On the proper choice of datasets and traffic features for real-time anomaly detection
Journal of Physics: Conference Series(2021)
摘要
Abstract Thanks to its ability to face unknown attacks, Anomaly-based Intrusion Detection is a key research topic in network security and different statistical methods, fed by suitable traffic features, have been proposed in the literature. The choice of a proper dataset is a critical element not only for performance comparison, but also for the correct identification of the normal traffic behaviour. In this paper we address the general problem of selecting traffic features from recent real traffic traces (MAWI data set) and verify how the real-time constraint impacts on the general performance. Although a state-of-the-art IDS (Intrusion Detection System) based on deep neural networks is considered, our conclusions can be extended to any anomaly detection algorithm and advocate for a fair comparison of IDSs using representative datasets and traffic features that can be extracted on-line (and do not depend on the entire dataset).
更多查看译文
关键词
traffic features,datasets,detection,real-time
AI 理解论文
溯源树
样例
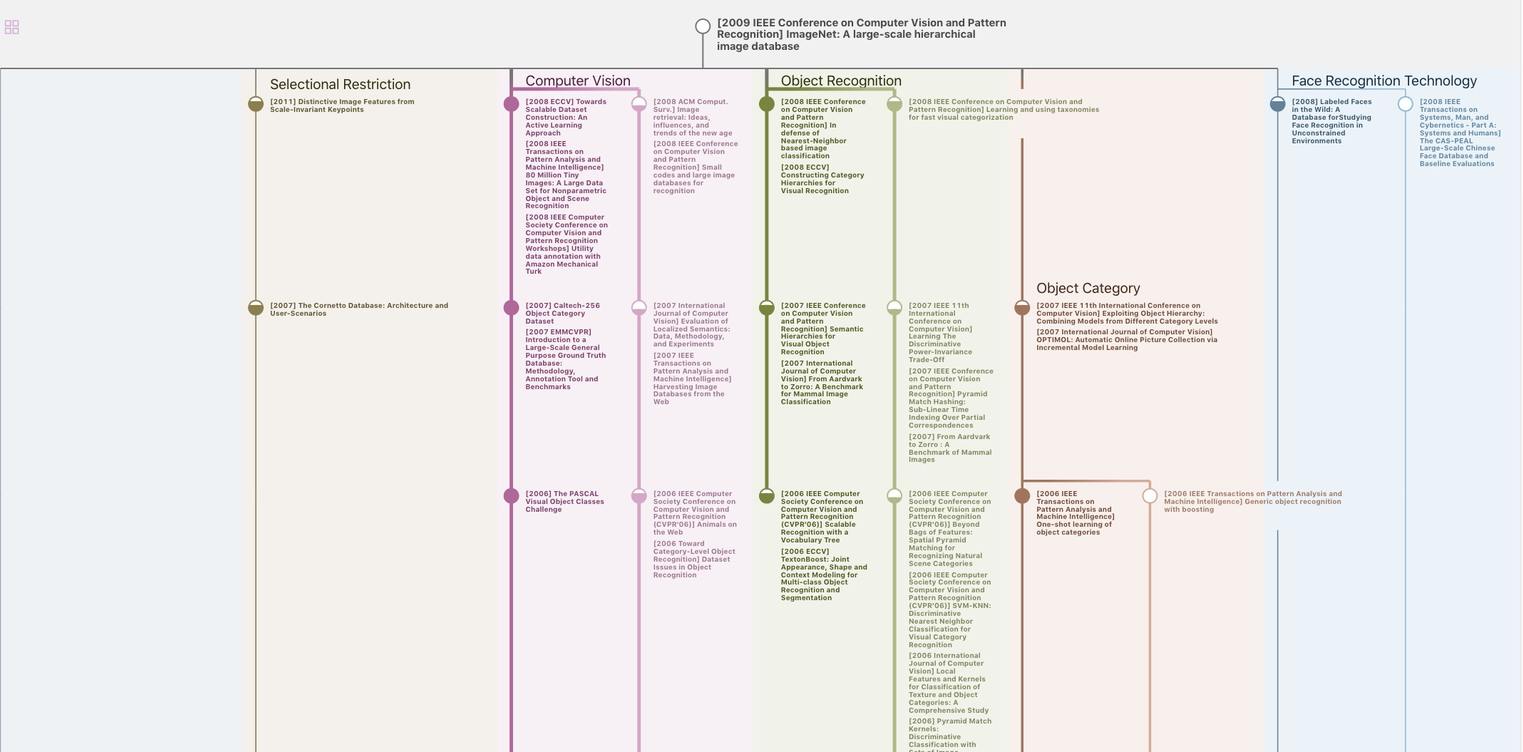
生成溯源树,研究论文发展脉络
Chat Paper
正在生成论文摘要