Joint Depth and Density Guided Single Image De-Raining
IEEE Transactions on Circuits and Systems for Video Technology(2022)
摘要
Single image de-raining is an important and highly challenging problem. To address this problem, some depth or density guided single-image de-raining methods have been developed with encouraging performance. However, these methods individually use the depth or the density to guide the network to conduct image de-raining. In this paper, a novel
joint depth and density guided de-raining
(JDDGD) method is technically developed. The JDDGD starts with a
depth-density inference network
(DDINet) to extract the depth and density information from an input rainy image, followed by a
depth-density-based
conditional generative adversarial network (DD-CGAN) to exploit the depth and density information provided by DDINet to achieve adaptive rain streak and fog removal. To prevent the spatially-varying local artifacts, an effective
global-local discriminators
structure is introduced in the proposed DD-CGAN to globally and locally inspect the generated images. In addition, multiple loss functions including
multi-scale pixel loss
,
multi-scale perceptual loss
, and
global-local generative adversarial loss
are also jointly used to train our model to achieve the best performance. Both quantitative and qualitative results show that the proposed JDDGD method achieves superior performance than previous
non-guided
,
density-guided
, and
depth-guided de-raining
methods.
更多查看译文
关键词
Single image de-raining,depth-density inference network,depth-density-based conditional generative adversarial network,global-local discriminators
AI 理解论文
溯源树
样例
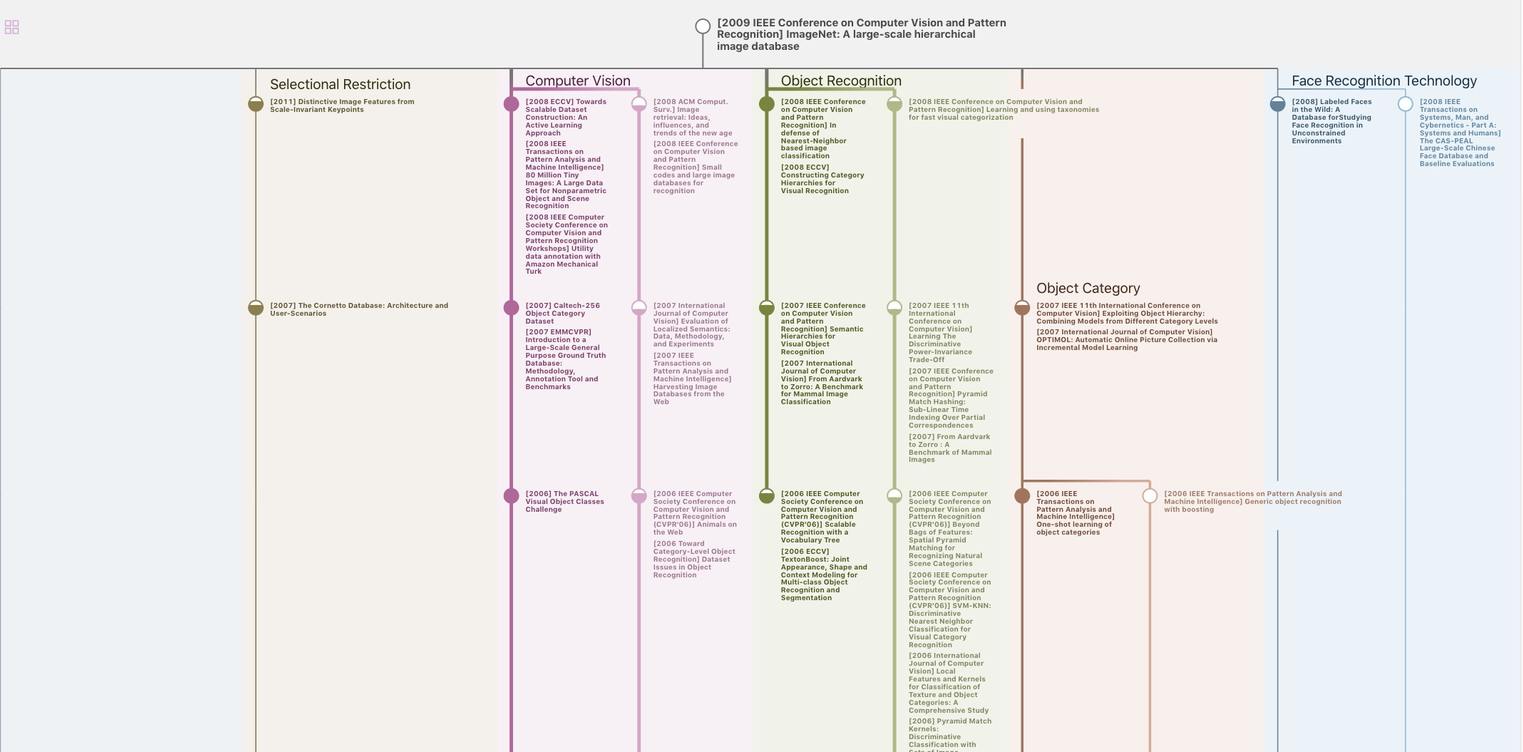
生成溯源树,研究论文发展脉络
Chat Paper
正在生成论文摘要