Anomaly Detection for Imbalanced Data via Deep Neural Network with Concentrated Loss
Frontiers in Artificial Intelligence and ApplicationsDesign Studies and Intelligence Engineering(2022)
摘要
Deep neural networks have recently been used to address surface anomaly detection in industrial quality control and have achieved much success. However, addressing the data imbalance problem, especially the Easy/Hard Examples (EHE) imbalance problem, remain a challenging task in anomaly detection. To alleviate this problem, we propose a two-stage convolutional neural network with a novel loss function, i.e., concentrated loss function. Specifically, the concentrated loss function enables the model to pay more attention to hard examples and improve the quality of segmentation for imbalanced data. To verify the effect of our method, we implement our method on the surface anomaly detection dataset, i.e., the KolektorSDD2 dataset. The experimental results show the superiority of our method over the other state-of-the-art approaches.
更多查看译文
关键词
imbalanced data,deep neural network,loss
AI 理解论文
溯源树
样例
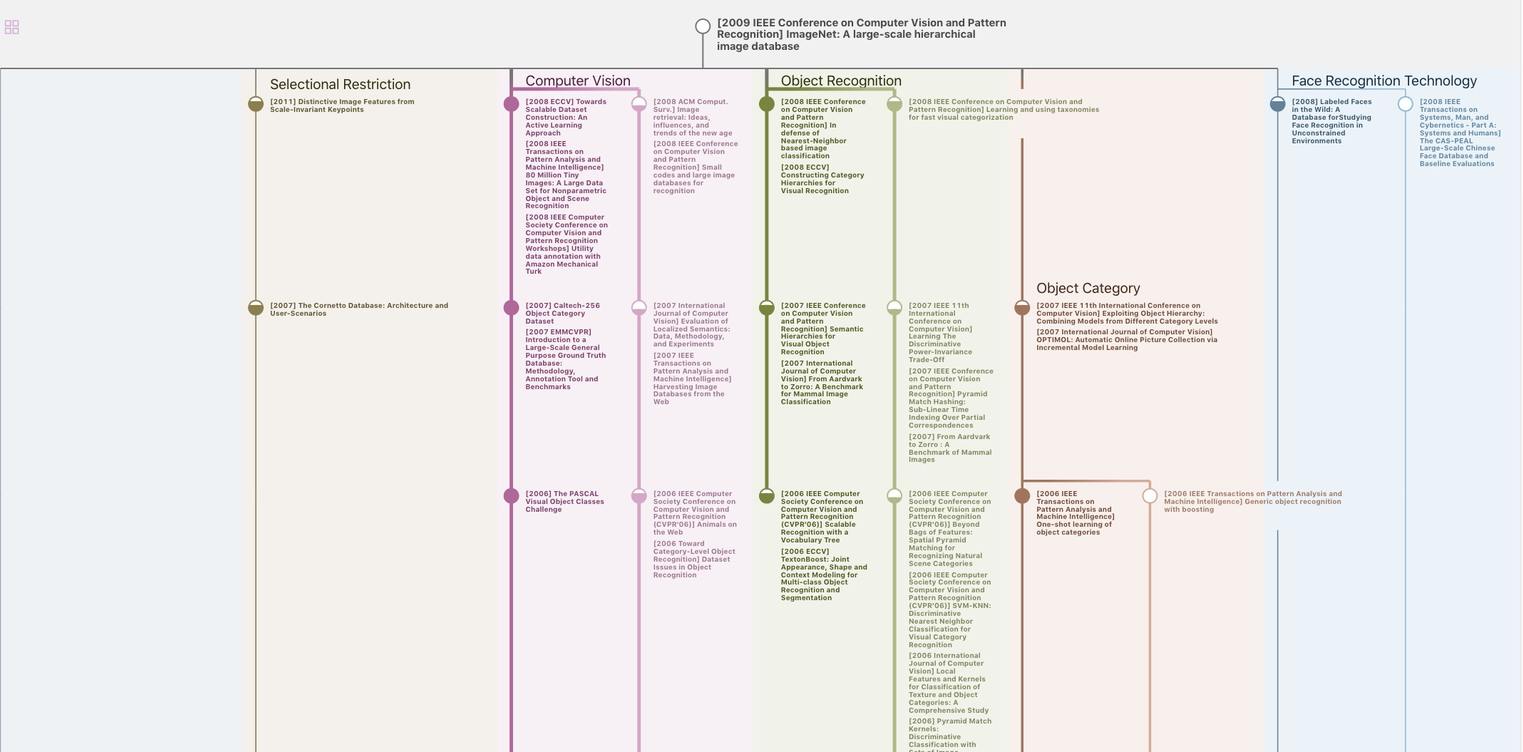
生成溯源树,研究论文发展脉络
Chat Paper
正在生成论文摘要