Quantification of Multi-Ion Mixtures Using a Machine Learning Assisted Integrated Electronic Tongue Leveraging Mobile and Cloud Platforms
ECS Meeting Abstracts(2021)
摘要
Potentiometric electronic tongues have been proposed as versatile sensor arrays for chemical analysis of a wide variety of analytes without the need for high specificity of individual sensors[i]. The prospect of portable electronic tongues offers new approaches for distributed, decentralized chemical analysis for various applications such as traceability of goods in supply chains[ii] , [iii]. A challenge for electronic tongues, however, has often been the quantitative determination of concentrations of target analytes in the presence of multiple interferents due to the inherent cross-sensitivity of the sensors employed. In the present contribution, we extend our previously reported work[iv] on an all-solid-state electronic tongue based on an array of electrodeposited polymeric sensors to study the feasibility and accuracy of employing this class of sensors to the quantification of multiple analytes. The sensor array studied herein is entirely integrated on a conventional printed circuit board (PCB). We demonstrate how a remarkably simple sensor configuration can be calibrated by means of a prescriptive training scheme and tree-based machine learning algorithms to provide a quantitative assessment of the concentrations of multiple metal cations concomitantly in a single measurement lasting less than 2 minutes. In contrast to conventional electrochemical sensors, no reference electrode was employed. Interestingly, a relative accuracy better than 7% was found for the determination of concentrations of various ions (Na, Mn, Fe, Al, Cu, Pb) in pure solutions at concentrations ranging between 2 mg/L and 100 mg/L. The potential applicability of this chemical testing framework to decentralized analysis was showcased by deploying a trained machine learning regression model as a cloud service that was invoked from a mobile phone serving as gateway for the portable potentiometric sensor array. Thus, the direct quantification of multiple cations in aqueous mixtures was realized by applying an automated machine learning pipeline including data pre-processing and feature extraction to the transient potentiometric data obtained from the miniaturized array of polymeric sensors integrated on a PCB. The proof-of-concept system employed 16 polymeric sensors that were electrodeposited on conventional electroless nickel immersion gold (ENIG) metallization. The conductive polymers were electrodeposited by chronoamperometry or cyclic voltammetry and tailored for enhanced cationic sensitivity. Characteristic features were extracted from the transient differential voltages between these polymeric sensors during the transition of the sensor array from a reference solution to a test solution, thereby obviating the need for a conventional reference electrode. The analysis of the differential voltages revealed deviations from a linear sensitivity, showing super-Nernstian responses and non-linear voltage trends when varying the concentration of the cations mentioned above. Extraction of cross-sensitivity parameters, such as average sensitivity slope and non-selectivity