A hierarchical intrusion detection system based on extreme learning machine and nature-inspired optimization
SSRN Electronic Journal(2023)
摘要
The surge in cyber-attacks has driven demand for robust Intrusion detection systems (IDSs) to protect underlying data and sustain availability of network services. Detecting and classifying multiple type of attacks requires robust machine learning approaches that can analyze network traffic and take appropri-ate measures. Traffic data usually consists of redundant, irrelevant, and noisy information, which could have a negative influence on the model performance. In this paper, we propose an improved bio-inspired meta-heuristic algorithm for efficient detection and classification of multi-stage attacks. The proposed model uses a one-versus-all sub-model based technique to deal with the multi-class classification prob-lem. Each sub-model employs an enhanced Harris Hawk optimization with extreme learning machine (ELM) as the base classifier. This hierarchy produces the best subset of features per attack, along with optimized ELMs weights, which can improve the detection rate significantly. The proposed technique was tested against various meta-heuristic algorithms and multi-class classifiers using the UNSWNB-15 dataset. In seven different types of attacks, experimental results outperformed other existing methods in terms of decreasing the crossover-error rate and obtaining the best values for the G-mean measure.(c) 2022 Elsevier Ltd. All rights reserved.
更多查看译文
关键词
Intrusion detection system,Swarm intelligence,Extreme learning machine,Feature extraction,Crossover error rate
AI 理解论文
溯源树
样例
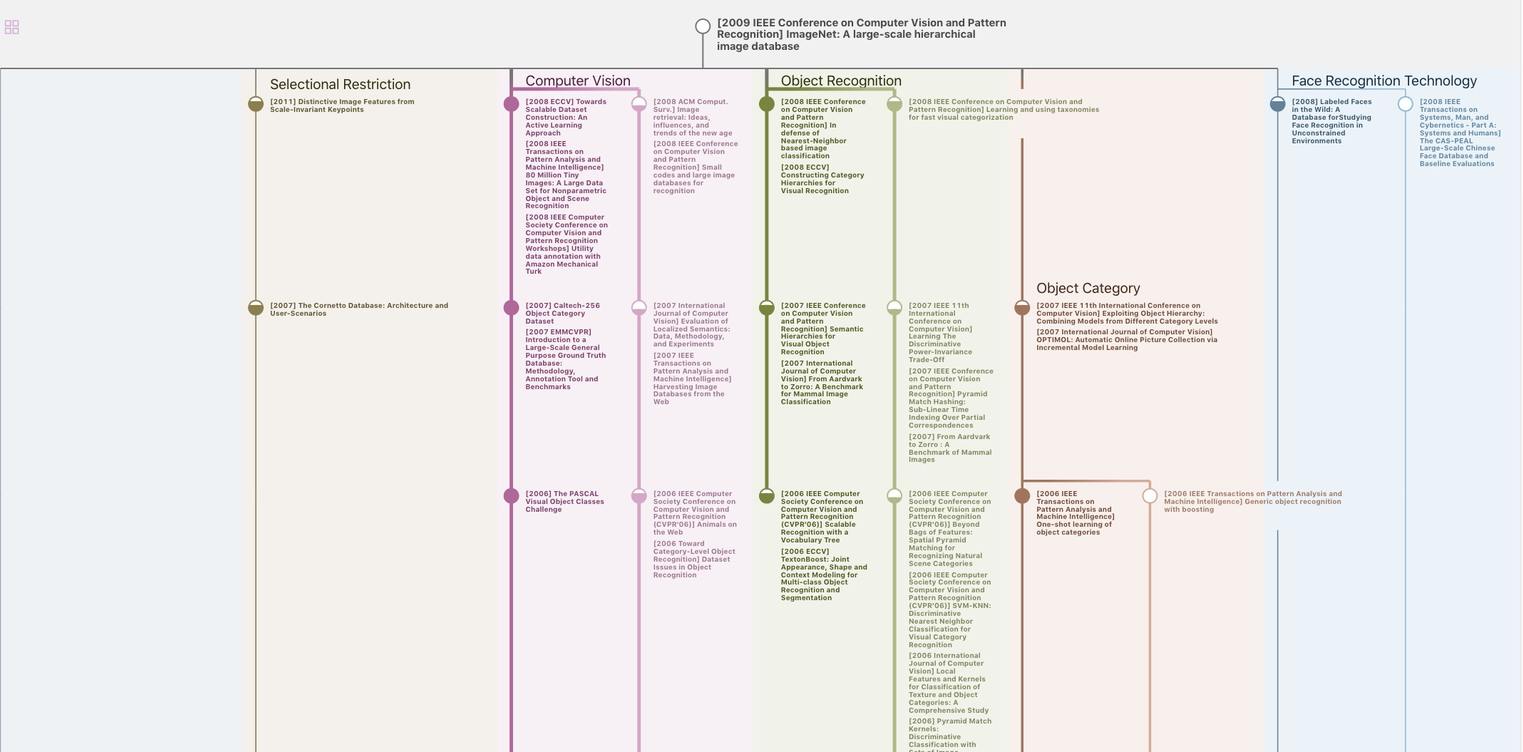
生成溯源树,研究论文发展脉络
Chat Paper
正在生成论文摘要