Using stochastic hierarchical aggregation constraints to nowcast regional economic aggregates
INTERNATIONAL JOURNAL OF FORECASTING(2024)
摘要
Recent decades have seen advances in using econometric methods to produce more timely and higher frequency estimates of economic activity at the national level, enabling better tracking of the economy in real-time. These advances have not generally been replicated at the sub -national level, likely because of the empirical challenges that nowcasting at a regional level presents, notably, the short time series of available data, changes in data frequency over time, and the hierarchical structure of the data. This paper develops a mixed -frequency Bayesian VAR model to address common features of the regional nowcasting context, using an application to regional productivity in the UK. We evaluate the contribution that different features of our model provide to the accuracy of point and density nowcasts, in particular, the role of hierarchical aggregation constraints. We show that these aggregation constraints, imposed in stochastic form, play a crucial role in delivering improved regional nowcasts; they prove more important than adding region -specific predictors when the equivalent national data are known, but not when this aggregate is unknown. (c) 2022 The Author(s). Published by Elsevier B.V. on behalf of International Institute of Forecasters. This is an open access article under the CC BY license (http://creativecommons.org/licenses/by/4.0/).
更多查看译文
关键词
Regional data,Mixed frequency,Nowcasting,Bayesian methods,Real-time data,Vector autoregressions
AI 理解论文
溯源树
样例
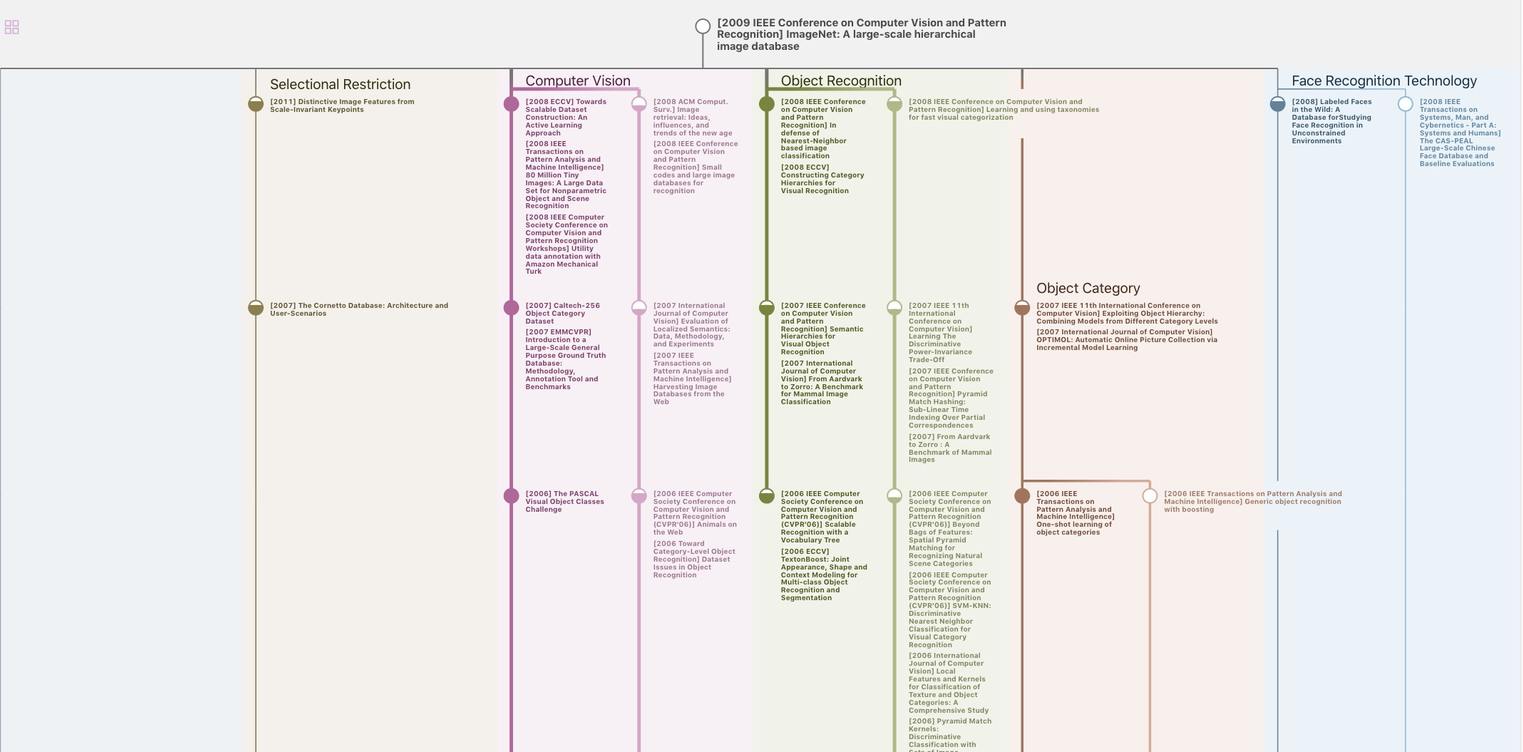
生成溯源树,研究论文发展脉络
Chat Paper
正在生成论文摘要