Social-Aware Caching Strategy Based on Joint Action Deep Reinforcement Learning
Wireless Communications and Mobile Computing(2021)
摘要
Caching in device-to-device (D2D) networks is emerging a promising trend, which enables to reduce backhaul traffic. Moreover, social interaction among users influences the performance of overall system network. Therefore, it is crucial to consider social attributes in the D2D networks to develop a caching strategy to resolve the problem of unbalanced content distributed. In this paper, we consider two types of users according to their activeness, i.e., active users and inactive users. Inactive users assist active users cache contents during off-peak periods and provide the contents to the active users during peak periods to relieve the pressure of base station (BS). In addition, caching system model is divided into physical domain model and social domain model. In physical domain, the quality of communication links is judged by the delay between D2D users. In social domain, based on a real-world dataset, CiaoDVD, we calculate user similarity in three dimensions and obtain user trust by a trust topology to measure user relationships. Finally, in order to maximize the cache hit ratio, a joint action deep Q -networks (JADQN) framework is proposed to pair the active users with inactive users and distribute the contents to inactive users. Simulation results indicate that the proposed strategy improves the cache hit ratio by 42.9% and reduces the download delay by 48.8% compared with least frequency used (LFU) algorithm, which validates the effectiveness of our method.
更多查看译文
AI 理解论文
溯源树
样例
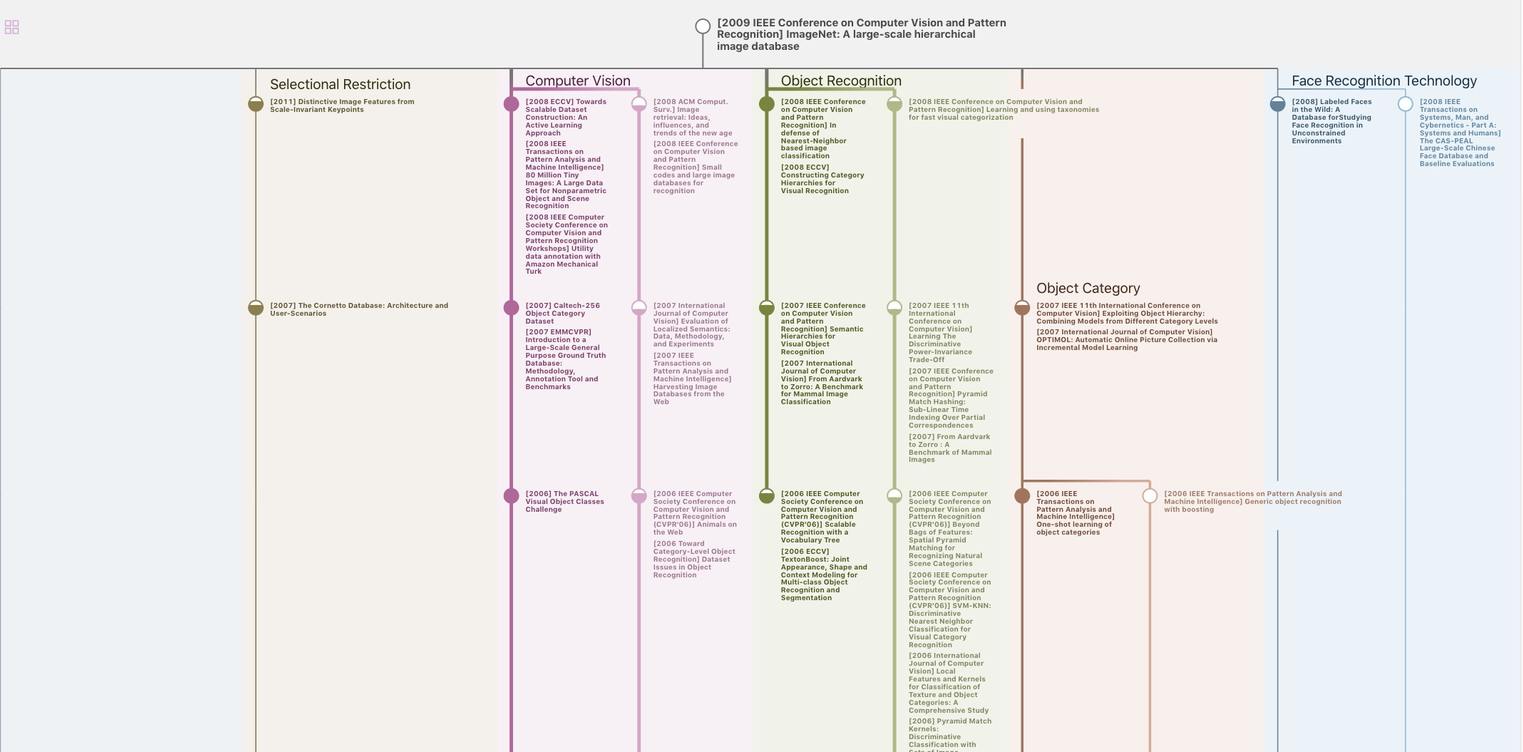
生成溯源树,研究论文发展脉络
Chat Paper
正在生成论文摘要