Improved deep reinforcement learning based convergence adjustment method for power flow calculation
The 16th IET International Conference on AC and DC Power Transmission (ACDC 2020)(2021)
摘要
The Power Flow Convergence (PFC) adjustment is an essential issue in the study of power system Operation State Calculation (OSC). Currently, as the grid structure expands markedly, the PFC adjustment is realized by adjusting the regional active and reactive power manually in most dispatching centres, which is tedious and personnel-experience oriented. Therefore, it is crucial to automate the PFC adjustment for efficiency and quality improvement. In this paper, an improved deep reinforcement learning (IDRL) based method and an action-executing strategy are proposed for the automation of the PFC adjustment. The optimal configuration scheme of the adjustable generators is derived from the trained online Q network for multi-load levels. Finally, the testing results on the IEEE-118 bus system demonstrate that the proposed method can obtain the convergent power flow state according to the given load level effectively.
更多查看译文
关键词
power flow calculation,Power Flow Convergence adjustment,power system Operation State Calculation,PFC adjustment,regional active power,reactive power,improved deep reinforcement learning based method,convergent power flow state,IDRL,action-executing strategy,IEEE-118 bus system,online Q network
AI 理解论文
溯源树
样例
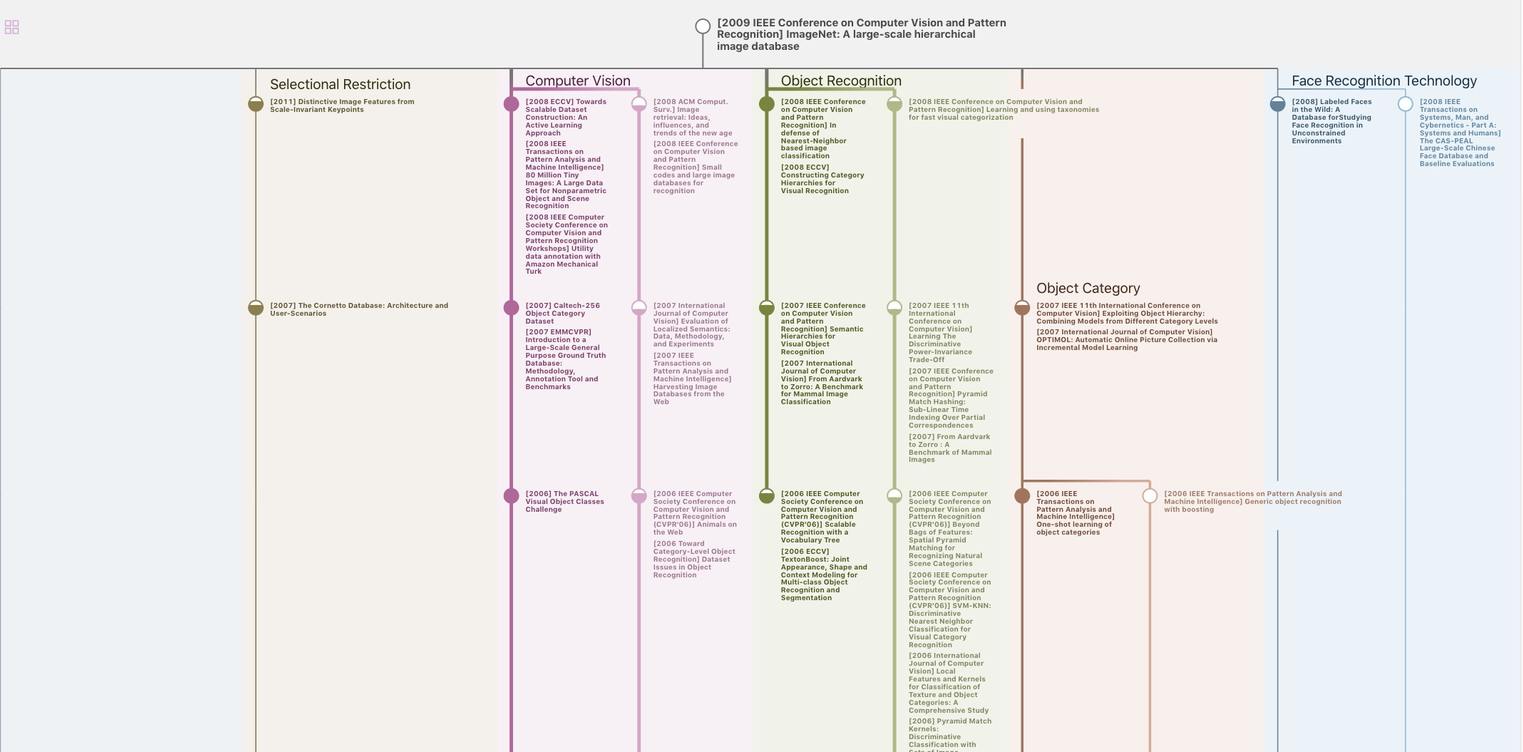
生成溯源树,研究论文发展脉络
Chat Paper
正在生成论文摘要