Hybrid hard-soft screening for high dimensional latent class analysis
STATISTICA SINICA(2023)
摘要
Latent class analysis (LCA) is a powerful tool for detecting unobservable subgroups within a population. When a large number of covariates (features) are considered, an LCA faces great challenges in terms of both classification accuracy and computational efficiency. In this paper, we propose a novel feature screening procedure that eliminates most irrelevant features before an LCA is conducted. The proposed method is built on an EM-based hybrid hard-soft thresholding update (HHS-EM) of the latent class parameters, which naturally accounts for the joint effects between features. We show that the HHS-EM enjoys the sure screening property and leads to a refined LCA that is effective and consistent for high-dimensional classification. The performance of the proposed method is illustrated by means of simulation studies and a real-data example.
更多查看译文
关键词
Feature screening,high-dimensional classification,latent class analysis,misclassification error,sure joint screening
AI 理解论文
溯源树
样例
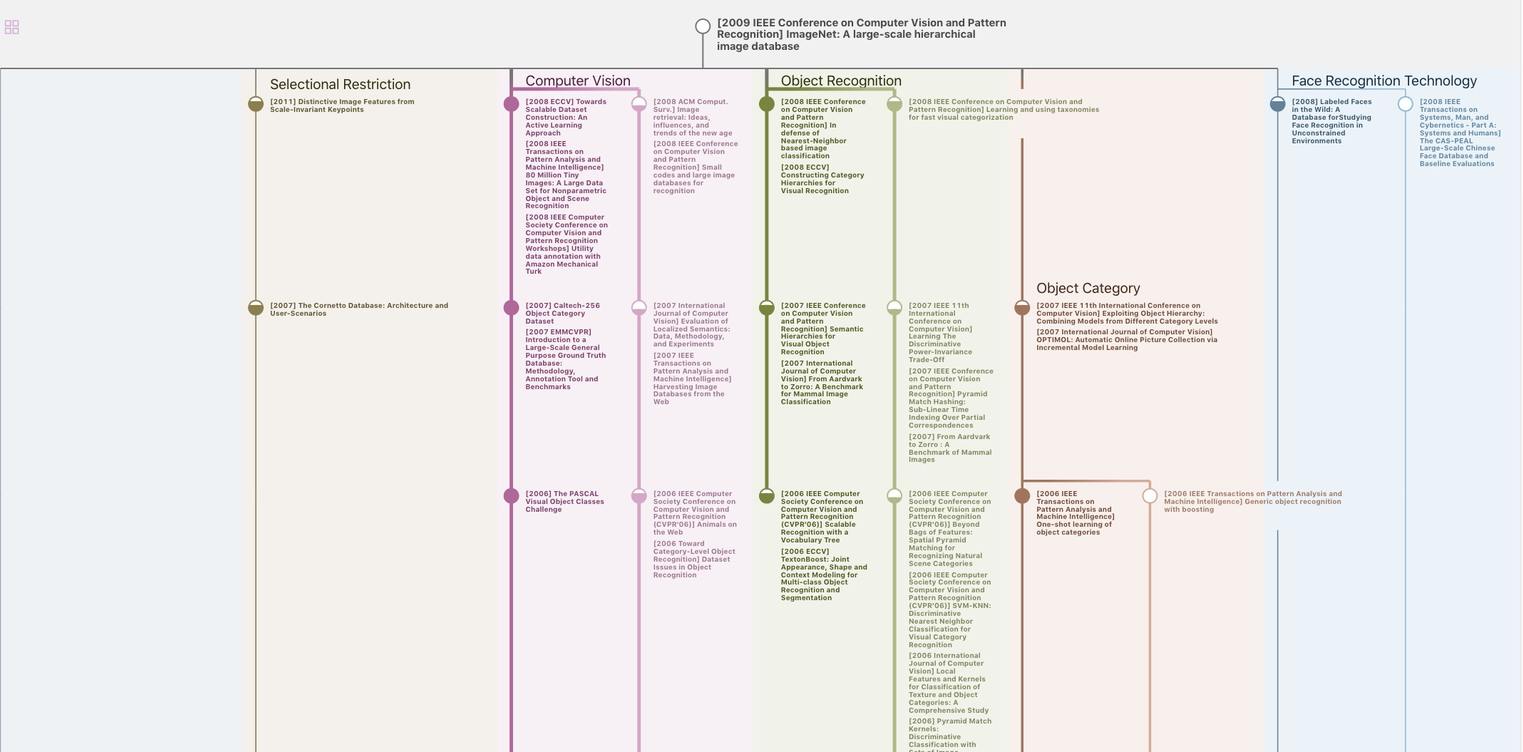
生成溯源树,研究论文发展脉络
Chat Paper
正在生成论文摘要