Continuous human learning optimization with enhanced exploitation and exploration
Intelligent Equipment, Robots, and VehiclesCommunications in Computer and Information Science(2023)
摘要
Human Learning Optimization (HLO) is an emergent inborn binary meta-heuristic inspired by human learning mechanisms. To solve continuous problems efficiently, continuous HLO (CHLO) variants were presented. However, the research on CHLO is at its very initial stage and we find that the underlying cooperation between the learning operators in previous CHLO variants is not ideal, which degrades the overall search ability. To tackle this drawback, this paper develops a new social learning operator (SLO) and presents a novel CHLO with Enhanced Exploration and Exploitation (CHLOEEE), in which the new SLO can efficiently explore a wider area and consequently strengthens the exploration ability. Thanks to the new SLO, the individual learning operator in CHLOEEE is totally relieved from the exploration duty and focuses on local search to further enhance the exploitation capability. By comparing and analyzing the search behaviors of CHLO variants, the advantages of CHLOEEE are clearly explained and the insight of the inherent mechanisms is revealed. Finally, the proposed CHLOEEE is evaluated on the CEC15, CEC17, and engineering benchmark problems and compared with the previous CHLO variants and other nine state-of-the-art meta-heuristic algorithms. The results show that CHLOEEE is superior to the latest CHLO variant, named CHLOEE, on two-thirds of all the benchmark functions and significantly outperforms the other algorithms on at least 12 out of 15 CEC15 functions and 21 out of 30 CEC17 functions. Furthermore, CHLOEEE achieves the best results on all the 9 engineering optimization problems.
更多查看译文
关键词
Continuous human learning optimization,Human learning optimization,Meta-heuristics,Social learning
AI 理解论文
溯源树
样例
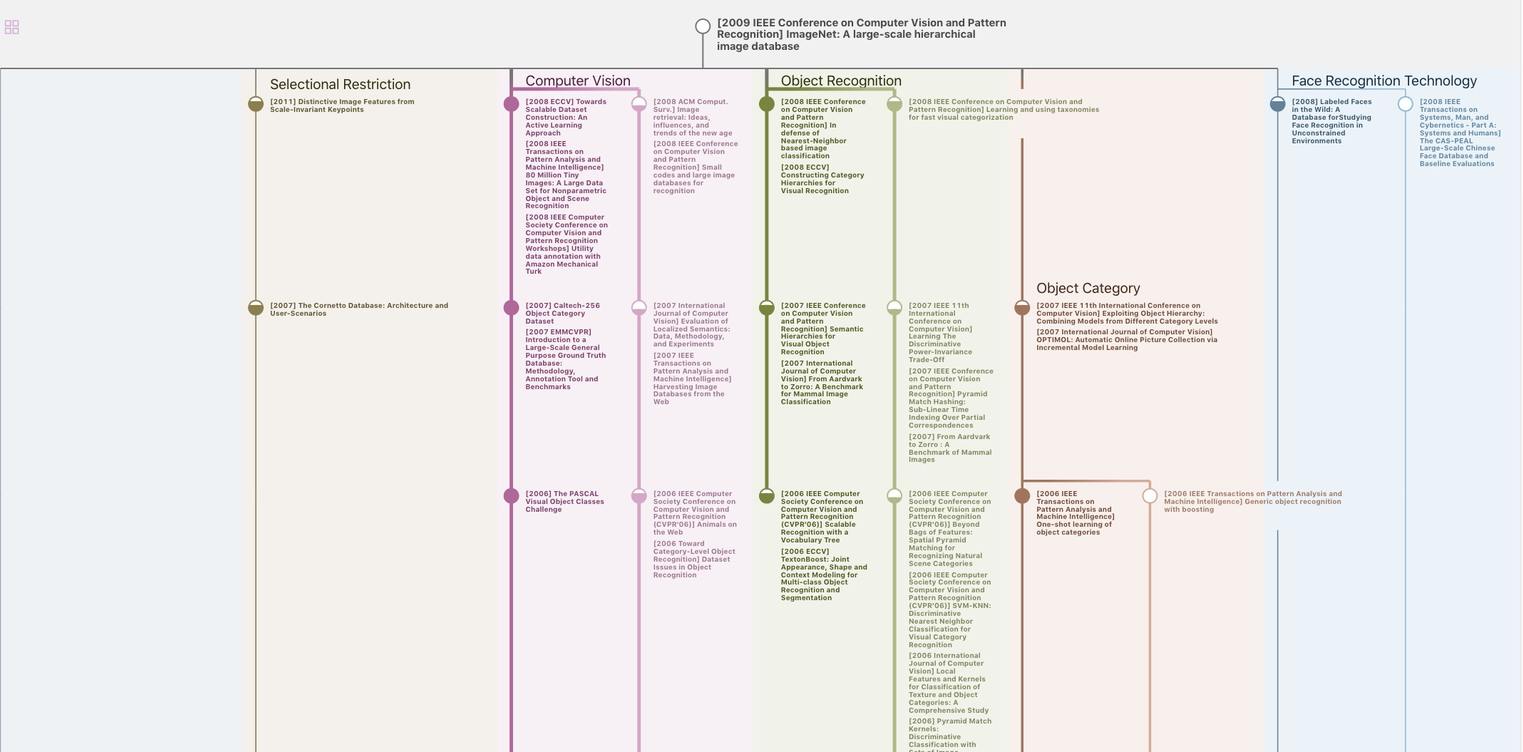
生成溯源树,研究论文发展脉络
Chat Paper
正在生成论文摘要