Estimation of Grouped Parameters Using Tanks-in-Series Lithium-Ion Battery Model
ECS Meeting Abstracts(2021)
摘要
Parameter estimation is critical for efficient Battery Management System (BMS) performance. The accuracy and predictability of a model depends on the precision of its parameters. Moreover, model parameters tend to change with battery aging. Efficient BMS performance requires accurate tracking and updating of relevant parameters as the battery ages. Estimating parameters for electrochemical models is challenging due to the complexity of the governing equations, and the possibility of degeneracy, with multiple sets of parameter values that give the same accuracy for fitting charge/discharge data. Parameter estimation of various lithium-ion battery systems has been attempted with different model types, including equivalent circuit model2, single particle model3 and pseudo 2D (P2D) model.4,5 Estimation approaches reported in literature, such as, GA6, heuristic algorithms7, etc., are difficult to implement in real-time due to computational complexity. In the past, we proposed and implemented reformulated p2D models for real-time parameter estimation.8,9 While the reformulated models are computationally inexpensive, the large set of parameters and resulting likelihood of degeneracy introduce substantial complexity. Recently, we proposed lithium-ion Tanks-in-Series battery model10. This model is generated by systematic volume-averaging of the p2D model that enables efficient and real-time simulation with reduced set of parameters. In this presentation, we propose to estimate non-dimensional grouped parameters using non-dimensional Tanks-in-Series battery model. The grouping of parameters further reduces the number of parameters that needs to be estimated. This also reduces the computational effort required to perform optimization. The non-dimensional model captures resistances offered due to transport, reaction kinetics, and ohmic drop in electrolyte based on the non-dimensional grouped parameters that absorb constitutive expressions and parameters, such as electrolyte diffusivity, ionic conductivity, reaction rate constants and solid phase diffusivity. The estimation is performed on experimental charge/discharge data obtained from cylindrical cells having NCA and NMC cathode chemistries. The accuracy of the estimated parameters is validated using experimental cycling data obtained at different c-rates by calculating the error in voltage-time curves between the model prediction using estimated parameters and the experimental data. Acknowledgements The authors acknowledge funding from the U.S. Department of Energy Office of Electricity Energy Storage Program through Sandia National Laboratories, under the guidance of Dr. Imre Gyuk, and the Texas Materials Institute at The University of Texas at Austin. Sandia National Laboratories is a multi-mission laboratory managed and operated by National Technology & Engineering Solutions of Sandia, LLC, a wholly owned subsidiary of Honeywell International, Inc., for the U.S. Department of Energy National Nuclear Security Administration under contract DE-NA-0003525. SAND2020-14131 A References V. Ramadesigan, P. W. C. Northrop, S. De, S. Santhanagopalan, R. D. Braatz, and V. R. Subramanian, J. Electrochem. Soc., 159, R31–R45 (2012). Y. Hu, S. Yurkovich, Y. Guezennec, and B. J. Yurkovich, Control Eng. Pract., 17, 1190–1201 (2009). A. P. Schmidt, M. Bitzer, Á. W. Imre, and L. Guzzella, IFAC Proc. Vol., 43, 198–203 (2010). J. C. Forman, S. J. Moura, J. L. Stein, and H. K. Fathy, in 5th Annual Dynamic Systems and Control Conference,, p. 1–8 (2012). S. Santhanagopalan, Q. Guo, and R. E. White, J. Electrochem. Soc., 154, 198–206 (2007). A. Jokar, B. Rajabloo, M. Désilets, and M. Lacroix, J. Electrochem. Soc., 163, A2876–A2886 (2016). J. Li, L. Zou, F. Tian, X. Dong, Z. Zou, and H. Yang, J. Electrochem. Soc., 163, A1646–A1652 (2016). V. Boovaragavan, S. Harinipriya, and V. R. Subramanian, J. Power Sources, 183, 361–365 (2008). V. Ramadesigan, K. Chen, N. A. Burns, V. Boovaragavan, R. D. Braatz, and V. R. Subramanian, J. Electrochem. Soc., 158, A1048–A1054 (2011). A. Subramaniam, S. Kolluri, C. D. Parke, M. Pathak, S. Santhanagopalan, and V. R. Subramanian, J. Electrochem. Soc., 167, 013534-013534–18 (2020).
