Incomplete multi-view clustering via independent self-representation learning
SCIENTIA SINICA Informationis(2021)
摘要
Incomplete multi-view clustering is a learning paradigm that combines heterogeneous and incomplete characteristics of multi-view data to obtain data structure and improve clustering performance. In practical application, each view will also be affected by missing values and outliers, in addition to missing some complete samples, which makes most traditional incomplete multi-view clustering methods ineffective. An incomplete multi-view clustering method based on independent self-presentation learning is proposed to solve the above issue. The proposed method fills in the missing features and learns the unique self-presentation matrix of each view using self-representation reconstruction. Then, to better mine the structure, low-rank constraints are added to the self-presentation matrix, and the diversity among different views is measured by introducing the Hilbert-Schmidt independence criterion. Experimental results on multiple data sets show that the proposed method can achieve better clustering than other advanced methods in most cases.
更多查看译文
关键词
learning,multi-view,self-representation
AI 理解论文
溯源树
样例
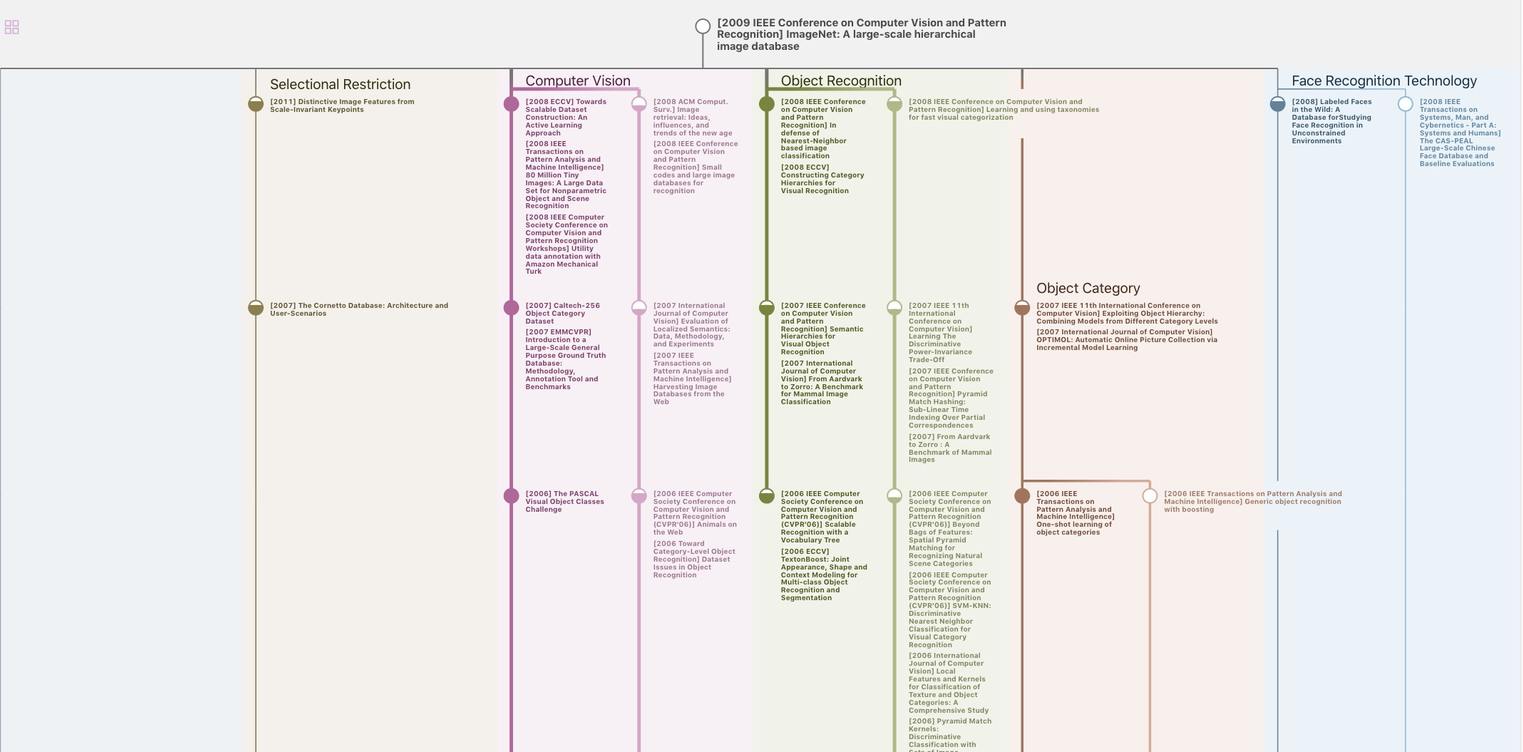
生成溯源树,研究论文发展脉络
Chat Paper
正在生成论文摘要