GAN-Based Dual Active Learning for Nosocomial Infection Detection
IEEE Transactions on Network Science and Engineering(2022)
摘要
Although monitor devices and information systems are increasingly connected across hospitals, but scenarios such as hospital acquired infections (HAIs) detection where data are extremely scare and imbalanced has remained to be solved. In this paper, an architecture with generative adversarial networks (GANs) coupled with dual active learning (AL) modules was proposed to mitigate the burden of sampling and learning an inaccurate model caused by imbalanced HAIs datasets. Results showed that generating minority class by GANs could ease the problem of imbalanced data and dual AL modules could make the process of training GANs and sampling generated data more efficient and effective. Experiments on two real-world clinical HAIs datasets confirmed the effectiveness of the proposed framework. GAN balanced dataset improved accuray and f1-score by 7.75% and 12.67% compared to imbalanced dataset. The area of active selection accuracy curve above random selection accuracy curve accumulated to 1.13 and 0.52 from 0 to 50 iterations on two datasets. This approach can be applied in regional healthcare environment as a useful method for handling data scarcity and imbalance.
更多查看译文
关键词
Hospital acquired infection,imbalanced classification,over-sampling,active learning
AI 理解论文
溯源树
样例
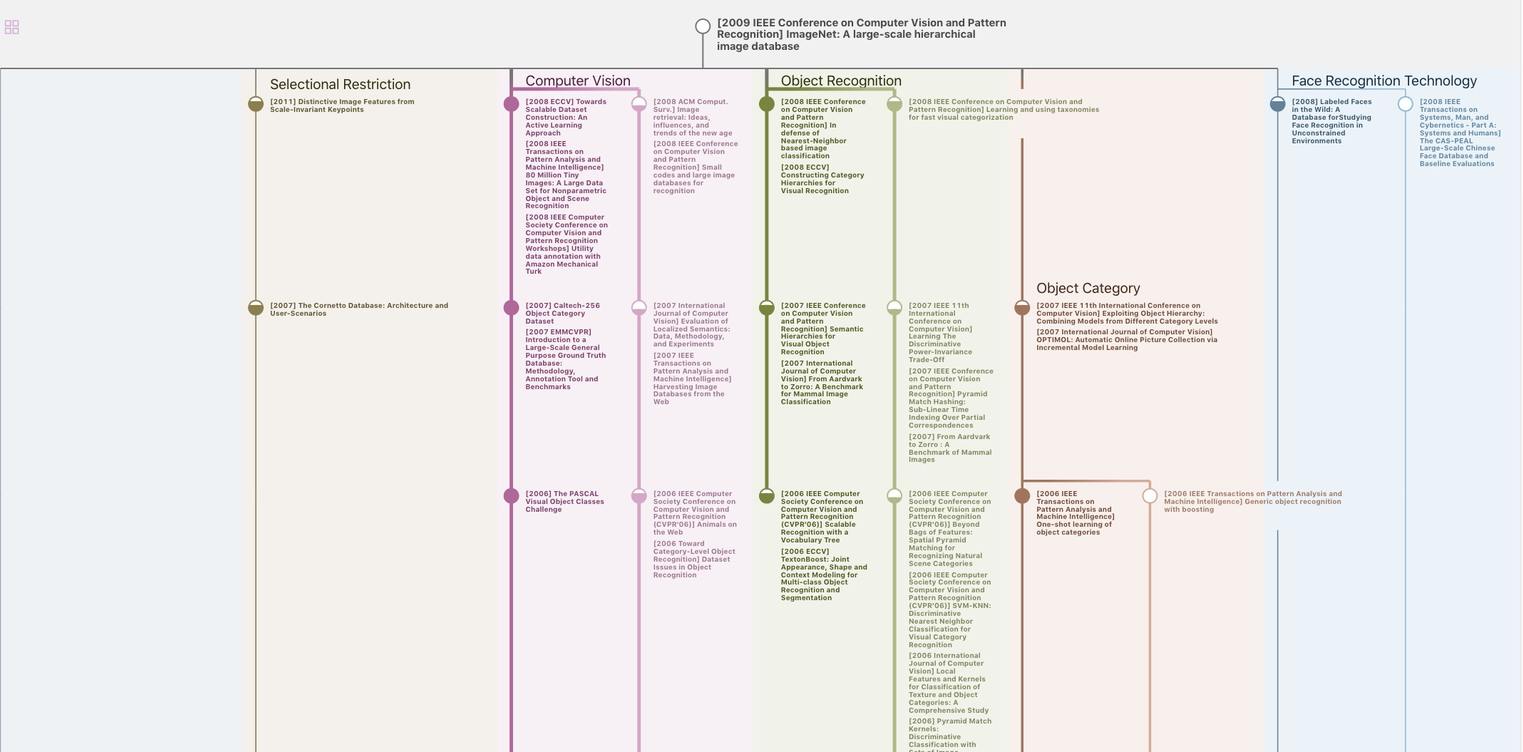
生成溯源树,研究论文发展脉络
Chat Paper
正在生成论文摘要