularizers" introduces variational methods with different regularizers. Sections "Multitasks" to "DNN Method" describe multitasks, nonlocal, and deep neural network (DNN) methods. Finally, section "Conclusion" presents our conclusions.
更多查看译文
关键词
Multiplicative denoising,Variational methods,Nonlocal methods,Multitasks,DNN methods
AI 理解论文
溯源树
样例
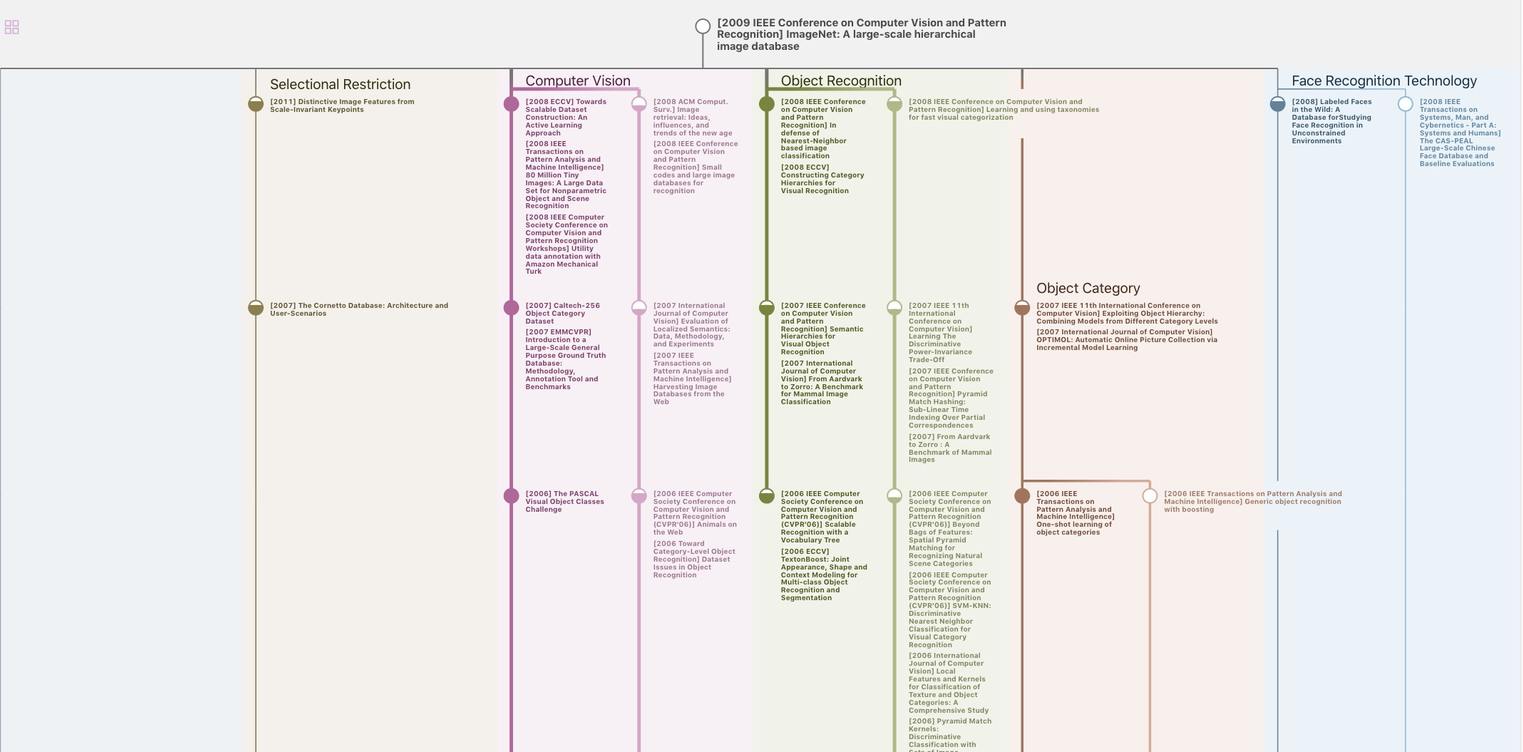
生成溯源树,研究论文发展脉络
Chat Paper
正在生成论文摘要