Automated myocardial segmentation in native t1-mapping cardiovascular magnetic resonance images based on machine learning: a validation study in the UK biobank"s covid-19 subset
European Heart Journal - Cardiovascular Imaging(2021)
摘要
Abstract Funding Acknowledgements Type of funding sources: Public grant(s) – National budget only. Main funding source(s): Innovate UK Background Regional assessment of septal native T1 values with cardiovascular magnetic resonance (CMR) is used to characterise diffuse myocardial diseases. Previous studies suggest its potential role in detecting early pathological alterations, which may help identify high-risk subjects at early disease stages. Automated analysis of myocardial native T1 images may enable faster CMR analysis and reduce inter-observer variability of manual analysis. However, the technical performance of such methodologies has not been previously reported. Purpose We tested, in a subset of UK Biobank participants, the degree of agreement between CMR septal myocardial T1 values obtained from our machine learning (ML) algorithm and septal native T1 values computed from manual segmentations. Methods We analysed the first 292 participants who were tested for severe acute respiratory syndrome coronavirus 2 (SARS-CoV-2) and had CMR imaging (1.5 Tesla, Siemens MAGNETOM Aera). T1 mapping was performed in a single mid-ventricular short axis (SAX) slice using ShMOLLI (WIP780B) sequences. Three experienced CMR readers independently measured native T1 values by manually placing a single region of interest (ROI) covering half of the anteroseptal and half of the inferoseptal wall using cvi42 post-processing software (version 5.11). A mean T1 value for each participant was then calculated. A ML algorithm developed by Circle Cardiovascular Imaging Inc. was then applied to the same images to derive the myocardium T1 values automatically. The algorithm was previously trained to segment myocardium from SAX T1 and non-T1 mapping images on two external CMR datasets. We compared the mean septal ROI T1 values to the mean myocardium T1 values predicted by the ML algorithm. Results Two studies were excluded after quality control. The ML-derived and the manually calculated mean T1 values were significantly correlated (r = 0.82, p < 0.001). The Bland-Altman analysis between the two methods showed a mean bias of 3.64 ms, with 95% limits of agreement of −38.88 to 53.46 ms, indicating good agreement (figure 1). Conclusions We demonstrated strong correlation and good agreement between native T1 values obtained from our automated analysis method and manual T1 septal analysis in a subset of UK Biobank participants. This algorithm may represent a valuable tool for clinicians allowing for fast and potentially less operator-dependent myocardial tissue characterisation. However, validation of more extensive datasets and quality control processes are needed.
更多查看译文
AI 理解论文
溯源树
样例
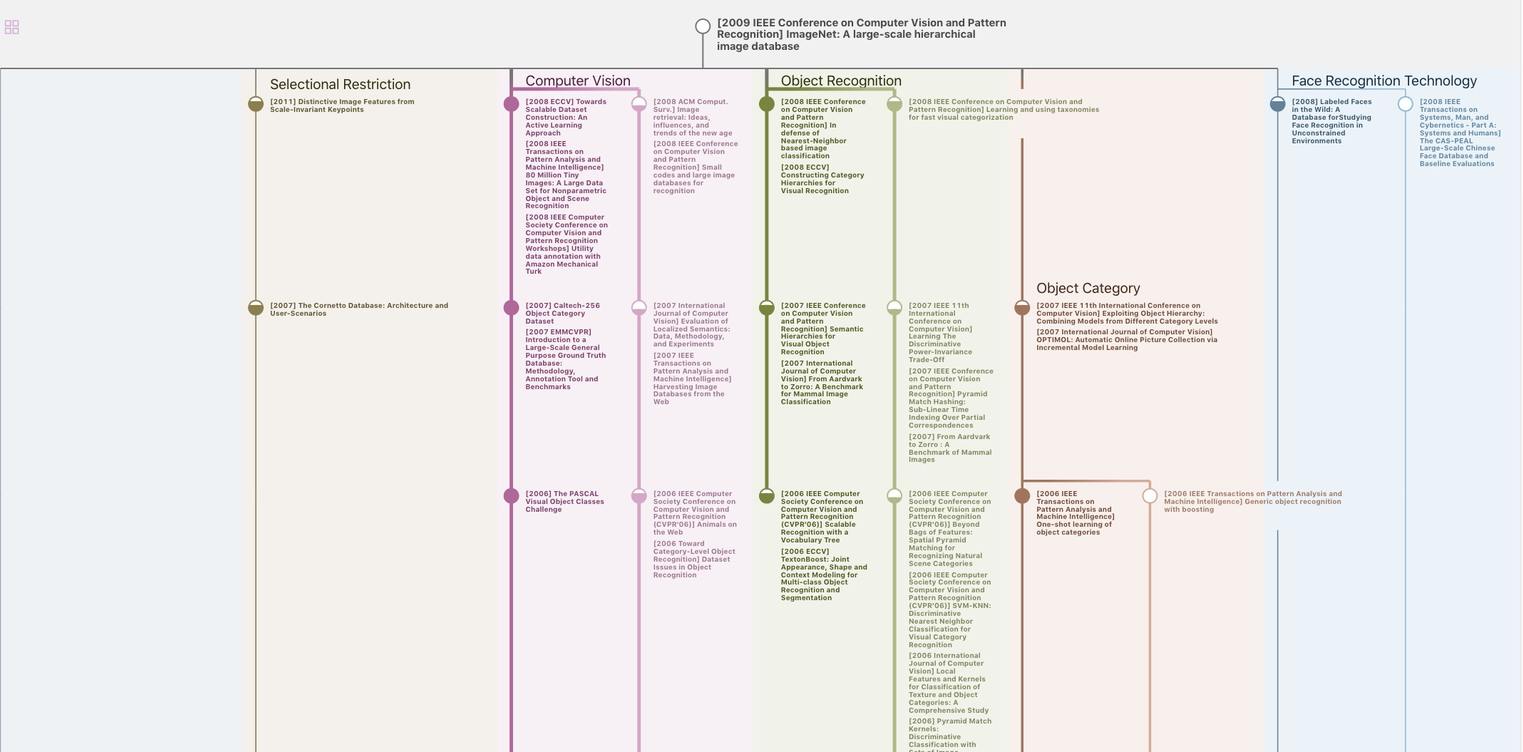
生成溯源树,研究论文发展脉络
Chat Paper
正在生成论文摘要