更多查看译文
AI 理解论文
溯源树
样例
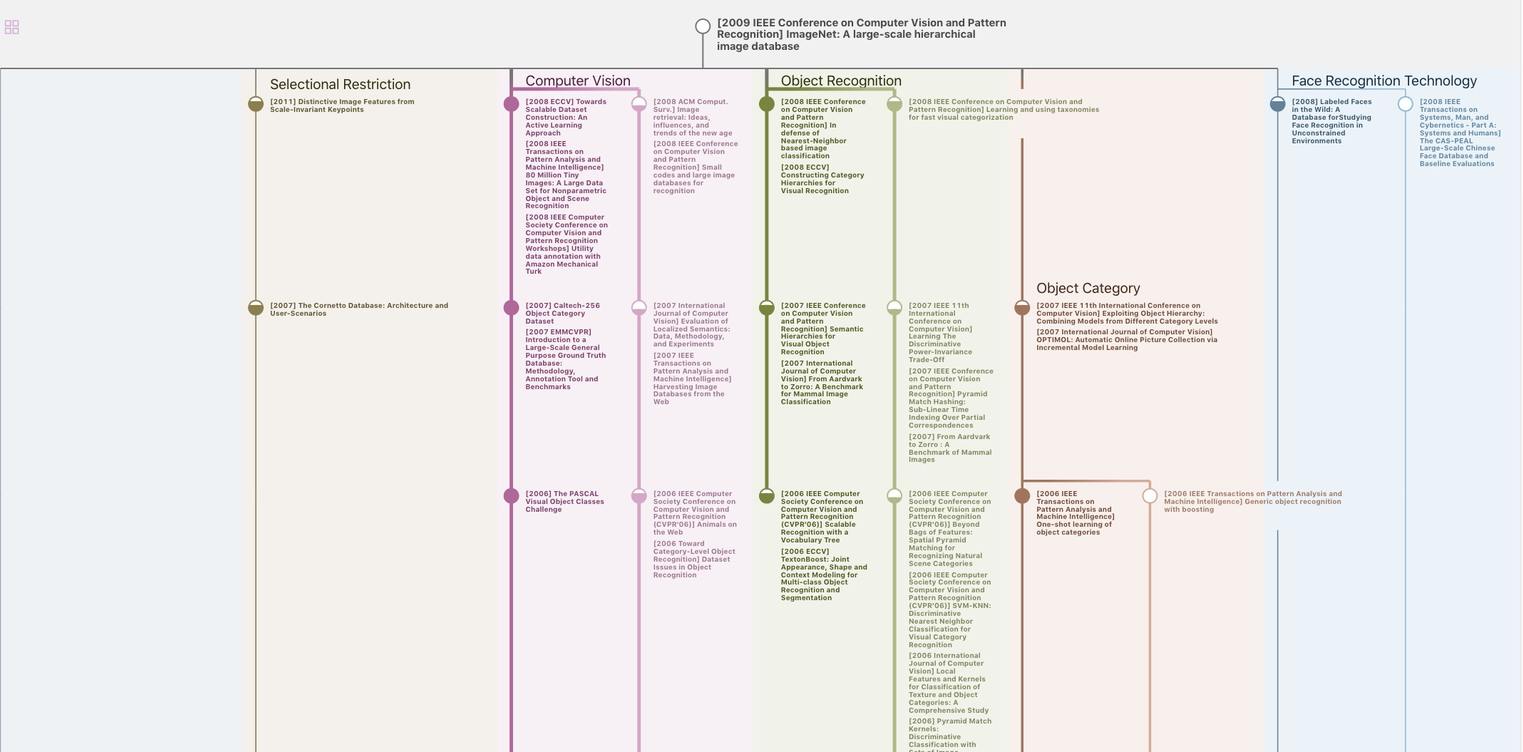
生成溯源树,研究论文发展脉络
Chat Paper
正在生成论文摘要