Explaining Neural Network Results by Sensitivity Analysis for Deception Detection
Communications in Computer and Information ScienceNeural Information Processing(2021)
摘要
Previous researches show that people are only able to recognise deception with an accuracy of 54%. In this paper, using physiological signals from observers, we train a three-layer neural network, a long short-term memory (LSTM) and a multi-tasking learning neural network (MTL-NN). We demonstrate that examined models are able to identify deception with an accuracy up to 62%, surpassing the average accuracy of human deception detection. The superior deception recognition ability shows that these tools are capable of helping people discriminate against deception. Further, to improve the interpret-ability of neural networks, we extract rules from the trained models using sensitivity analysis. We find that the rule extraction methods using sensitivity analysis along with genetic algorithm (GA) based data reduction successfully explain all three neural network models. We hope the rule extraction methods can help to improve the interpret-ability of neural networks.
更多查看译文
关键词
neural network results,neural network,sensitivity analysis,detection
AI 理解论文
溯源树
样例
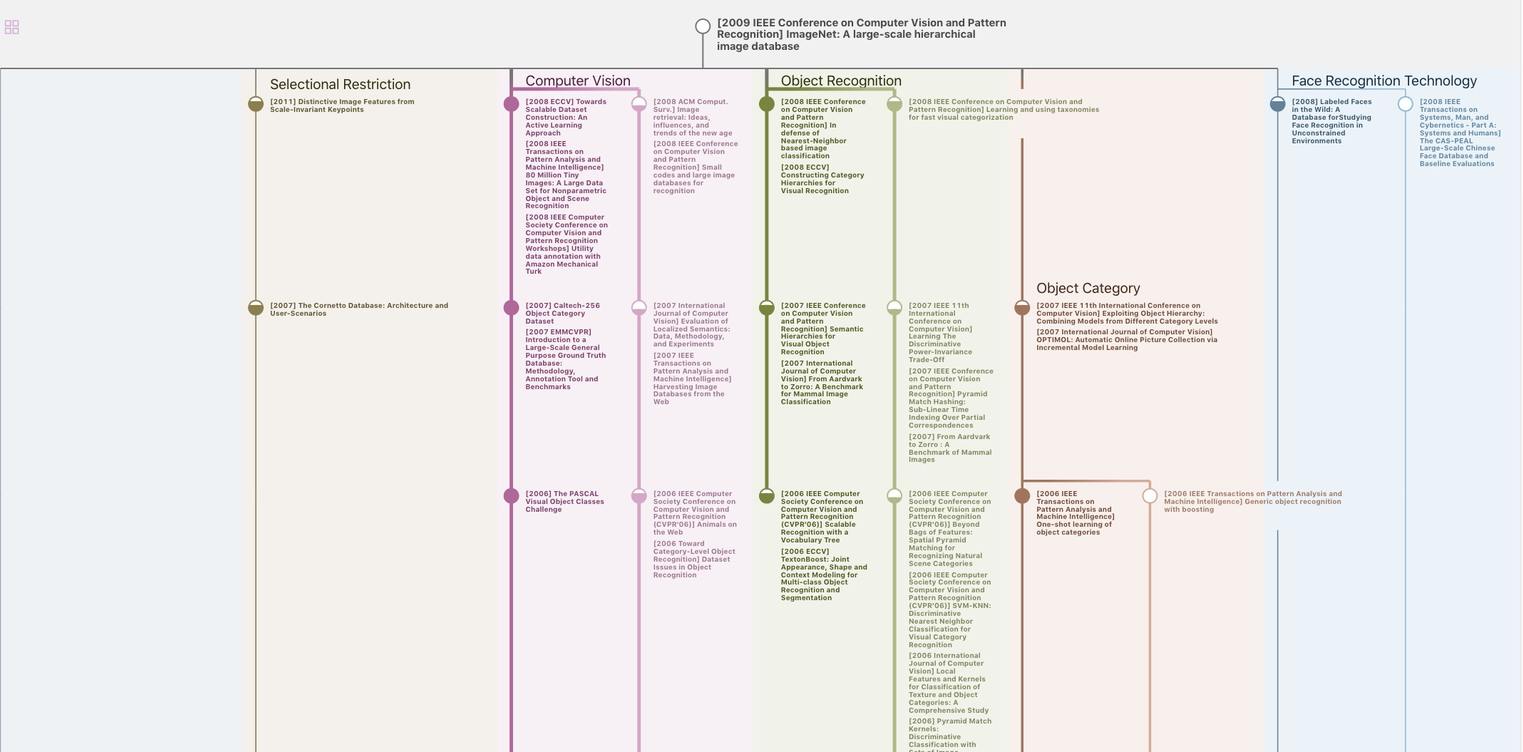
生成溯源树,研究论文发展脉络
Chat Paper
正在生成论文摘要