Neoclassical toroidal viscosity torque prediction via deep learning
Nuclear Fusion(2021)
摘要
Abstract GPECnet is a densely connected neural network that has been trained on GPEC data, to predict the plasma stability, neoclassical toroidal viscosity (NTV) torque, and optimized 3D coil current distributions for desired NTV torque profiles. Using NTV torque, driven by non-axisymmetric field perturbations in a tokamak, can be vital in optimizing pedestal performance by controlling the rotation profile in both the core, to ensure tearing stability, and the edge, to avoid edge localized modes (ELMs). The generalized perturbed equilibrium code (GPEC) software package can be used to calculate the plasma stability to 3D perturbations and the NTV torque profile generated by applied 3D magnetic fields. These calculations, however, involve complex integrations over space and energy distributions, which takes time to compute. Initially, GPECnet has been trained solely on data representative of the quiescent H-mode (QH) scenario, in which neutral beams are often balanced and toroidal rotation is low across the plasma profile. This work provides the foundation for active control of the rotation shear using a combination of beams and 3D fields for robust and high performance QH mode operation.
更多查看译文
关键词
deep learning, neoclassical toroidal viscosity torque, machine learning, NTV torque
AI 理解论文
溯源树
样例
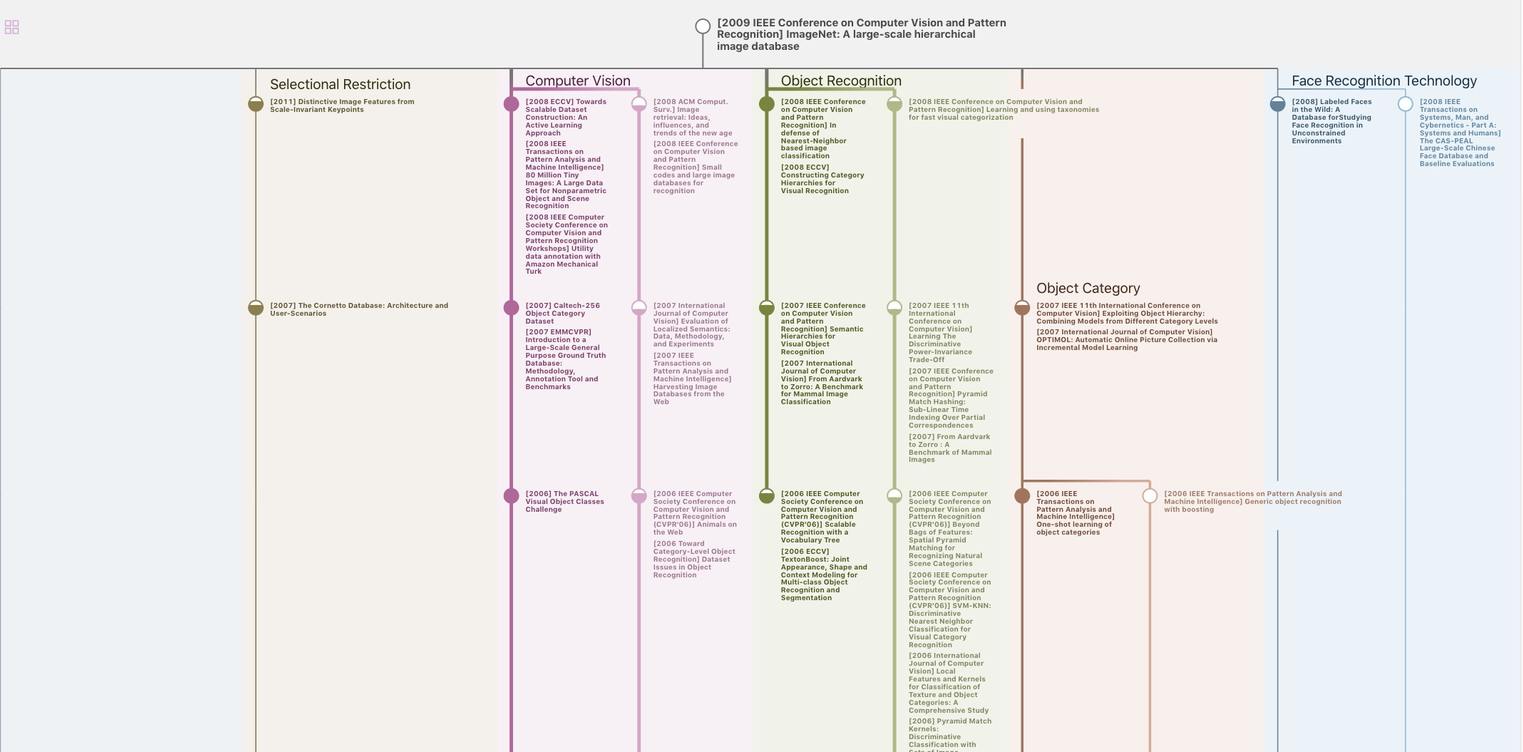
生成溯源树,研究论文发展脉络
Chat Paper
正在生成论文摘要