1255. External Validation and Systematic Quantification of the Predictive Performance of Carbapenem Resistant Enterobacterales Risk Prediction Models in Hospitalized Patients
Open Forum Infectious Diseases(2021)
摘要
Abstract Background Accurately predicting the presence of a carbapenem resistant enterobacterales (CRE) in hospitalized patients presents itself as an opportunity that would support timely initiation of CRE active agents. The aim of this study is to determine how reliably the existing risk prediction models identify patients likely to require empiric anti-CRE treatment, preliminary results of which are presented herein. Methods A systematic search identified all existing CRE prediction models for validation in our patient population. Medical records of hospitalized patients within the Mount Sinai Health System in New York were subsequently reviewed. Data was gathered on model predictors, baseline demographics, clinical information, microbiology results, antibiotic utilization history and index infection. Besides calculating the AUROC, the main outcome of our study was to establish optimal prediction score cutoffs and false positive rates (FPR) where corresponding model performance maintains a false negative rate (FNR) of < 10%, < 20% and < 30%, respectively. Results 12 models were retained for validation. We identified 106 patients, 41 of which were treated for a CRE infection. Previous admission, organ transplantation, CKD, infection type, and carbapenem use were baseline variables that significantly differed between the groups treated for a CRE or non-CRE related infection (Table 1). The models ability to discriminate varied as evidenced by the AUROC range of 0.5 to 0.77 (Figure 1), suggesting the Seligmen et al. model as the overall best. When evaluated at the pre-specified FNR intervals of < 10%, < 20% and < 30%, the model by Lodise et al., Seligman et al., and Vazquez-Guillamet et al. produced the best FPR, respectively (Table 2). Table 1. Baseline characchteristics Table 2. Model Performance Figure 1. AUROCs Conclusion Discriminative ability of the risk prediction models showed varying performance. The model by Lodise et al. appears to be most useful when a low risk level is deemed acceptable for failure rate, while at a moderate to high risk of missing a CRE case (20% and 30% FNR), the methods by Seligman and Vazquez-Guillamet et al. are most desirable as they minimize the chance of over-treatment. Additional work to increase sample size and to evaluate the models inter-rater reliability is currently on going. Disclosures All Authors: No reported disclosures
更多查看译文
AI 理解论文
溯源树
样例
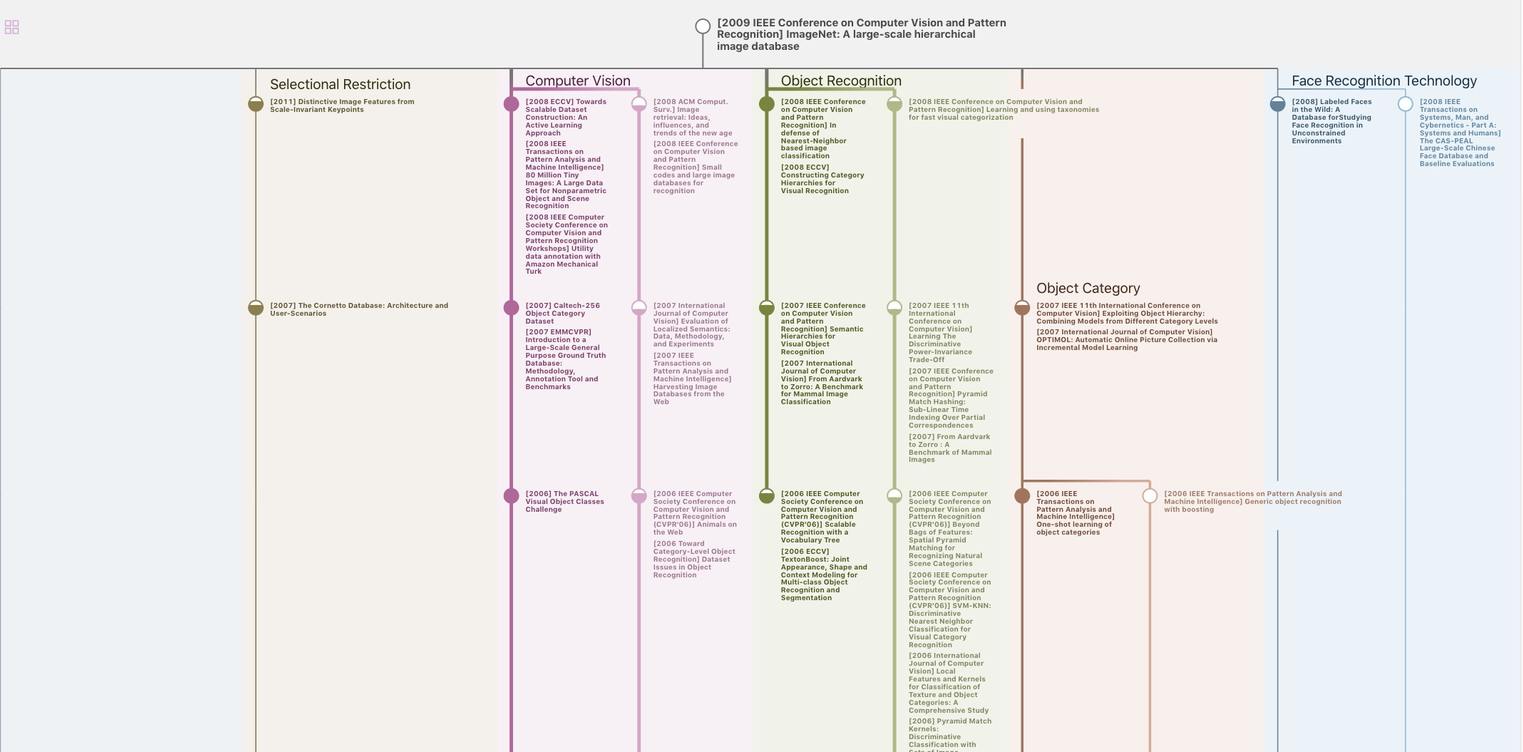
生成溯源树,研究论文发展脉络
Chat Paper
正在生成论文摘要