Study on Hysteresis of Combustion Oscillation in Continuously Variable Resonance Combustor using Deep Neural Network
TRANSACTIONS OF THE JAPAN SOCIETY FOR AERONAUTICAL AND SPACE SCIENCES, AEROSPACE TECHNOLOGY JAPAN(2021)
摘要
Deep auto-encoder (DAE) is applied to the decomposition analysis on the transition process of intrinsic combustion oscillation. Two dimensional URANS simulation reproduced combustion oscillation in CVRC (continuously variable resonance combustor) including the dynamics of the stable-to-unstable and unstable-to-stable transition. The transition process has hysteresis while decreasing/increasing its oxidizer post length to change the stability. The time evolution of temperature, pressure, and heat release distributions are sampled during the hysteresis process. The DAE consists of an encoder network that outputs two feature variables and a decoder network that outputs the approximation of the input data set. The sampled time series of the distribution data are put into the DAE to train. The two variables characterize the state of the oscillation in a 2D feature space. The weight vector of the neurons in the last layer are trained so that the weighted sum of them approximately compose the simulated fields; and thus, the fields are decomposed into modes. By tracing the trajectory of the transition on the feature space during the hysteresis, it is found that the space successfully classifies the level of combustion instability. The decomposed modes are useful to identify the key phenomena of hysteresis process.
更多查看译文
关键词
continuously variable resonance combustor,combustion oscillation,neural network,hysteresis
AI 理解论文
溯源树
样例
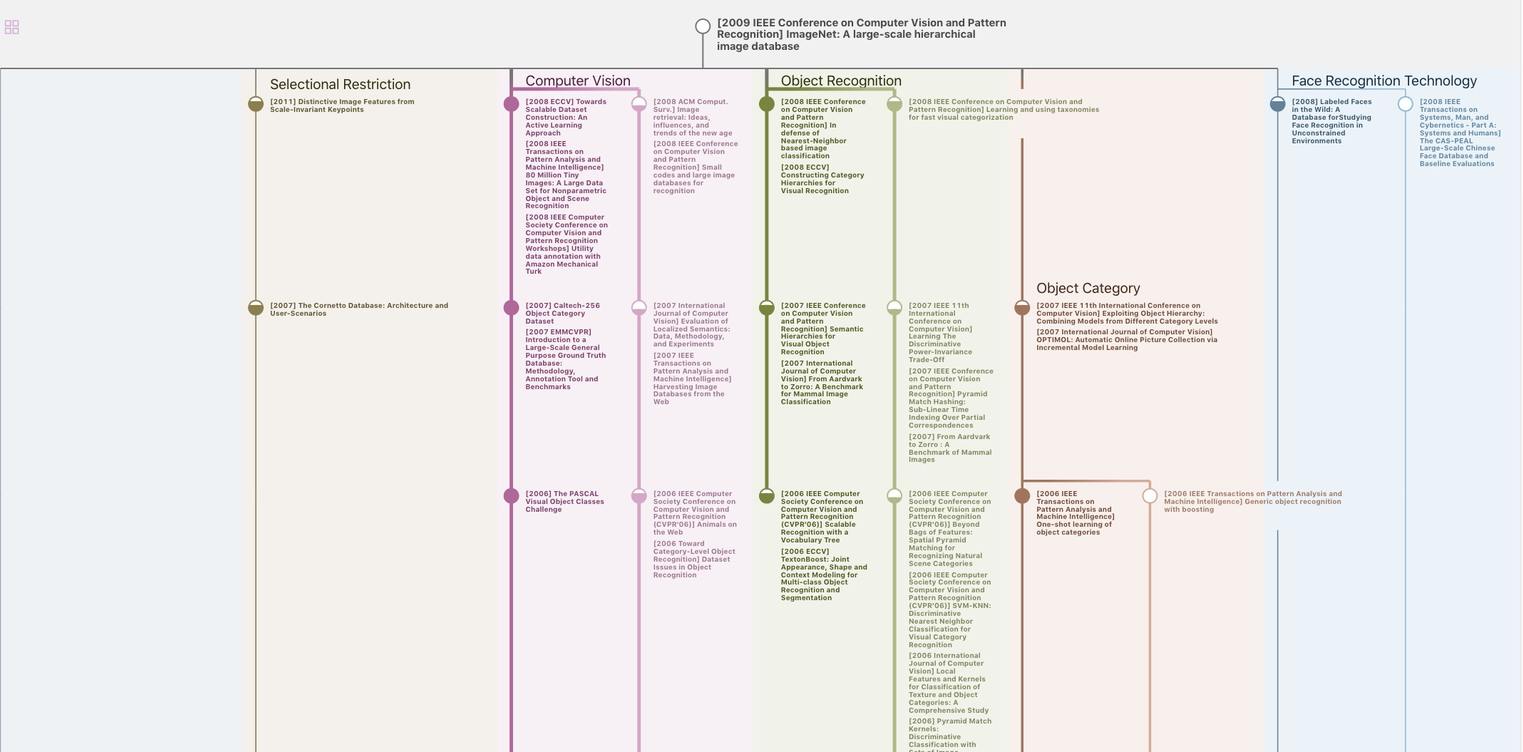
生成溯源树,研究论文发展脉络
Chat Paper
正在生成论文摘要