factor, demonstrate how the potentiometric response of the electronic tongue based entirely on non-selective sensors differs from that of an array of exclusively selective sensors (e.g. ion-selective electrodes, ISEs) presented in previous works. Training data for the tree-based machine learning algorithm was obtained by alternatively immersing the sensor array in reference and test solutions continuously using an automated test rig. In addition to the conventional approach of measuring the equilibrium potential after a certain settling time, we find that the analysis of the complete evolution of the potentiometric signal in time provides additional information that is useful for training of the regression models. Dimension reduction of time series data was achieved through various techniques, such as down-sampling, feature transformation (e.g. PCA) and feature extraction. Here, five characteristics from each potentiometric transient were used to describe the complete voltage perturbation of the sensor during transition between reference and test solution. Discrimination of multiple ions and sensitivity to variation in their concentrations was demonstrated by testing 25 nitrate-based mixtures of six ions (Al, Cu, Na, Mn, Fe, Pb) in the range 0.5-10 mg/L following an Orthogonal Experimental Design (OED). Extra Trees-based regression models showed significantly higher quantification accuracy compared to widely used multivariate regression models such as Multiple Linear Regression (MLR). Concentrations of the metal cations in pure solutions were determined in less than 2 minutes at an average mean relative error of 1-7% in the concentration range 2-100 mg/L. In a model mixture comprising Al, Cu, Na and Fe, the mean relative error was found to depend on the type of ion, and varied between 1% for Fe and 44% for Na in the concentration range 1-10 mg/L. Regression models trained using the combination of the five extracted features showed higher predictive capabilities compared to single-features based algorithms, thereby underscoring the importance of feature selection for data-driven sensors. Finally, we demonstrate the functionality of the proposed portable device by quantifying concentrations of multi-ion mixtures using an integrated device comprising the sensor array, microcontroller-based data acquisition, wireless data transmission, a mobile app and cloud service providing the trained regression model. [i] Vlasov, Y.; Legin, A.; Rudnitskaya, A.; Di Natale, C.; D'Amico, A. Nonspecific sensor arrays ("electronic tongue") for chemical analysis of liquids: (IUPAC technical report), Pure Appl. Chem., 2005, 77, 1965-1983 [ii] Legin A.; Rudnitskaya, A.; Vlasov, Y. Electronic tongues: New analytical perspective for chemical sensors, Comp. Anal. Chem., 2003, 39, 437-486 [iii] Kirsanov, D.; Correa, D.S.; Gaal, G.; Riul, A. Electronic Tongues for Inedible Media., Sensors 2019, 19, 5113 [iv] P. W. Ruch et al., "A portable potentiometric electronic tongue leveraging smartphone and cloud platforms," 2019 IEEE International Symposium on Olfaction and Electronic Nose (ISOEN), Fukuoka, Japan, 2019. doi: 10.1109/ISOEN.2019.8823244 Figure 1
更多查看译文
AI 理解论文
溯源树
样例
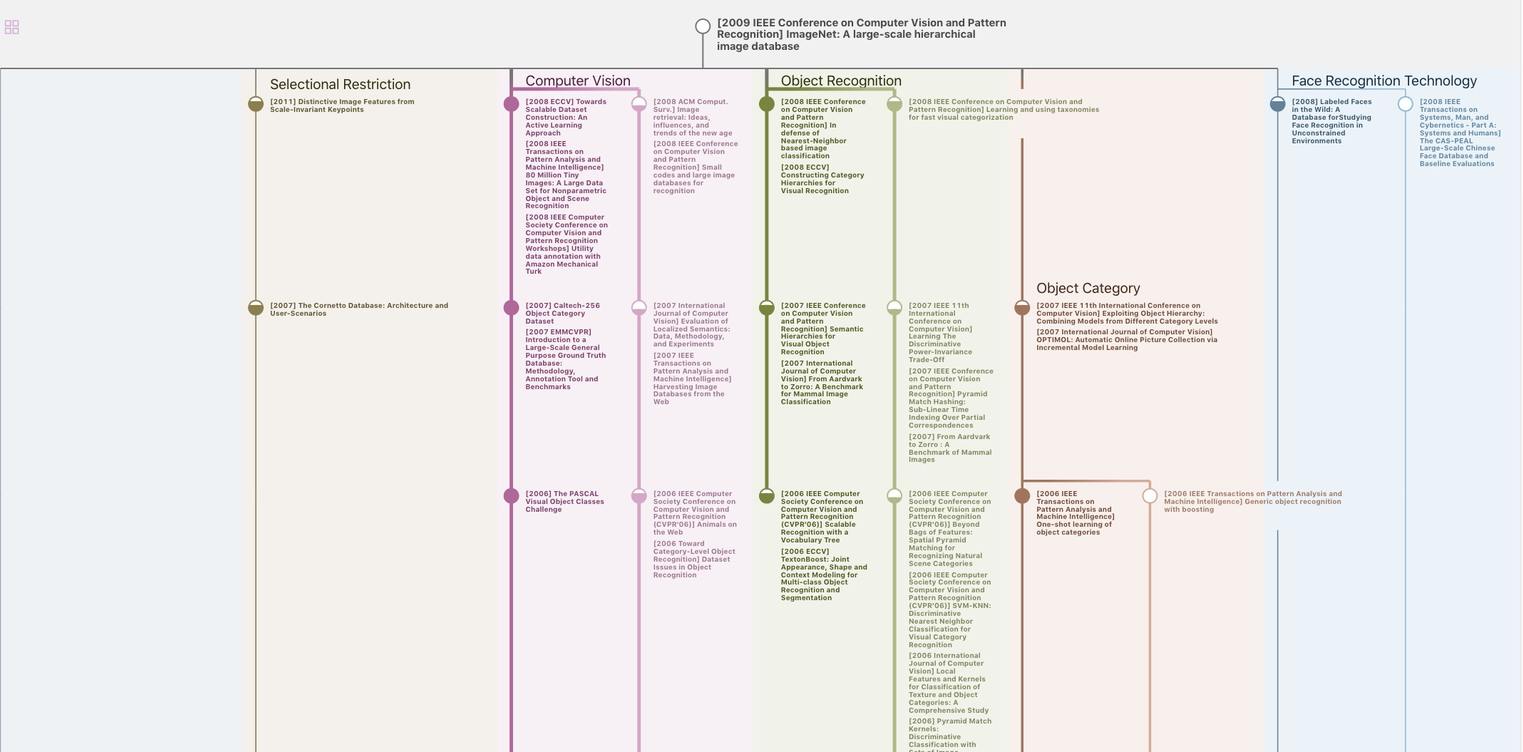
生成溯源树,研究论文发展脉络
Chat Paper
正在生成论文摘要